Comprehensive Analysis of Network Robustness Evaluation Based on Convolutional Neural Networks with Spatial Pyramid Pooling
CoRR(2023)
摘要
Connectivity robustness, a crucial aspect for understanding, optimizing, and repairing complex networks, has traditionally been evaluated through time-consuming and often impractical simulations. Fortunately, machine learning provides a new avenue for addressing this challenge. However, several key issues remain unresolved, including the performance in more general edge removal scenarios, capturing robustness through attack curves instead of directly training for robustness, scalability of predictive tasks, and transferability of predictive capabilities. In this paper, we address these challenges by designing a convolutional neural networks (CNN) model with spatial pyramid pooling networks (SPP-net), adapting existing evaluation metrics, redesigning the attack modes, introducing appropriate filtering rules, and incorporating the value of robustness as training data. The results demonstrate the thoroughness of the proposed CNN framework in addressing the challenges of high computational time across various network types, failure component types and failure scenarios. However, the performance of the proposed CNN model varies: for evaluation tasks that are consistent with the trained network type, the proposed CNN model consistently achieves accurate evaluations of both attack curves and robustness values across all removal scenarios. When the predicted network type differs from the trained network, the CNN model still demonstrates favorable performance in the scenario of random node failure, showcasing its scalability and performance transferability. Nevertheless, the performance falls short of expectations in other removal scenarios. This observed scenario-sensitivity in the evaluation of network features has been overlooked in previous studies and necessitates further attention and optimization. Lastly, we discuss important unresolved questions and further investigation.
更多查看译文
关键词
network robustness evaluation,convolutional neural networks,pyramid
AI 理解论文
溯源树
样例
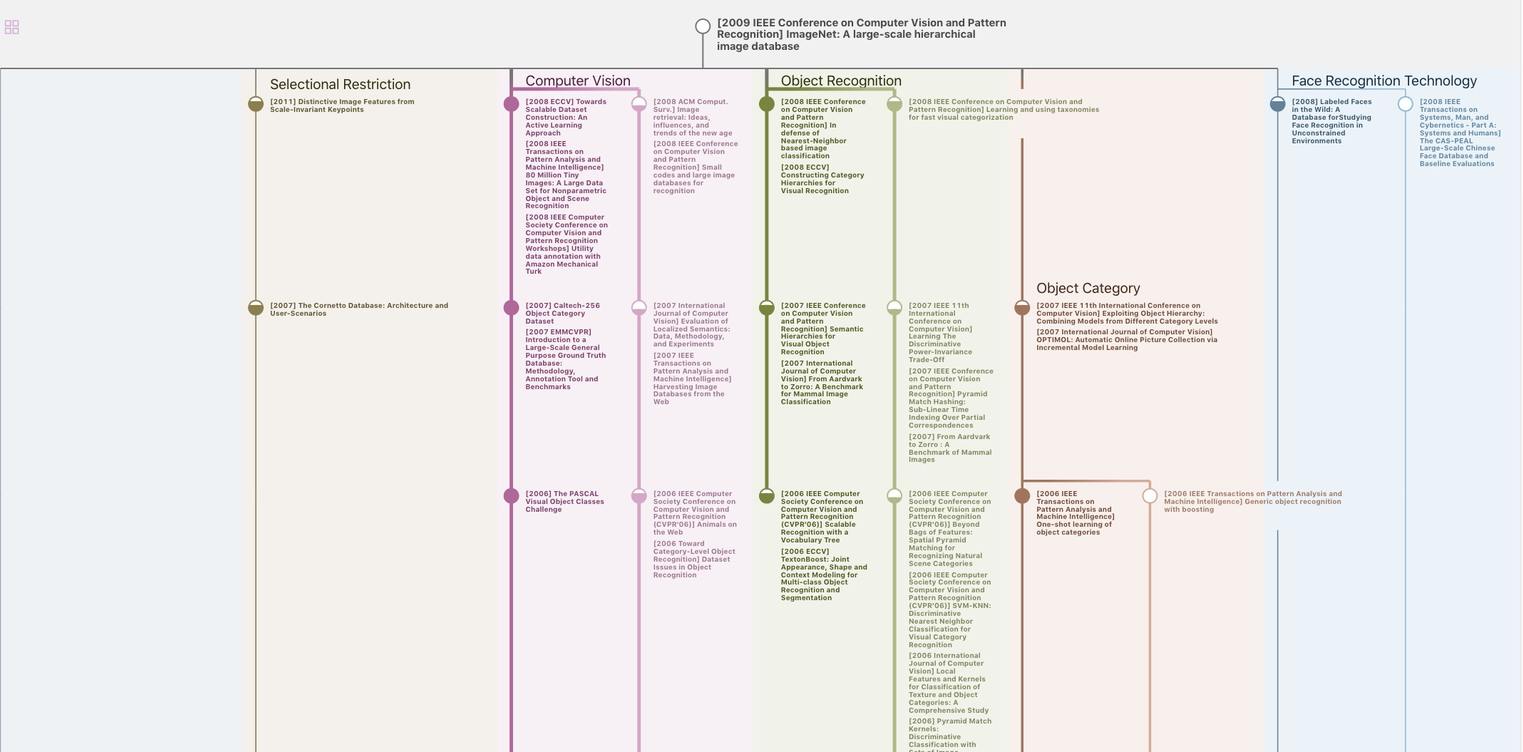
生成溯源树,研究论文发展脉络
Chat Paper
正在生成论文摘要