Low-Light Image Enhancement with Illumination-Aware Gamma Correction and Complete Image Modelling Network
2023 IEEE/CVF International Conference on Computer Vision (ICCV)(2023)
摘要
This paper presents a novel network structure with illumination-aware gamma correction and complete image modelling to solve the low-light image enhancement problem. Low-light environments usually lead to less informative large-scale dark areas, directly learning deep representations from low-light images is insensitive to recovering normal illumination. We propose to integrate the effectiveness of gamma correction with the strong modelling capacities of deep networks, which enables the correction factor gamma to be learned in a coarse to elaborate manner via adaptively perceiving the deviated illumination. Because exponential operation introduces high computational complexity, we propose to use Taylor Series to approximate gamma correction, accelerating the training and inference speed. Dark areas usually occupy large scales in low-light images, common local modelling structures, e.g., CNN, SwinIR, are thus insufficient to recover accurate illumination across whole low-light images. We propose a novel Transformer block to completely simulate the dependencies of all pixels across images via a local-to-global hierarchical attention mechanism, so that dark areas could be inferred by borrowing the information from far informative regions in a highly effective manner. Extensive experiments on several benchmark datasets demonstrate that our approach outperforms state-of-the-art methods.
更多查看译文
关键词
complete image modelling network,gamma,low-light,illumination-aware
AI 理解论文
溯源树
样例
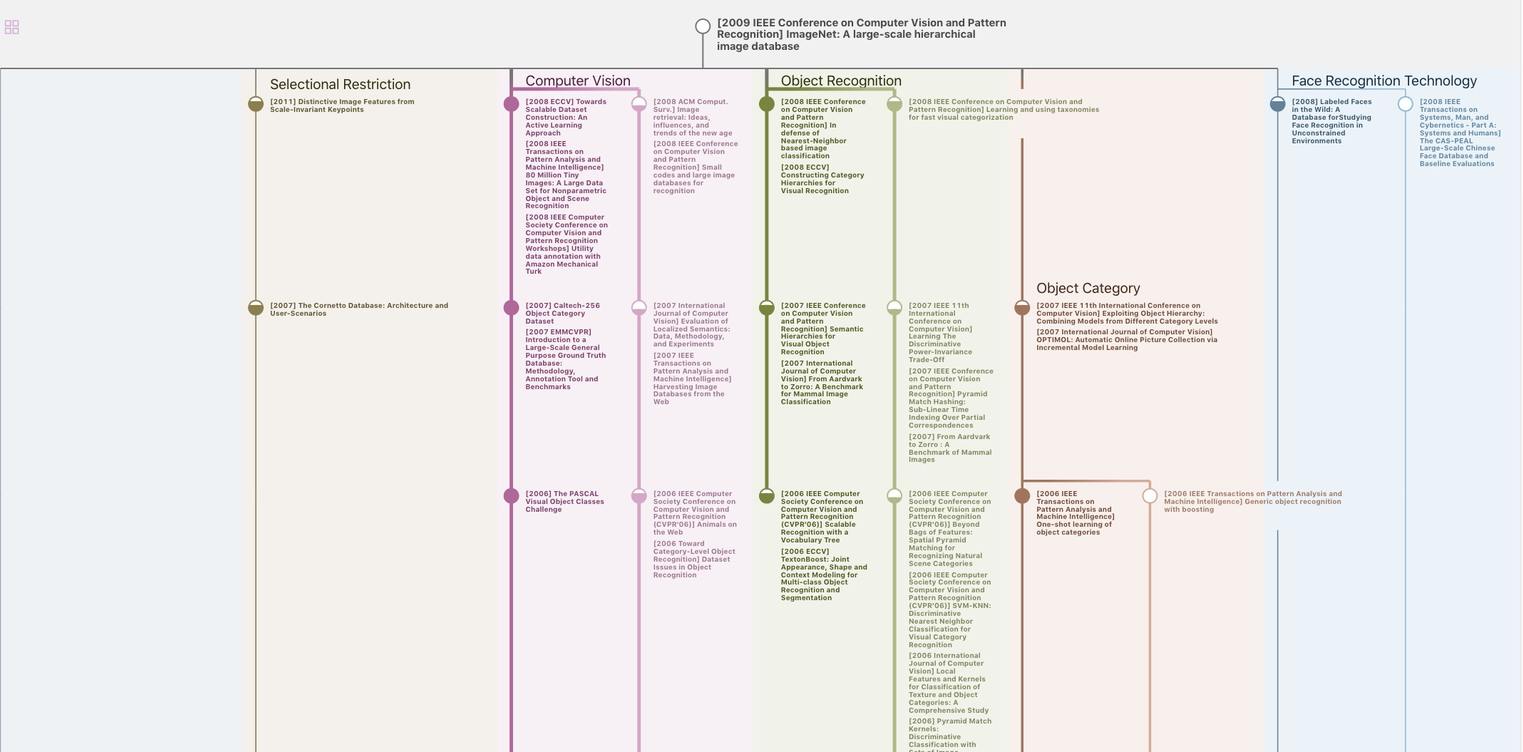
生成溯源树,研究论文发展脉络
Chat Paper
正在生成论文摘要