Digital Twinning of Cardiac Electrophysiology Models From the Surface ECG: A Geodesic Backpropagation Approach
IEEE TRANSACTIONS ON BIOMEDICAL ENGINEERING(2024)
摘要
The eikonal equation has become an indispensable tool for modeling cardiac electrical activation accurately and efficiently. In principle, by matching clinically recorded and eikonal-based electrocardiograms (ECGs), it is possible to build patient-specific models of cardiac electrophysiology in a purely non-invasive manner. Nonetheless, the fitting procedure remains a challenging task. The present study introduces a novel method, Geodesic-BP, to solve the inverse eikonal problem. Geodesic-BP is well-suited for GPU-accelerated machine learning frameworks, allowing us to optimize the parameters of the eikonal equation to reproduce a given ECG. We show that Geodesic-BP can reconstruct a simulated cardiac activation with high accuracy in a synthetic test case, even in the presence of modeling inaccuracies. Furthermore, we apply our algorithm to a publicly available dataset of a biventricular rabbit model, with promising results. Given the future shift towards personalized medicine, Geodesic-BP has the potential to help in future functionalizations of cardiac models meeting clinical time constraints while maintaining the physiological accuracy of state-of-the-art cardiac models.
更多查看译文
关键词
Mathematical models,Computational modeling,Electrocardiography,Optimization,Numerical models,Heart,Backpropagation,Cardiac digital twin,eikonal model,ECG,electrophysiology,backpropagation,machine learning
AI 理解论文
溯源树
样例
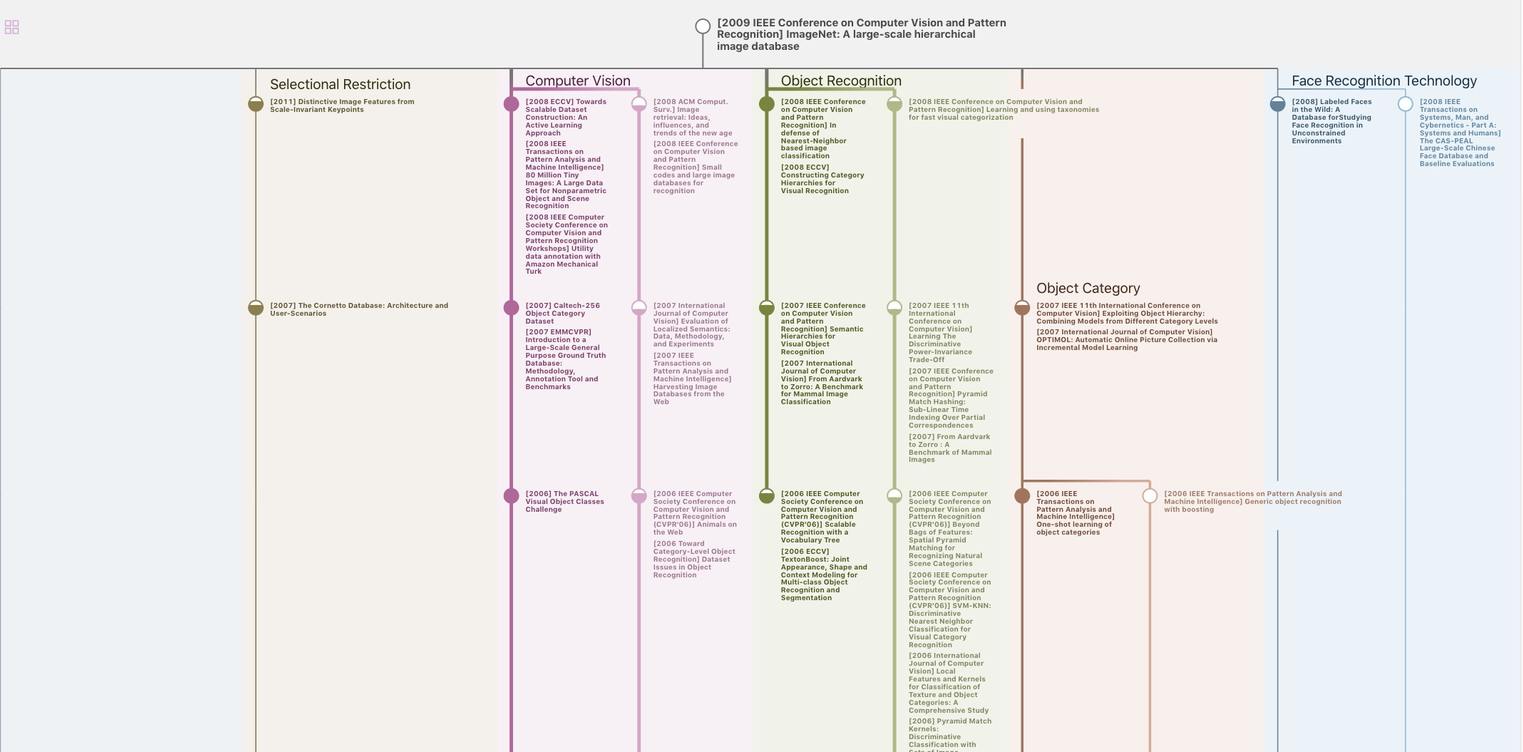
生成溯源树,研究论文发展脉络
Chat Paper
正在生成论文摘要