Robust Reward-Free Actor-Critic for Cooperative Multiagent Reinforcement Learning.
IEEE transactions on neural networks and learning systems(2023)
摘要
In this article, we consider centralized training and decentralized execution (CTDE) with diverse and private reward functions in cooperative multiagent reinforcement learning (MARL). The main challenge is that an unknown number of agents, whose identities are also unknown, can deliberately generate malicious messages and transmit them to the central controller. We term these malicious actions as Byzantine attacks. First, without Byzantine attacks, we propose a reward-free deep deterministic policy gradient (RF-DDPG) algorithm, in which gradients of agents' critics rather than rewards are sent to the central controller for preserving privacy. Second, to cope with Byzantine attacks, we develop a robust extension of RF-DDPG termed R2F-DDPG, which replaces the vulnerable average aggregation rule with robust ones. We propose a novel class of RL-specific Byzantine attacks that fail conventional robust aggregation rules, motivating the projection-boosted robust aggregation rules for R2F-DDPG. Numerical experiments show that RF-DDPG successfully trains agents to work cooperatively and that R2F-DDPG demonstrates robustness to Byzantine attacks.
更多查看译文
关键词
Byzantine attacks,centralized training and decentralized execution (CTDE),multiagent reinforcement learning (MARL)
AI 理解论文
溯源树
样例
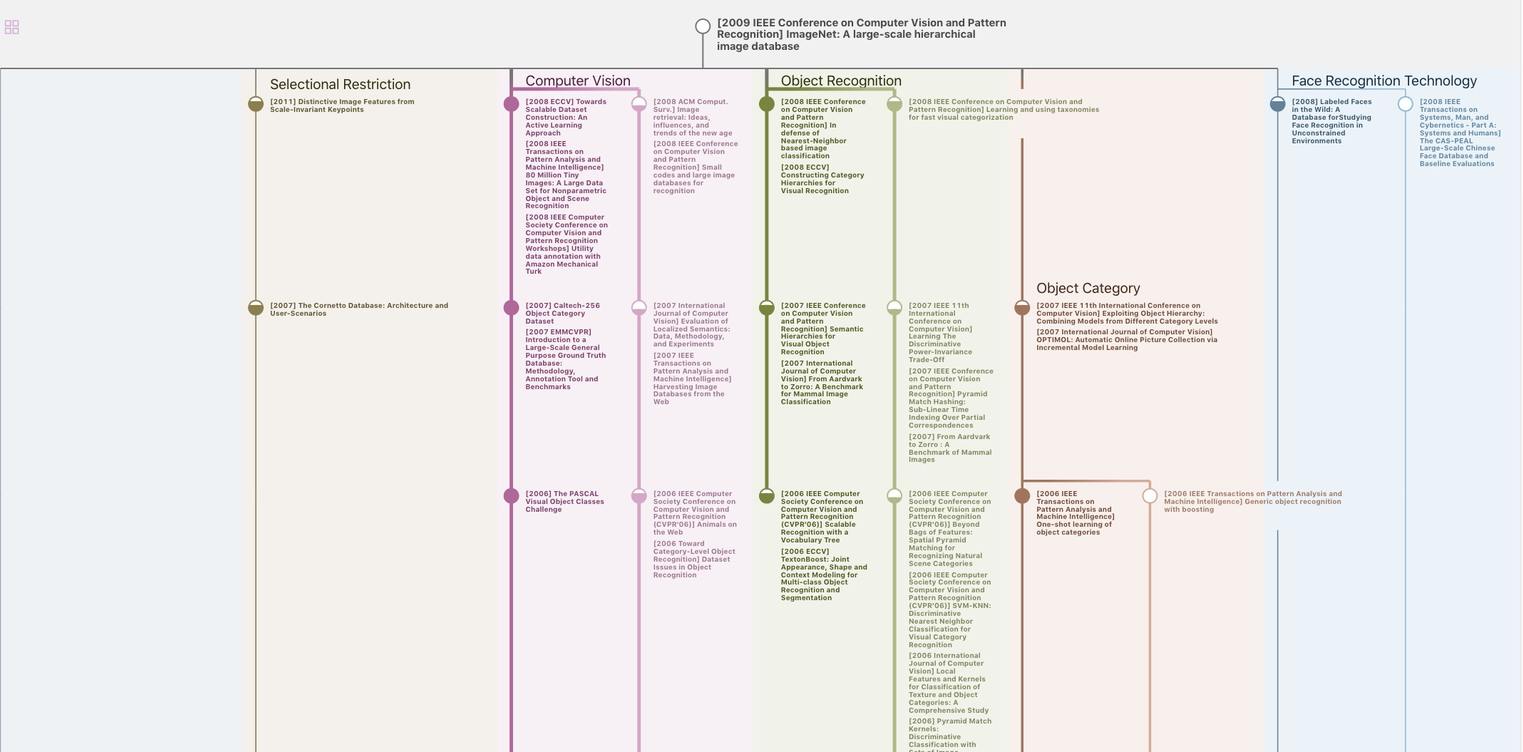
生成溯源树,研究论文发展脉络
Chat Paper
正在生成论文摘要