Adaptive Decomposition and Shared Weight Volumetric Transformer Blocks for Efficient Patch-free 3D Medical Image Segmentation.
IEEE journal of biomedical and health informatics(2023)
摘要
High resolution (HR) 3D medical image segmentation is vital for an accurate diagnosis. However, in the field of medical imaging, it is still a challenging task to achieve a high segmentation performance with cost-effective and feasible computation resources. Previous methods commonly use patch-sampling to reduce the input size, but this inevitably harms the global context and decreases the model's performance. In recent years, a few patch-free strategies have been presented to deal with this issue, but either they have limited performance due to their over-simplified model structures or they follow a complicated training process. In this study, to effectively address these issues, we present Adaptive Decomposition (A-Decomp) and Shared Weight Volumetric Transformer Blocks (SW-VTB). A-Decomp can adaptively decompose features and reduce their spatial size, which greatly lowers GPU memory consumption. SW-VTB is able to capture long-range dependencies at a low cost with its lightweight design and cross-scale weight-sharing mechanism. Our proposed cross-scale weight-sharing approach enhances the network's ability to capture scale-invariant core semantic information in addition to reducing parameter numbers. By combining these two designs together, we present a novel patch-free segmentation framework named VolumeFormer. Experimental results on two datasets show that VolumeFormer outperforms existing patch-based and patch-free methods with a comparatively fast inference speed and relatively compact design. Code is available at: https://github.com/Dootmaan/VolumeFormer.
更多查看译文
关键词
3D medical image segmentation,deep learning,patch-free,vision transformer
AI 理解论文
溯源树
样例
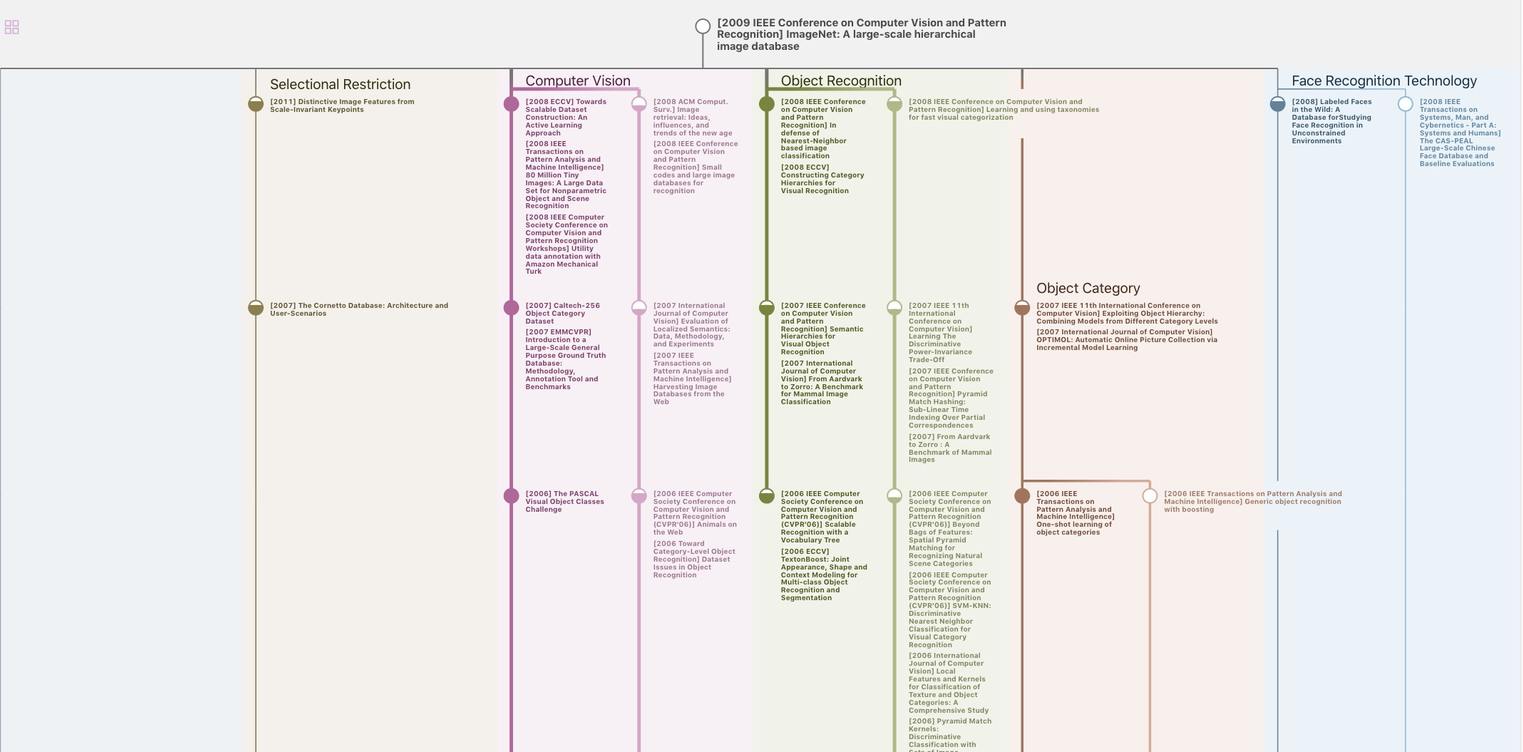
生成溯源树,研究论文发展脉络
Chat Paper
正在生成论文摘要