Skeletal Muscle Estimation Using Magnetic-Resonance-imaging-based Equations for Dual-Energy X-ray Absorptiometry and Bioelectrical Impedance Analysis.
European journal of clinical nutrition(2023)
摘要
Background/Objectives Skeletal muscle mass (SMM) estimation is important but challenging in clinical settings. Criterion methods, such as magnetic resonance imaging (MRI), are often inaccessible. However, surrogate methods, such as dual-energy X-ray absorptiometry (DXA) and multi-frequency bioelectrical impedance analysis (MFBIA), can use MRI-based equations to estimate SMM, although the agreement between these methods is unclear. Subjects/Methods Total and segmental SMM were estimated with DXA and MFBIA using MRI-based equations in 313 healthy adults (120 M, 193 F; age 30.2 ± 13.0 y; BMI 24.6 ± 4.0 kg/m 2 ). DXA total SMM was estimated using the Kim and McCarthy equations, and segmental SMM was estimated using the McCarthy equations. Relationships between DXA and MFBIA SMM were examined using Deming regression, Lin’s concordance correlation coefficient (CCC), equivalence testing, Bland-Altman analysis, and related tests. Results Strong linear relationships were observed for total (R 2 0.95, CCC 0.96–0.97), leg (R 2 0.90, CCC 0.85) and arm (R 2 0.93, CCC 0.93) SMM in the entire sample. Kim equation SMM demonstrated statistical equivalence with MFBIA for total SMM, but the Deming regression slope differed from 1 and proportional bias was present. McCarthy equation total SMM exhibited a regression slope that did not differ from 1, and no proportional bias was present in the entire sample. However, equivalence with MFBIA was not observed. Systematically higher leg and arm SMM values were observed with DXA as compared to MFBIA. Conclusions While DXA and MFBIA total SMM generally exhibited strong agreement, higher appendicular SMM by DXA highlights technical differences between methods.
更多查看译文
关键词
Medical imaging,Nutrition,Medicine/Public Health,general,Public Health,Epidemiology,Internal Medicine,Clinical Nutrition,Metabolic Diseases
AI 理解论文
溯源树
样例
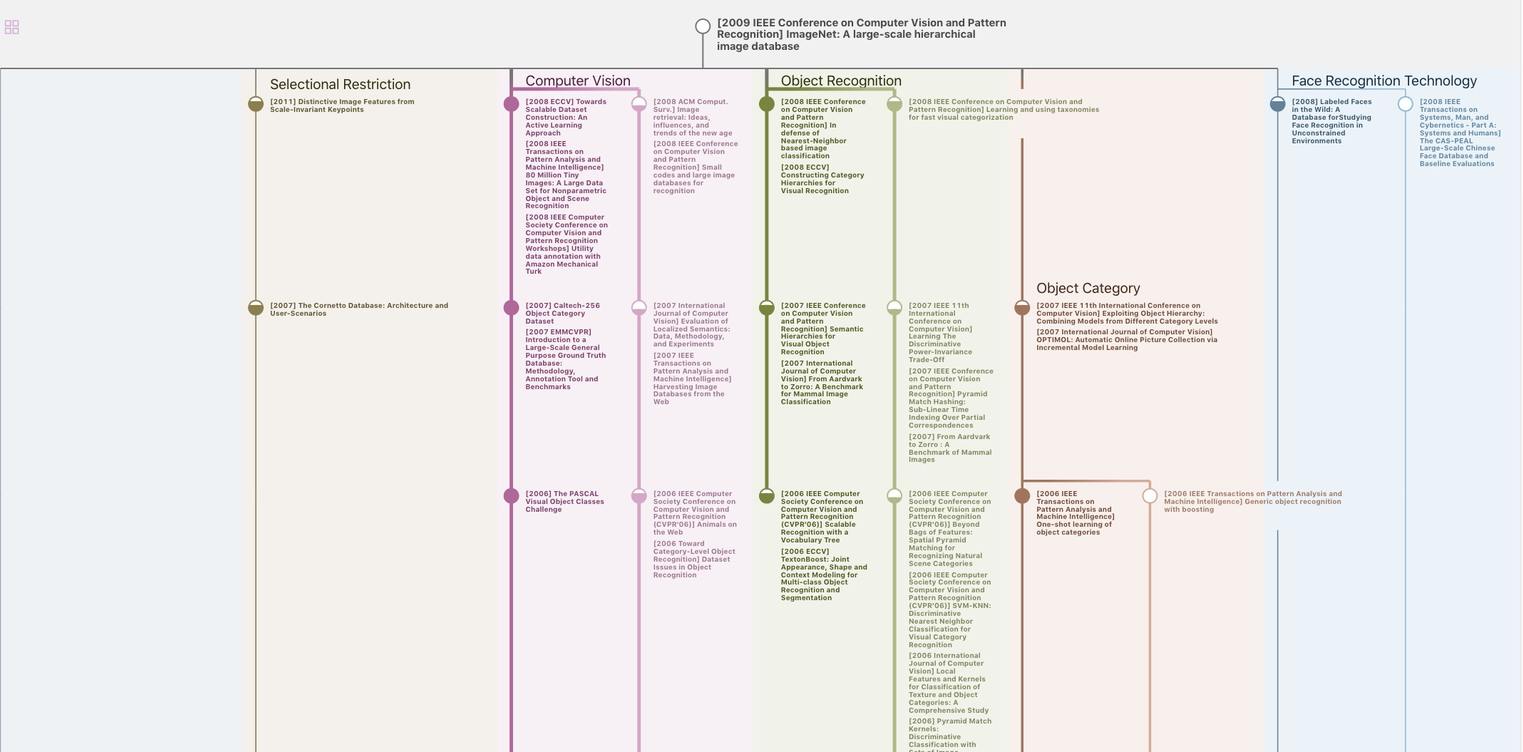
生成溯源树,研究论文发展脉络
Chat Paper
正在生成论文摘要