HimGNN: a Novel Hierarchical Molecular Graph Representation Learning Framework for Property Prediction.
BRIEFINGS IN BIOINFORMATICS(2023)
摘要
Accurate prediction of molecular properties is an important topic in drug discovery. Recent works have developed various representation schemes for molecular structures to capture different chemical information in molecules. The atom and motif can be viewed as hierarchical molecular structures that are widely used for learning molecular representations to predict chemical properties. Previous works have attempted to exploit both atom and motif to address the problem of information loss in single representation learning for various tasks. To further fuse such hierarchical information, the correspondence between learned chemical features from different molecular structures should be considered. Herein, we propose a novel framework for molecular property prediction, called hierarchical molecular graph neural networks (HimGNN). HimGNN learns hierarchical topology representations by applying graph neural networks on atom- and motif-based graphs. In order to boost the representational power of the motif feature, we design a Transformer-based local augmentation module to enrich motif features by introducing heterogeneous atom information in motif representation learning. Besides, we focus on the molecular hierarchical relationship and propose a simple yet effective rescaling module, called contextual self-rescaling, that adaptively recalibrates molecular representations by explicitly modelling interdependencies between atom and motif features. Extensive computational experiments demonstrate that HimGNN can achieve promising performances over state-of-the-art baselines on both classification and regression tasks in molecular property prediction.
更多查看译文
关键词
Homology Modeling,Materials Discovery,Drug Target Identification,Computational Chemistry
AI 理解论文
溯源树
样例
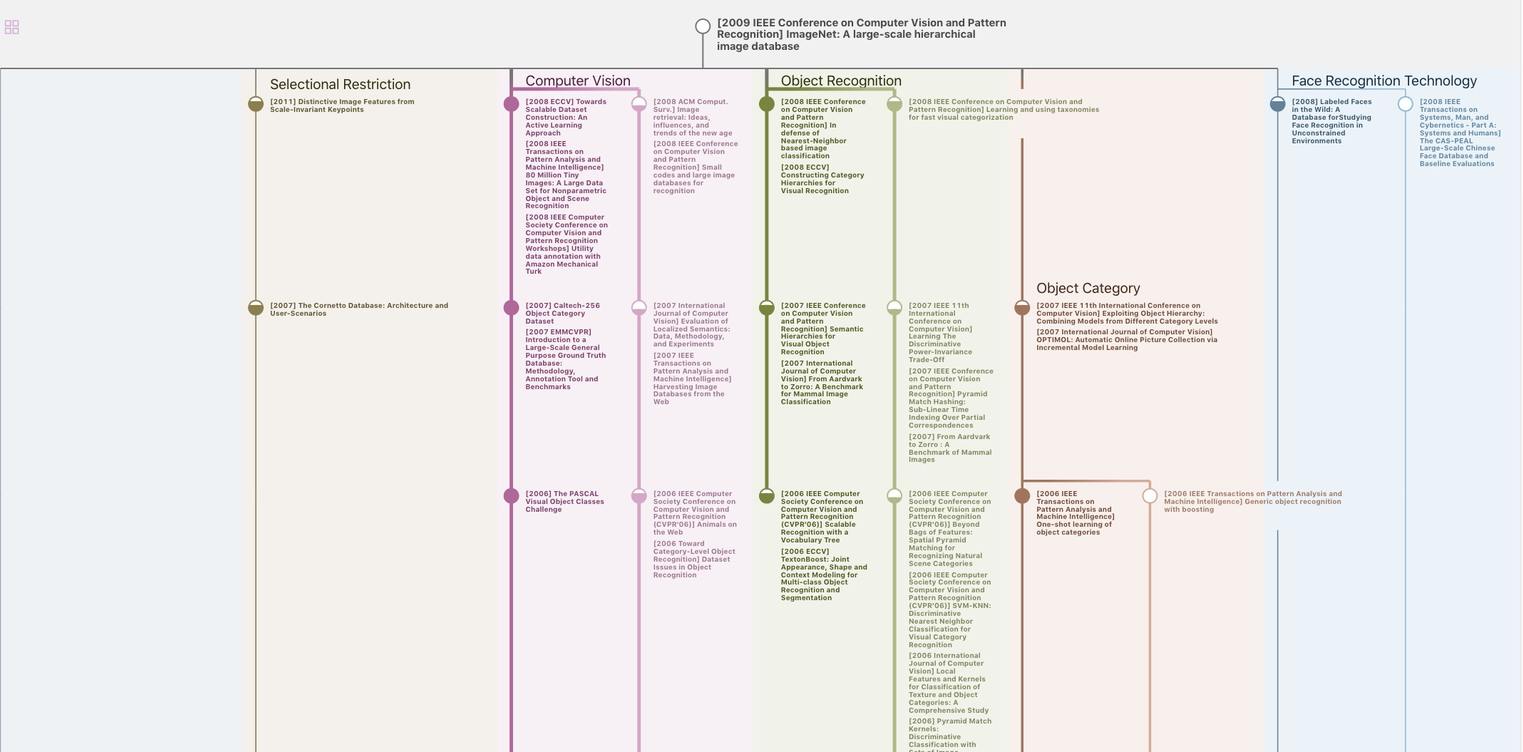
生成溯源树,研究论文发展脉络
Chat Paper
正在生成论文摘要