Accelerating the Discovery of Hybrid Perovskites with Targeted Band Gaps via Interpretable Machine Learning
ACS applied materials & interfaces(2023)
摘要
The band gap of hybrid organic-inorganic perovskites(HOIPs)is a key factor affecting the light absorption characteristics andthus the performance of perovskite solar cells (PSCs). However, bandgap engineering, using experimental trial and error and high-throughputdensity functional theory calculations, is blind and costly. Therefore,it is critical to statistically identify the multiple factors influencingband gaps and to rationally design perovskites with targeted bandgaps. This study combined feature engineering, the gradient-boostedregression tree (GBRT) algorithm, and the genetic algorithm-basedsymbolic regression (GASR) algorithm to develop an interpretable machinelearning (ML) strategy for predicting the band gap of HOIPs accuratelyand quantitatively interpreting the factors affecting the band gap.Seven best physical features were selected to construct a GBRT modelwith a root-mean-square error of less than 0.060 eV, and the mostimportant feature is the electronegativity difference between theB-site and the X-site (& chi;(B-X)). Further, a mathematicalformula (E ( g ) = & chi;(B-X) (2) + 0.881 & chi;(B-X))was constructed with GASR for a quantitative interpretation of theband gap influence patterns. According to the ML model, the HOIP MA(0.23)FA(0.02)Cs(0.75)Pb(0.59)Sn(0.41)Br(0.24)I(2.76) was obtained, with asuitable band gap of 1.39 eV. Our proposed interpretable ML strategyprovides an effective approach for developing HOIP structures withtargeted band gaps, which can also be applied to other material fields.
更多查看译文
关键词
perovskite solar cell,band gap,machine learning,GBRT,symbolic regression
AI 理解论文
溯源树
样例
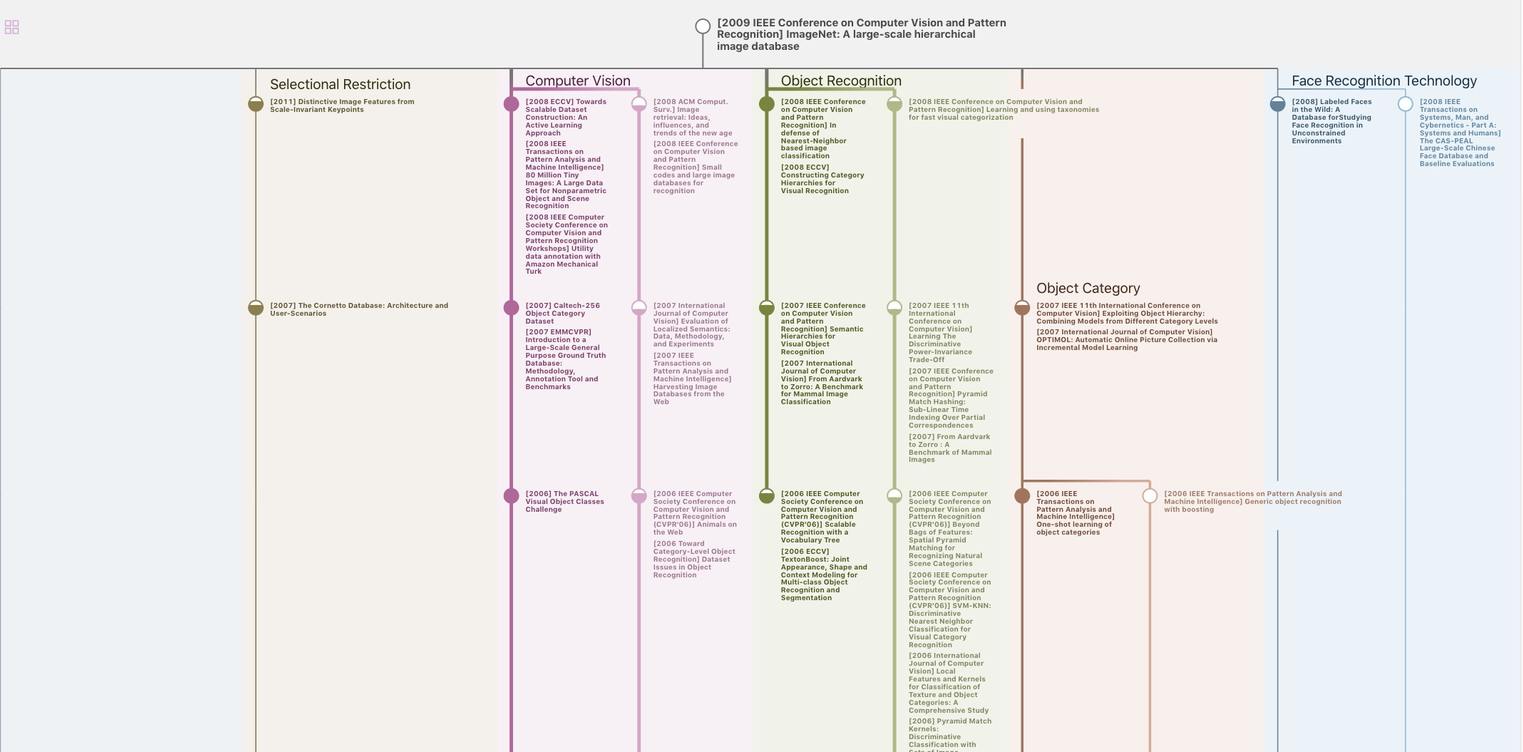
生成溯源树,研究论文发展脉络
Chat Paper
正在生成论文摘要