Can diffusion tensor imaging have a diagnostic utility to differentiate early-onset forms of bipolar disorder and schizophrenia: A neuroimaging study with explainable machine learning algorithms
PSYCHIATRY RESEARCH-NEUROIMAGING(2023)
摘要
Background/aim: Accurate diagnosis of early-onset psychotic disorders is crucial to improve clinical outcomes. This study aimed to differentiate patients with early-onset schizophrenia (EOS) from early-onset bipolar disorder (EBD) with machine learning (ML) algorithms using white matter tracts (WMT).Method: Diffusion tensor imaging was obtained from adolescents with either EOS (n = 43) or EBD (n = 32). Global probabilistic tractography using an automated tract-based TRACULA software was performed to analyze the fractional anisotropy (FA) of forty-two WMT. The nested cross-validation was performed in feature selection and model construction. EXtreme Gradient Boosting (XGBoost) was applied to select the features that can give the best performance in the ML model. The interpretability of the model was explored with the SHApley Additive exPlanations (SHAP).Findings: The XGBoost algorithm identified nine out of the 42 major WMTs with significant predictive power. Among ML models, Support Vector Machine-Linear showed the best performance. Higher SHAP values of left acoustic radiation, bilateral anterior thalamic radiation, and the corpus callosum were associated with a higher likelihood of EOS.Conclusions: Our findings suggested that ML models based on the FA values of major WMT reconstructed by global probabilistic tractography can unveil hidden microstructural aberrations to distinguish EOS from EBD.
更多查看译文
关键词
Early-onset schizophrenia,Early-onset bipolar disorder,Diffusion tensor imaging,Machine learning
AI 理解论文
溯源树
样例
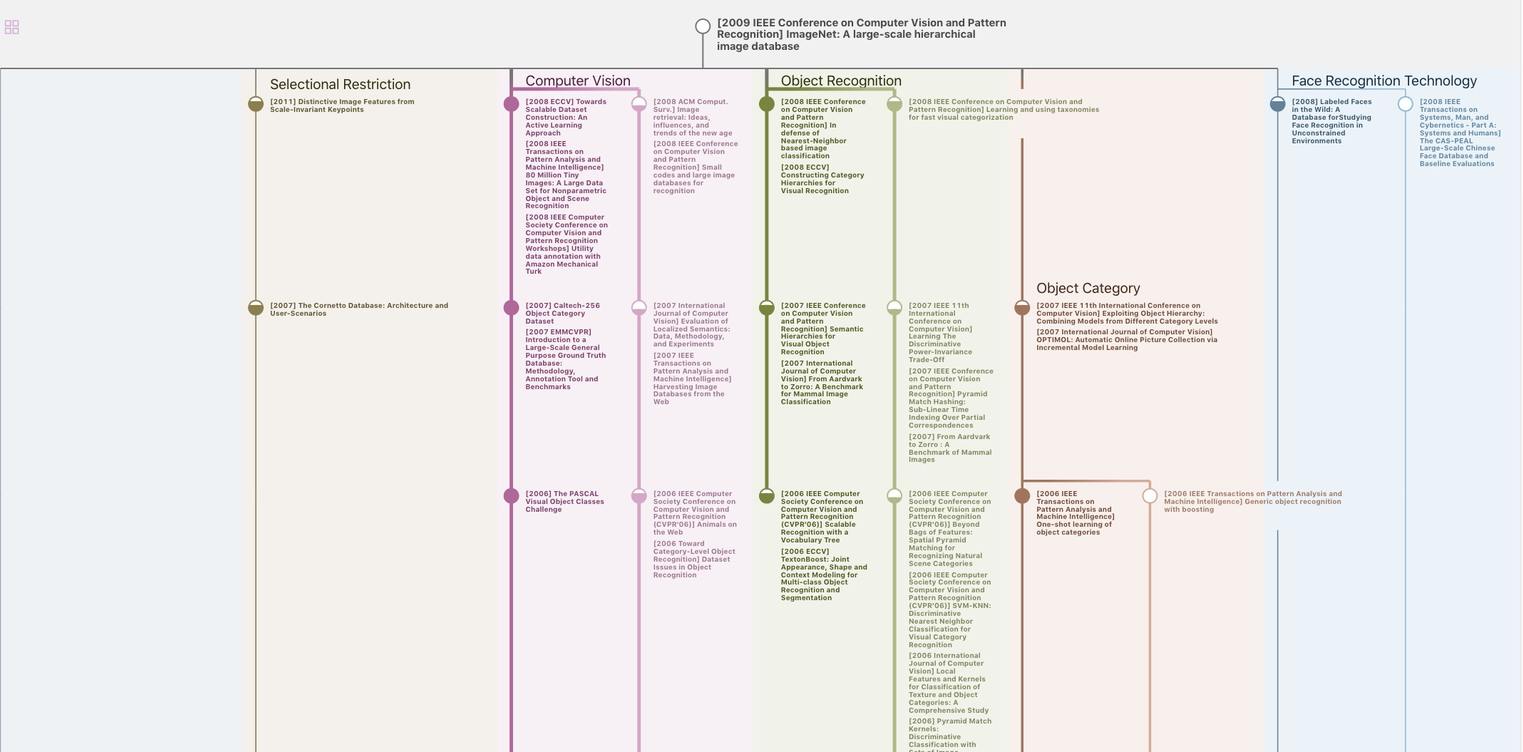
生成溯源树,研究论文发展脉络
Chat Paper
正在生成论文摘要