Simulation Evidence of Trust Calibration: Using POMDP with Signal Detection Theory to Adapt Agent Features for Optimised Task Outcome During Human-Agent Collaboration
International Journal of Social Robotics(2023)
摘要
Appropriately calibrated human trust is essential for successful Human-Agent collaboration. Probabilistic frameworks using a partially observable Markov decision process (POMDP) have been previously employed to model the trust dynamics of human behavior, optimising the outcomes of a task completed with a collaborative recommender system. A POMDP model utilising signal detection theory to account for latent user trust is presented, with the model working to calibrate user trust via the implementation of three distinct agent features: disclaimer message, request for additional information, and no additional feature. A simulation experiment is run to investigate the efficacy of the proposed POMDP model compared against a random feature model and a control model. Evidence demonstrates that the proposed POMDP model can appropriately adapt agent features in-task based on human trust belief estimates in order to achieve trust calibration. Specifically, task accuracy is highest with the POMDP model, followed by the control and then the random model. This emphasises the importance of trust calibration, as agents that lack considered design to implement features in an appropriate way can be more detrimental to task outcome compared to an agent with no additional features.
更多查看译文
关键词
Human-agent collaboration,Recommender system,Trust calibration,Partially observable Markov decision process,Signal detection theory
AI 理解论文
溯源树
样例
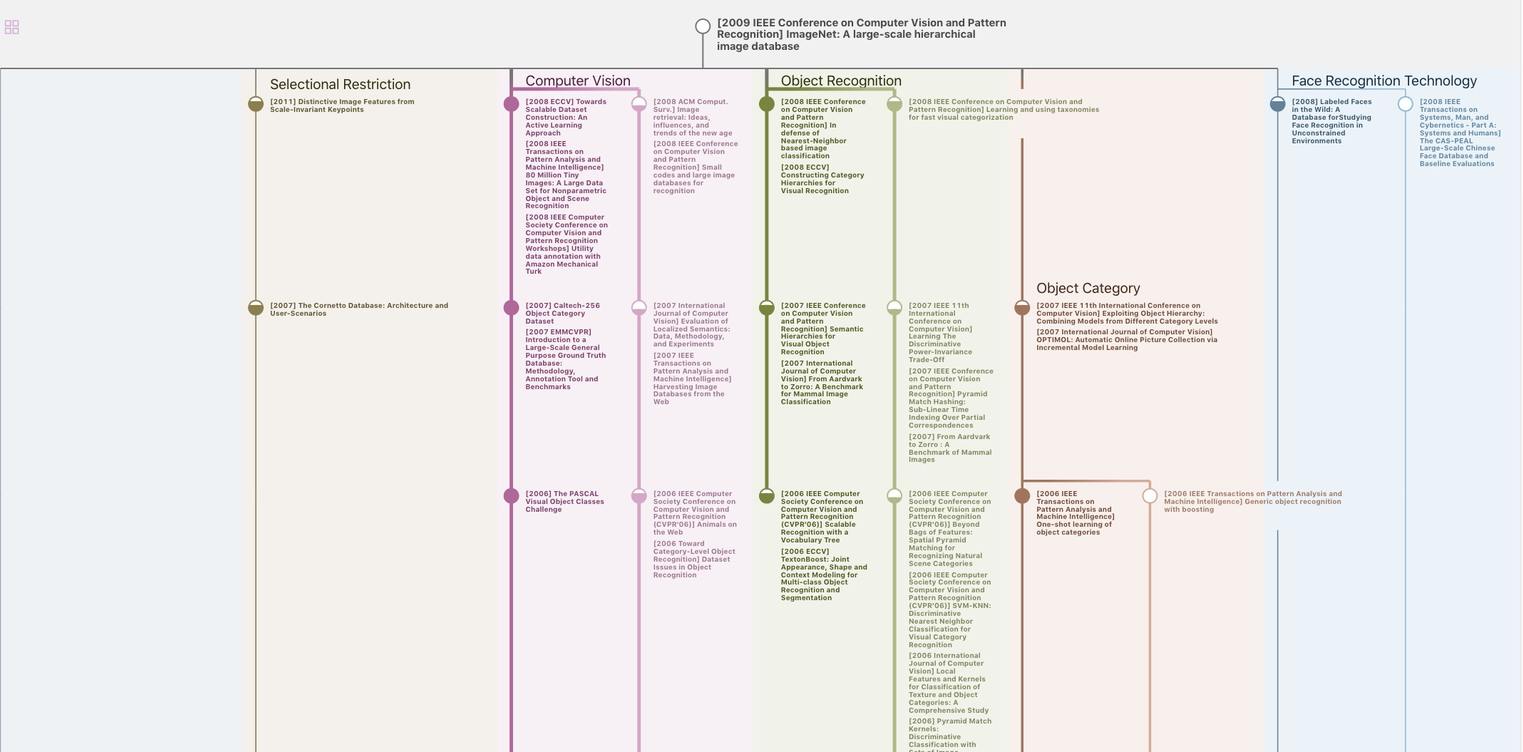
生成溯源树,研究论文发展脉络
Chat Paper
正在生成论文摘要