A Biologically-Inspired Sparse Self-Representation Approach for Projected Fuzzy Double C-Means Clustering
Cognitive Computation(2023)
摘要
Data redundancy is frequently encountered in biologically data. Locality preserving projection (LPP) is a dimensionality reduction approach to mitigate the data redundancy while preserving the substantial geometry inspired by biological processes. Its application can contribute promisingly to the fuzzy c-means (FCM) clustering. However, the existing locality preserving based FCM clustering methods that combine LPP with FCM focus only on the local information, probably resulting in somewhat conservatism. A novel FCM clustering method, namely, projected fuzzy double c-means clustering using sparse self-representation (PFD SSR), is developed in this paper. The main idea of PFD SSR is three-fold: (1) Inspired by biological processes, a so-called sparse self-representation (SSR) method is employed. Hence, the global data distribution is investigated so as to enhance the clustering performance; (2) LPP is utilized to handle both the raw data and the dictionary matrix obtained by SSR, which greatly reduces the feature dimensions and solidly preserves the intrinsic data distribution. In addition, the regularization terms of these two achievements under projection are introduced to the FCM’s objective function, which helps reduce the risk of being trapped into local optima during the model training; and (3) the alternative direction technique is applied to learn the model. The experimental results on 11 datasets including 6 biologically data sets demonstrated the proposed method outperforms the state-of-art clustering methods. The proposed subspace clustering method has a good ability of handling the high-dimensional data, especially biological data.
更多查看译文
关键词
Fuzzy c-means clustering,Dimension reduction,Locality preserving projection,Sparse self-representation,Regularization
AI 理解论文
溯源树
样例
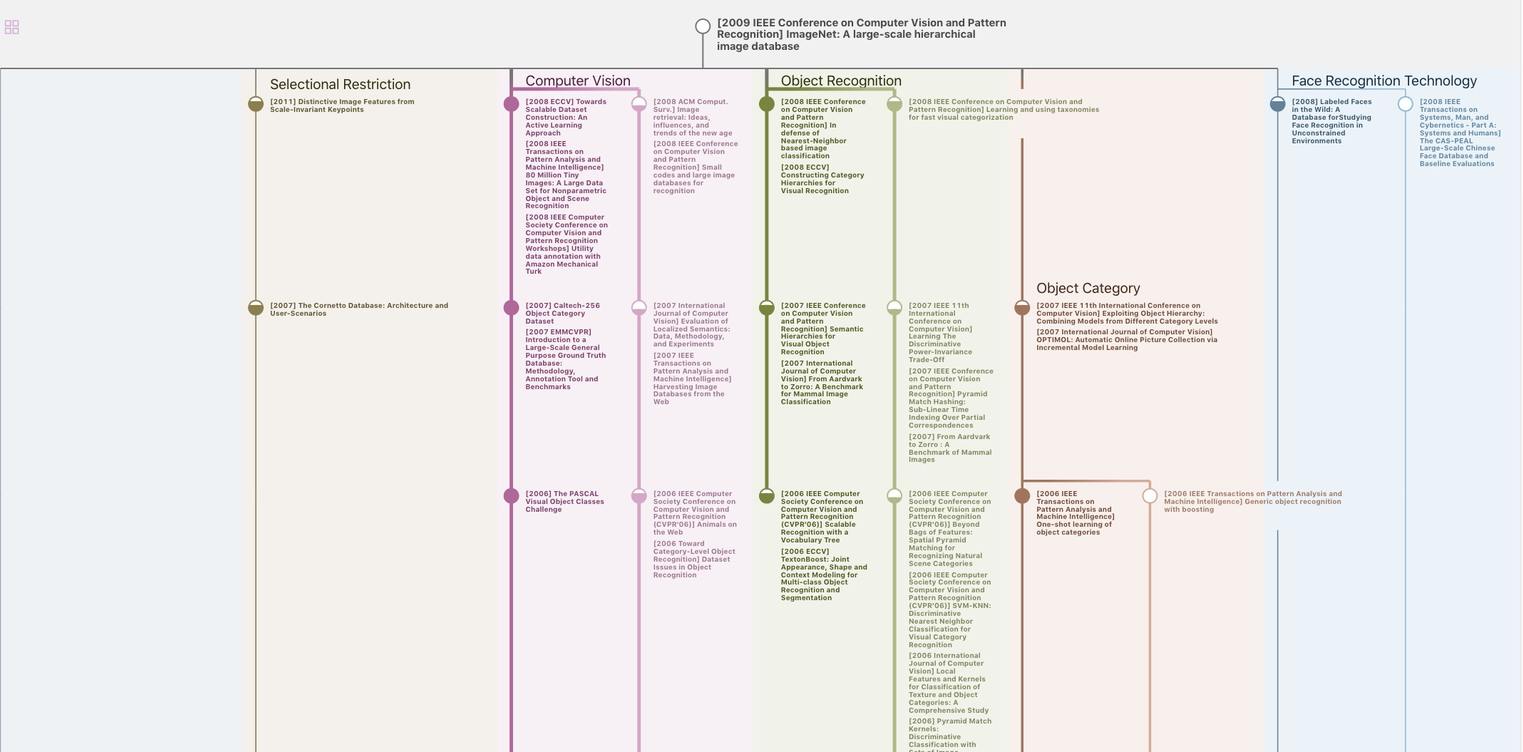
生成溯源树,研究论文发展脉络
Chat Paper
正在生成论文摘要