Deep Contextualised Text Representation and Learning for Sarcasm Detection
Arabian Journal for Science and Engineering(2024)
摘要
These days, the world has become a non-denominational place due to cyberspace. Digital services such as search portals and social platforms have become intricate with the regular course of daily life. As a massive number of clients’ thoughts are available on the internet, sentiment analysis has become one of the most prolific investigation arenas in natural language processing. Sarcasm detection is a vital phase in sentiment analysis because of the intrinsically vague nature of sarcasm. To subsidize as a resolution to the current ever-increasing arena of attention on the ever-increasing volume of digital data, this work proposes a novel voting-based ensemble approach for sarcasm detection utilizing deep learning techniques. This paper uses Bidirectional Encoder Representations from Transformers to build contextual word embeddings and feeds those to the network, which is an ensemble of four deep learning models. Convolutional Neural Network, Bidirectional Long Short-Term Memory, and parallel and sequential combinations of both are among the models. For classification, the acquired features from each model are experimented with four machine learning classifiers, such as Support Vector Machine, Least Squares Support Vector Machine, Multinomial Naive Bayes, and Random Forest, along with Sigmoid activation function. Out of these five, the optimal classifier is selected for each model. Finally, a majority voting is performed on the outputs of all four models to differentiate between sarcastic and non-sarcastic texts. The proposed approach has been assessed using two benchmark datasets containing English-language texts, namely, news headlines and the self-annotated reddit corpus. Experiments demonstrated that the proposed methodology produces an accuracy of 94.89% on the news headlines repository, which is a gain of 2.99%, and an F 1-score of 80.49% on the self-annotated reddit corpus, which is an improvement of 0.52% over the previous state-of-the-art methodologies.
更多查看译文
关键词
Sentiment analysis,Sarcasm detection,Contextual word embeddings,Majority voting
AI 理解论文
溯源树
样例
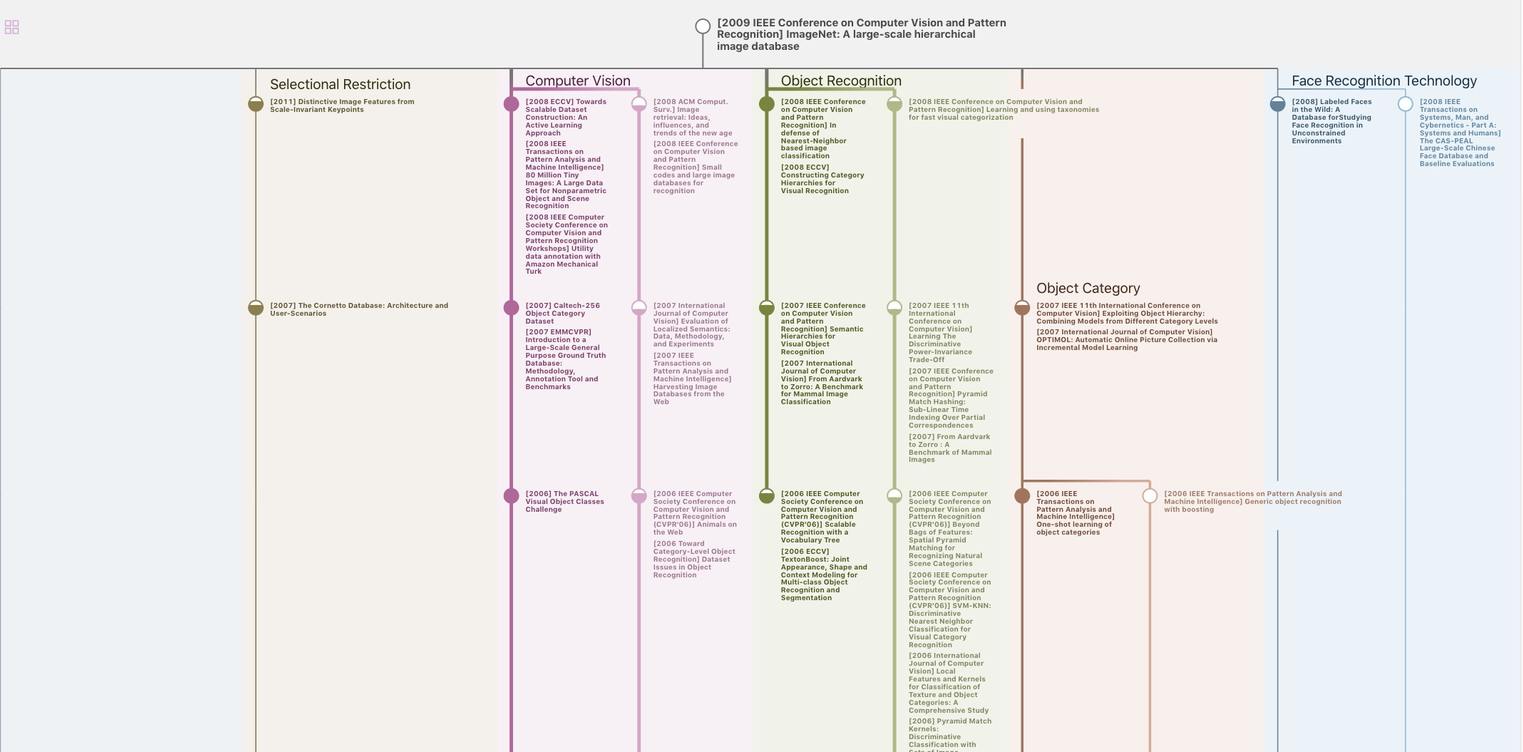
生成溯源树,研究论文发展脉络
Chat Paper
正在生成论文摘要