Segmentation of pectoral muscle from mammograms using U-Net having densely connected convolutional layers
Multimedia Tools and Applications(2024)
摘要
Segmentation of the pectoral muscle is one of the most fundamental steps in developing a Computer-Aided Diagnosis (CAD) System. Considering the presence of various artifacts and homogeneity among the breast region, the pectoral muscle segmentation is a relatively tricky task. This study proposes a modified U-Net structure for automatic pectoral muscle segmentation from mammogram images. The modified U-Net architecture is different from the traditional U-Net architecture in having densely connected convolution layers. The proposed architecture was trained, validated, and tested by utilizing 322 and 200 mammogram images from two publicly available datasets: Mammographic Image Analysis Society (mini-MIAS) and the INBreast. Mini-MIAS contains scanned filmed mammograms, whereas INBreast contains full-field digital (FFDM) mammograms. Unlike other segmentation techniques, the modified U-Net architecture proposed does not require any pre-processing method and performs equally well on both scanned filmed and FFDM mammograms. We tested the system by fusing both the above datasets to verify our claim. Our proposed architecture has obtained satisfactory results on the mixed dataset. The experimental results showed that the pectoral muscle segmentation approach received a dice coefficient of 96.38 ± 1.52 and 96.82 ± 2.23 on mini-MIAS and the INBreast databases, respectively. Moreover on mixing both the datasets and randomly testing 20% of the samples, the algorithm achieved a Dice coefficient of 95.82 ± 1.23.
更多查看译文
关键词
Breast cancer,Digital mammogram,Pectoral muscle segmentation,U-Net
AI 理解论文
溯源树
样例
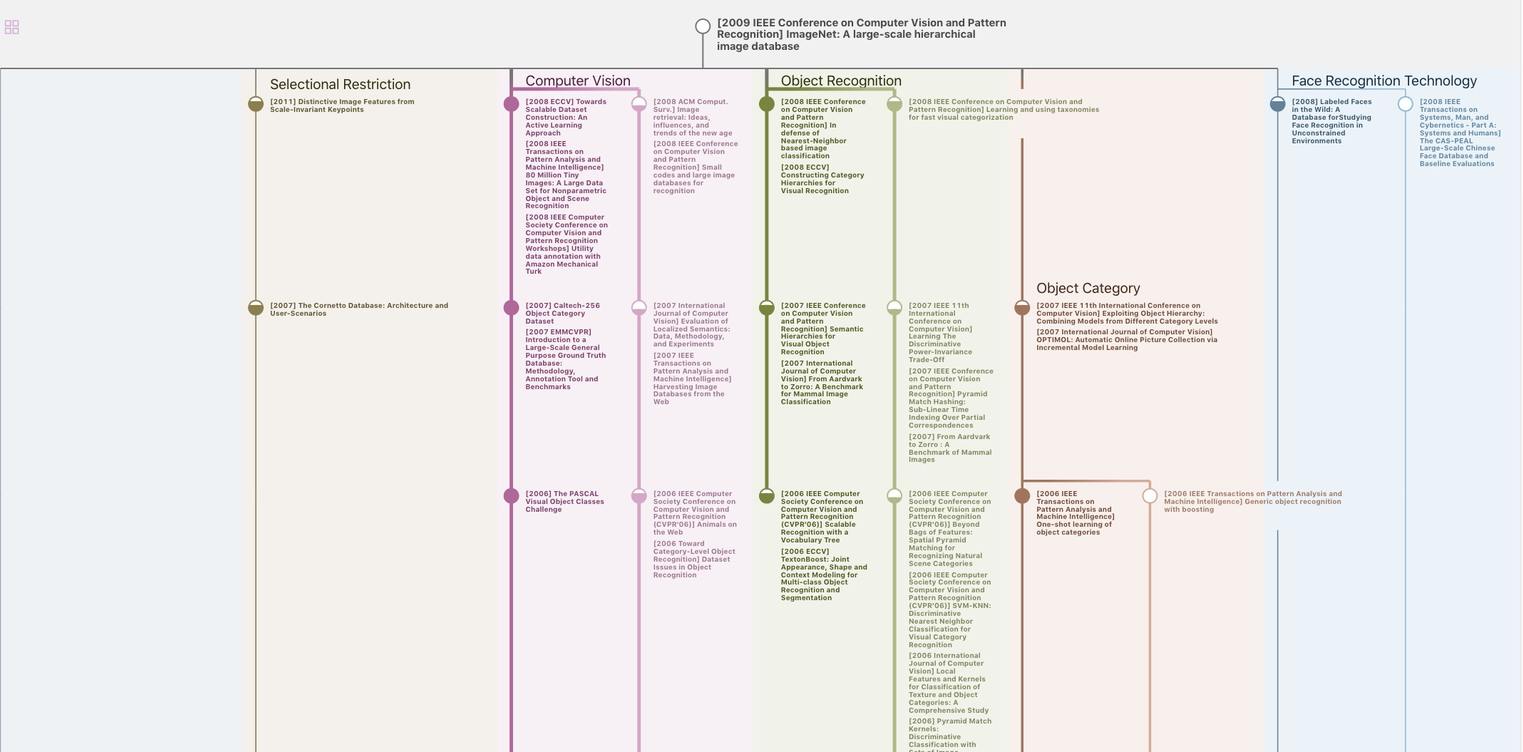
生成溯源树,研究论文发展脉络
Chat Paper
正在生成论文摘要