How to train a neural network potential
JOURNAL OF CHEMICAL PHYSICS(2023)
摘要
The introduction of modern Machine Learning Potentials (MLPs) has led to a paradigm change in the development of potential energy surfaces for atomistic simulations. By providing efficient access to energies and forces, they allow us to perform large-scale simulations of extended systems, which are not directly accessible by demanding first-principles methods. In these simulations, MLPs can reach the accuracy of electronic structure calculations, provided that they have been properly trained and validated using a suitable set of reference data. Due to their highly flexible functional form, the construction of MLPs has to be done with great care. In this Tutorial, we describe the necessary key steps for training reliable MLPs, from data generation via training to final validation. The procedure, which is illustrated for the example of a high-dimensional neural network potential, is general and applicable to many types of MLPs.
更多查看译文
关键词
neural network,potential
AI 理解论文
溯源树
样例
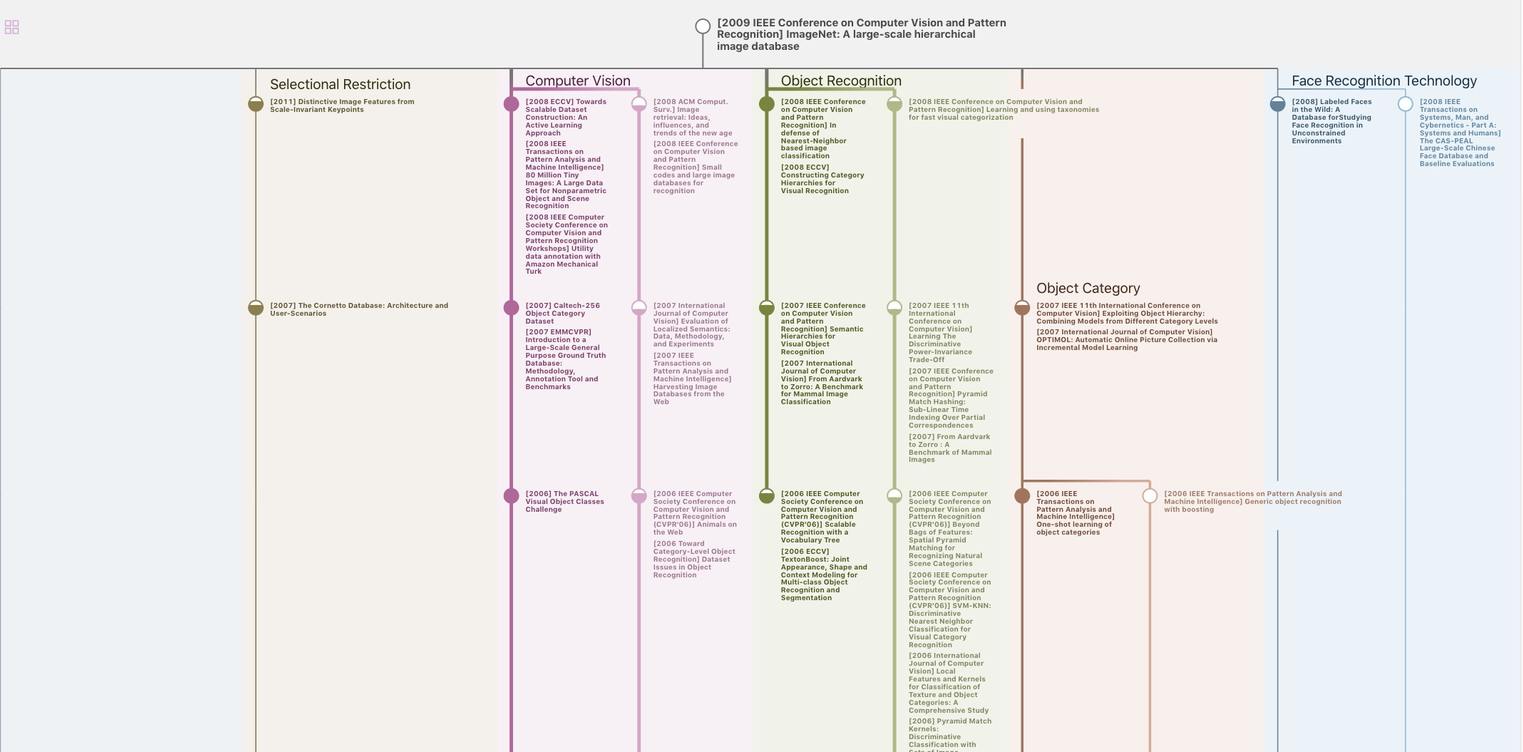
生成溯源树,研究论文发展脉络
Chat Paper
正在生成论文摘要