Multi-FedLS: a Framework for Cross-Silo Federated Learning Applications on Multi-Cloud Environments
CoRR(2023)
摘要
Federated Learning (FL) is a distributed Machine Learning (ML) technique that can benefit from cloud environments while preserving data privacy. We propose Multi-FedLS, a framework that manages multi-cloud resources, reducing execution time and financial costs of Cross-Silo Federated Learning applications by using preemptible VMs, cheaper than on-demand ones but that can be revoked at any time. Our framework encloses four modules: Pre-Scheduling, Initial Mapping, Fault Tolerance, and Dynamic Scheduler. This paper extends our previous work \cite{brum2022sbac} by formally describing the Multi-FedLS resource manager framework and its modules. Experiments were conducted with three Cross-Silo FL applications on CloudLab and a proof-of-concept confirms that Multi-FedLS can be executed on a multi-cloud composed by AWS and GCP, two commercial cloud providers. Results show that the problem of executing Cross-Silo FL applications in multi-cloud environments with preemptible VMs can be efficiently resolved using a mathematical formulation, fault tolerance techniques, and a simple heuristic to choose a new VM in case of revocation.
更多查看译文
关键词
learning,framework,multi-fedls,cross-silo,multi-cloud
AI 理解论文
溯源树
样例
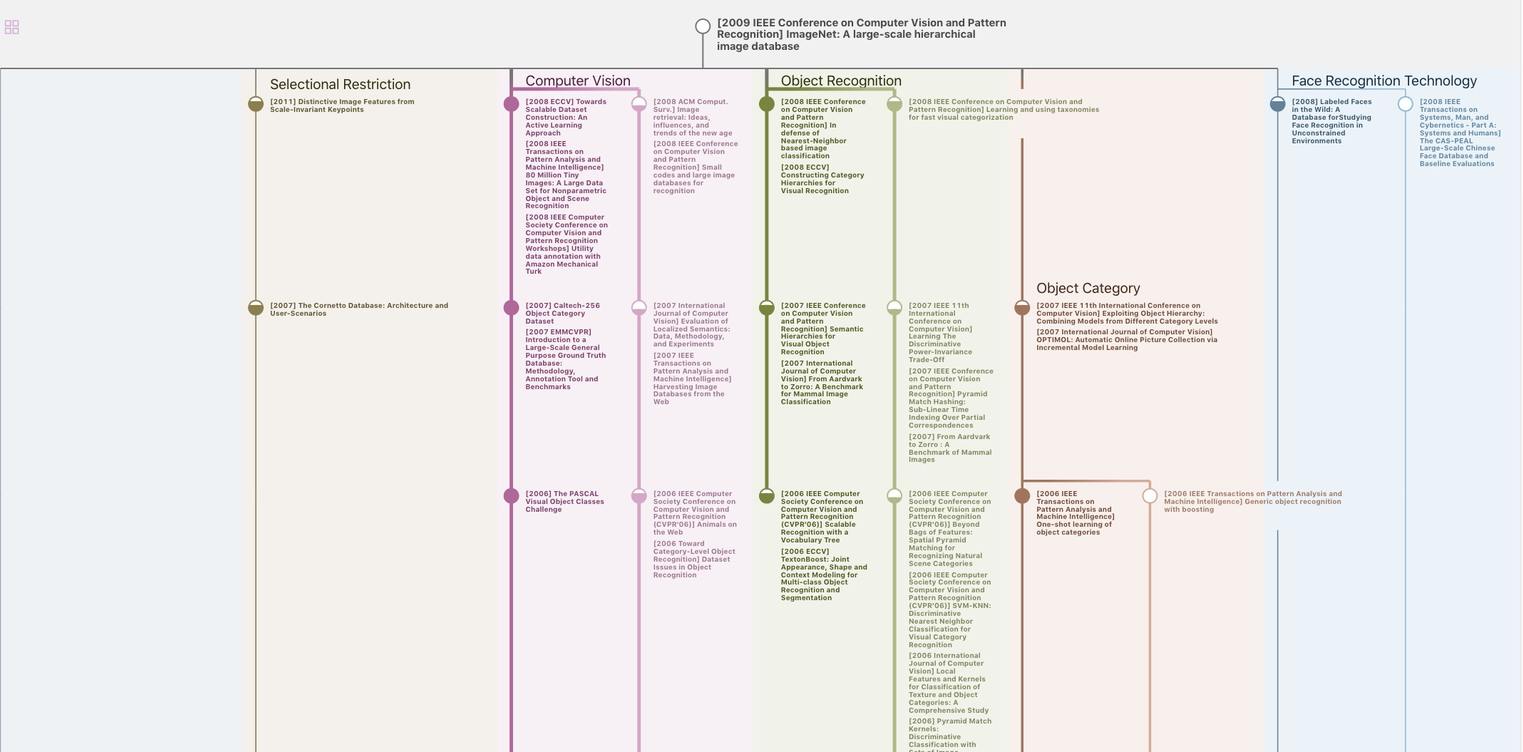
生成溯源树,研究论文发展脉络
Chat Paper
正在生成论文摘要