Quantification of ciprofloxacin in pharmaceutical products from various brands using FT-NIR: A comparative investigation of PLS and MCR-ALS.
Spectrochimica acta. Part A, Molecular and biomolecular spectroscopy(2023)
摘要
This study aims to quantify ciprofloxacin in commercial tablets with varying excipient compositions using Fourier Transform Near-Infrared Spectroscopy (FT-NIR) and chemometric models: Partial Least Squares (PLS) and Multivariate Curve Resolution - Alternating Least Squares (MCR-ALS). Matrix variation, arising from differences in excipient compositions among the tablets, can impact quantification accuracy. We discuss this phenomenon, emphasizing potential issues introduced by varying certain excipients and its importance in reliable ciprofloxacin quantification. We evaluated the performance of PLS and MCR-ALS models independently on two sets of tablets, each containing the same drug substance but different excipients. The statistical results revealed promising results with PLS prediction error of 0.38% w/w of the first set and 0.47% w/w of the second set, while MCR-ALS achieved prediction errors of 0.67% w/w of the first set and 1.76% w/w of the second set. To address the challenge of matrix variation, we developed single models for PLS and MCR-ALS using a dataset combining both first and second sets. The PLS single model demonstrated a prediction error of 4.3% w/w and a relative error of 6.41% w/w, while the MCR-ALS single model showed a prediction error of 1.88% w/w and a relative error of 1.29% w/w. We then assessed the performance of the single PLS and MCR-ALS models developed based on the combination of the first and the second set in quantifying ciprofloxacin in various commercial tablet brands containing new excipients. The PLS model achieved a prediction error ranging between 6.2% w/w and 8.39% w/w, with relative errors varied between 8.53% w/w and 12.82% w/w. On the other hand, the MCR-ALS model had a prediction error between 1.11% w/w and 2.66% w/w, and the relative errors ranging from 0.8% to 1.74% w/w.
更多查看译文
AI 理解论文
溯源树
样例
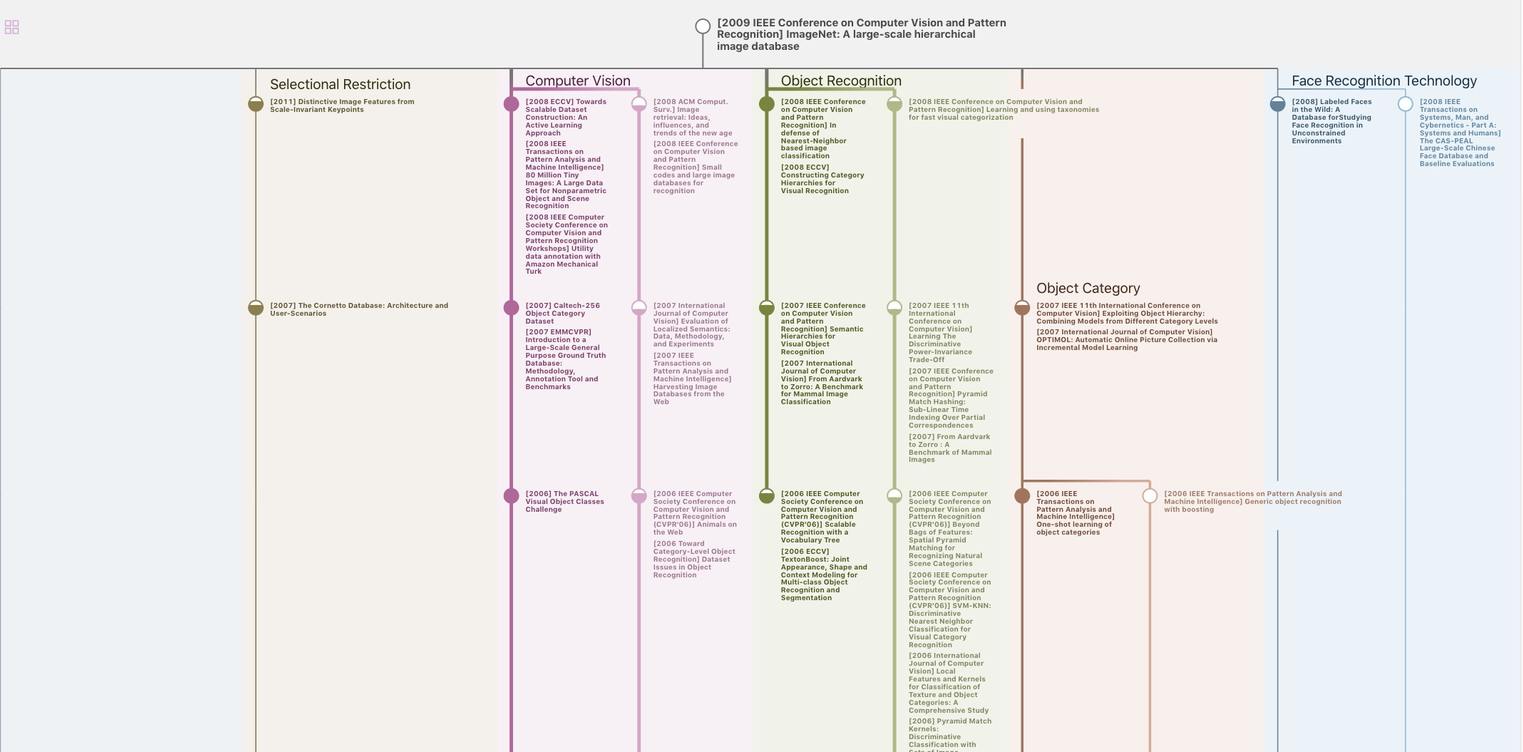
生成溯源树,研究论文发展脉络
Chat Paper
正在生成论文摘要