H3-OPT: Accurate Prediction of CDR-H3 Loop Structures of Antibodies with Deep Learning
eLife(2024)
摘要
Accurate prediction of the structurally diverse complementarity determining region heavy chain 3 (CDR-H3) loop structure remains a primary and long-standing challenge for antibody modeling. Here, we present the H3-OPT toolkit for predicting the 3D structures of monoclonal antibodies and nanobodies. H3-OPT combines the strengths of AlphaFold2 with a pre-trained protein language model, and provides a 2.24 Å average RMSD Cα between predicted and experimentally determined CDR-H3 loops, thus outperforming other current computational methods in our non-redundant high-quality dataset. The model was validated by experimentally solving three structures of anti-VEGF nanobodies predicted by H3-OPT. We examined the potential applications of H3-OPT through analyzing antibody surface properties and antibody-antigen interactions. This structural prediction tool can be used to optimize antibody-antigen binding, and to engineer therapeutic antibodies with biophysical properties for specialized drug administration route.
更多查看译文
关键词
Antibody Engineering
AI 理解论文
溯源树
样例
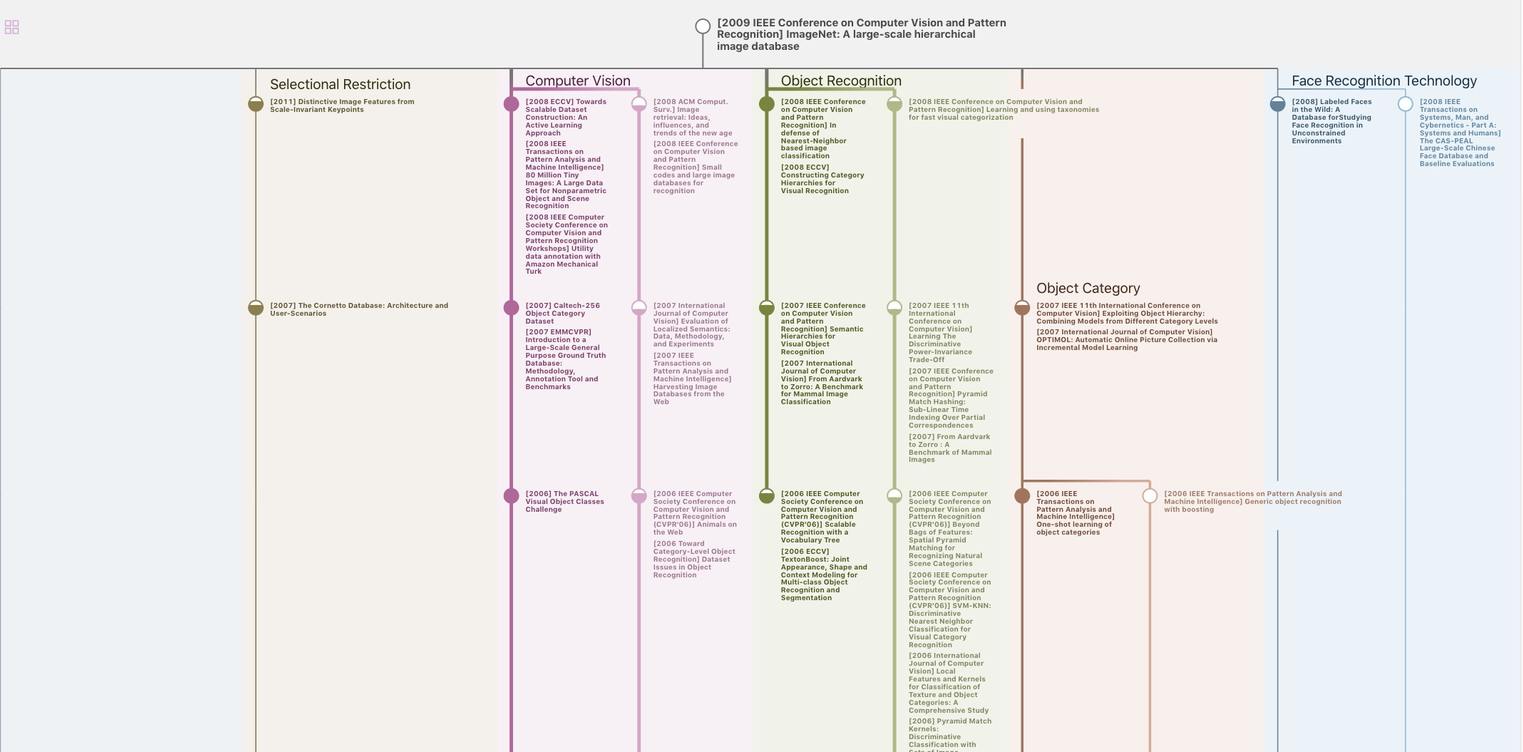
生成溯源树,研究论文发展脉络
Chat Paper
正在生成论文摘要