Evaluating quantum generative models via imbalanced data classification benchmarks
CoRR(2023)
摘要
A limited set of tools exist for assessing whether the behavior of quantum machine learning models diverges from conventional models, outside of abstract or theoretical settings. We present a systematic application of explainable artificial intelligence techniques to analyze synthetic data generated from a hybrid quantum-classical neural network adapted from twenty different real-world data sets, including solar flares, cardiac arrhythmia, and speech data. Each of these data sets exhibits varying degrees of complexity and class imbalance. We benchmark the quantum-generated data relative to state-of-the-art methods for mitigating class imbalance for associated classification tasks. We leverage this approach to elucidate the qualities of a problem that make it more or less likely to be amenable to a hybrid quantum-classical generative model.
更多查看译文
关键词
generative models,quantum,data classification
AI 理解论文
溯源树
样例
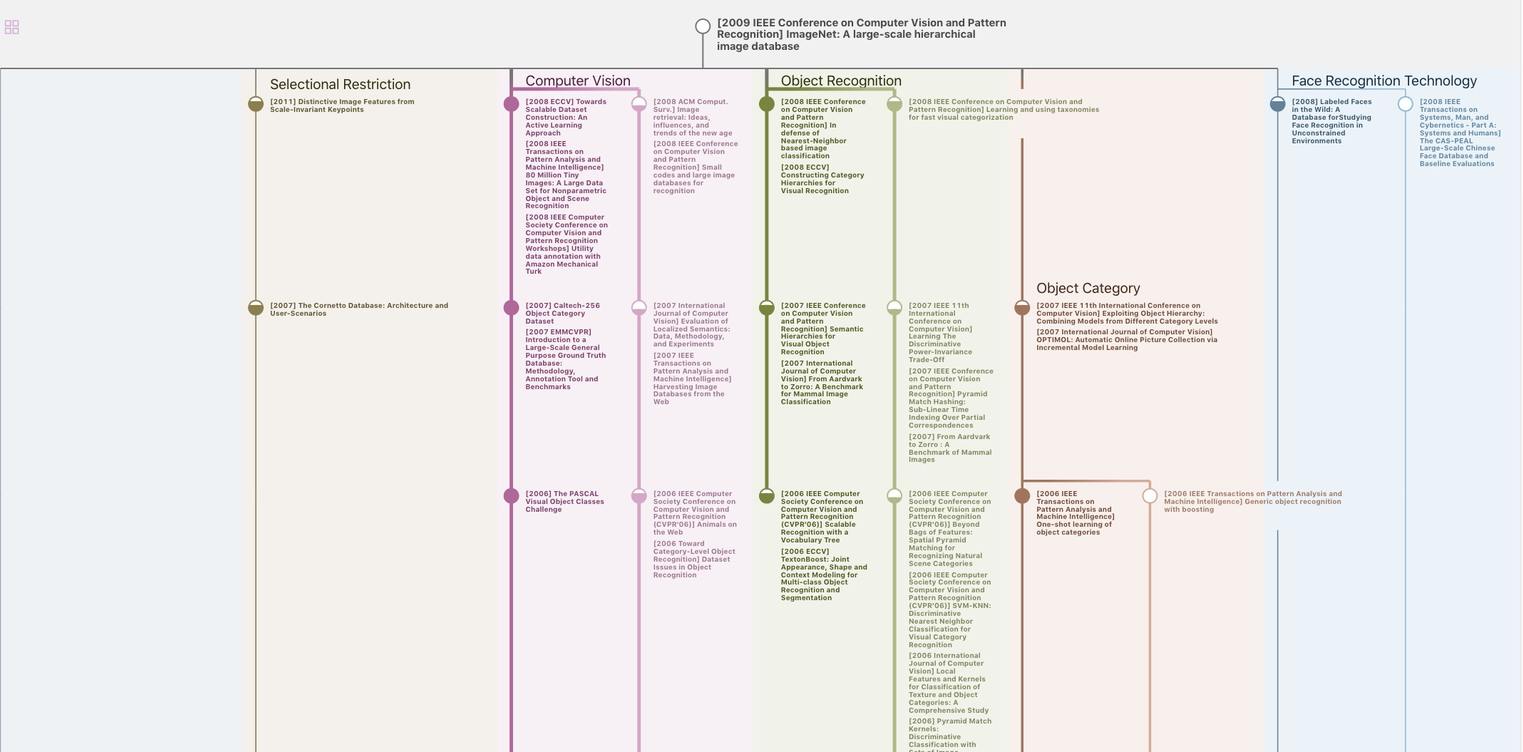
生成溯源树,研究论文发展脉络
Chat Paper
正在生成论文摘要