Unlocking Accuracy and Fairness in Differentially Private Image Classification
CoRR(2023)
摘要
Privacy-preserving machine learning aims to train models on private data without leaking sensitive information. Differential privacy (DP) is considered the gold standard framework for privacy-preserving training, as it provides formal privacy guarantees. However, compared to their non-private counterparts, models trained with DP often have significantly reduced accuracy. Private classifiers are also believed to exhibit larger performance disparities across subpopulations, raising fairness concerns. The poor performance of classifiers trained with DP has prevented the widespread adoption of privacy preserving machine learning in industry. Here we show that pre-trained foundation models fine-tuned with DP can achieve similar accuracy to non-private classifiers, even in the presence of significant distribution shifts between pre-training data and downstream tasks. We achieve private accuracies within a few percent of the non-private state of the art across four datasets, including two medical imaging benchmarks. Furthermore, our private medical classifiers do not exhibit larger performance disparities across demographic groups than non-private models. This milestone to make DP training a practical and reliable technology has the potential to widely enable machine learning practitioners to train safely on sensitive datasets while protecting individuals' privacy.
更多查看译文
关键词
private image,fairness,classification
AI 理解论文
溯源树
样例
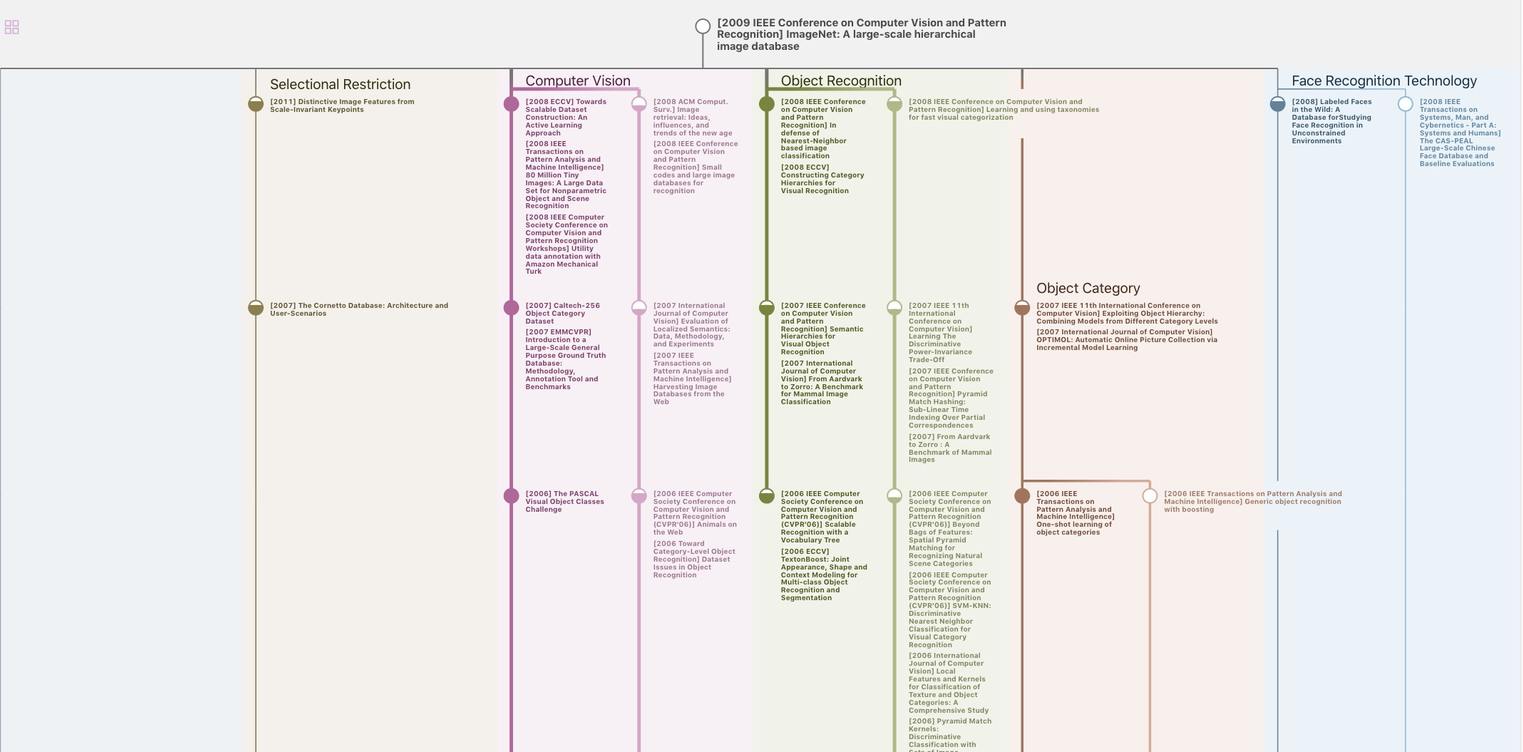
生成溯源树,研究论文发展脉络
Chat Paper
正在生成论文摘要