Using iterative random forest to find geospatial environmental and Sociodemographic predictors of suicide attempts
Frontiers in psychiatry(2023)
摘要
IntroductionDespite a recent global decrease in suicide rates, death by suicide has increased in the United States. It is therefore imperative to identify the risk factors associated with suicide attempts to combat this growing epidemic. In this study, we aim to identify potential risk factors of suicide attempt using geospatial features in an Artificial intelligence framework. MethodsWe use iterative Random Forest, an explainable artificial intelligence method, to predict suicide attempts using data from the Million Veteran Program. This cohort incorporated 405,540 patients with 391,409 controls and 14,131 attempts. Our predictive model incorporates multiple climatic features at ZIP-code-level geospatial resolution. We additionally consider demographic features from the American Community Survey as well as the number of firearms and alcohol vendors per 10,000 people to assess the contributions of proximal environment, access to means, and restraint decrease to suicide attempts. In total 1,784 features were included in the predictive model. ResultsOur results show that geographic areas with higher concentrations of married males living with spouses are predictive of lower rates of suicide attempts, whereas geographic areas where males are more likely to live alone and to rent housing are predictive of higher rates of suicide attempts. We also identified climatic features that were associated with suicide attempt risk by age group. Additionally, we observed that firearms and alcohol vendors were associated with increased risk for suicide attempts irrespective of the age group examined, but that their effects were small in comparison to the top features. DiscussionTaken together, our findings highlight the importance of social determinants and environmental factors in understanding suicide risk among veterans.
更多查看译文
关键词
iterative random forest,suicide,sociodemographic predictors
AI 理解论文
溯源树
样例
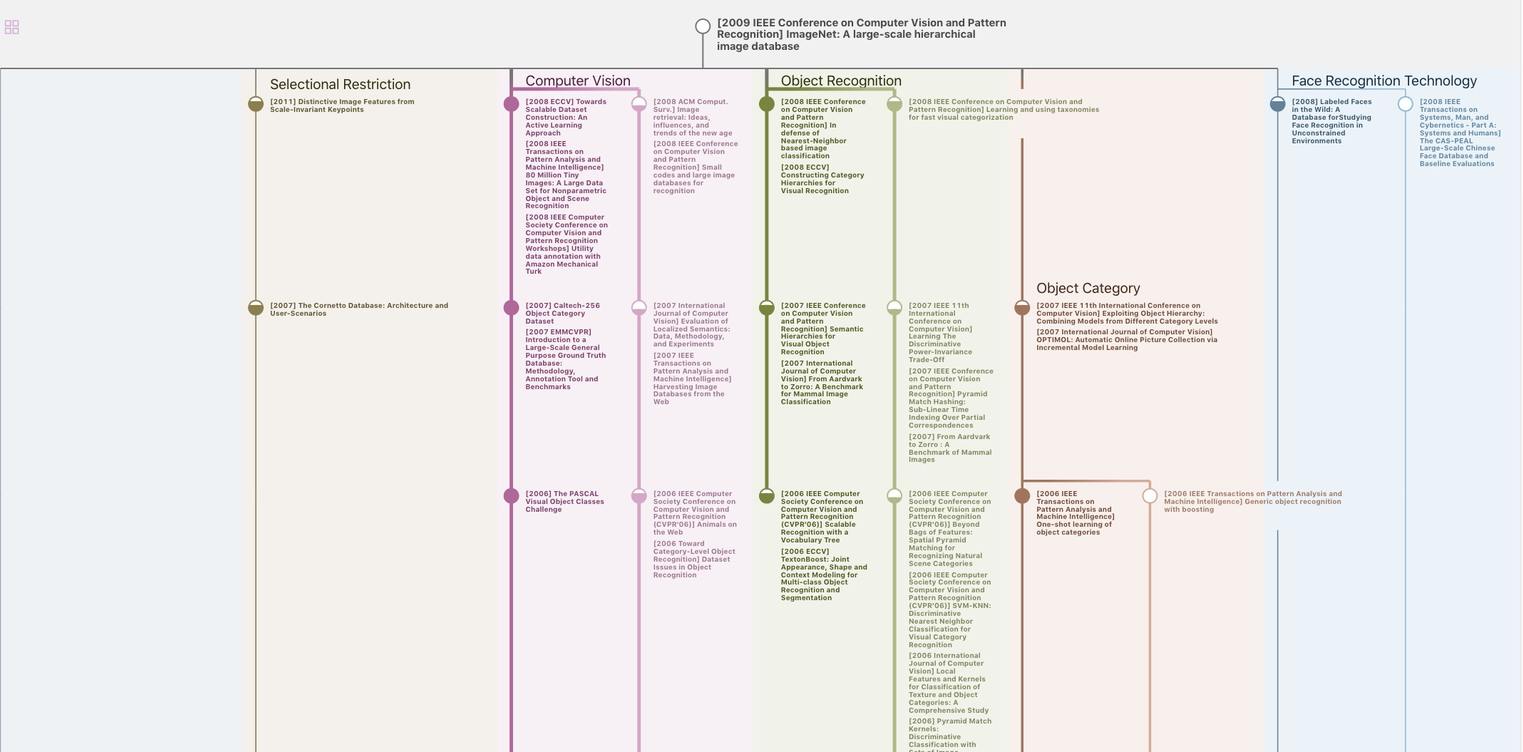
生成溯源树,研究论文发展脉络
Chat Paper
正在生成论文摘要