Understanding the host-pathogen evolutionary balance through Gaussian process modeling of SARS-CoV-2.
Patterns (New York, N.Y.)(2023)
摘要
We have developed a machine learning (ML) approach using Gaussian process (GP)-based spatial covariance (SCV) to track the impact of spatial-temporal mutational events driving host-pathogen balance in biology. We show how SCV can be applied to understanding the response of evolving covariant relationships linking the variant pattern of virus spread to pathology for the entire SARS-CoV-2 genome on a daily basis. We show that GP-based SCV relationships in conjunction with genome-wide co-occurrence analysis provides an early warning anomaly detection (EWAD) system for the emergence of variants of concern (VOCs). EWAD can anticipate changes in the pattern of performance of spread and pathology weeks in advance, identifying signatures destined to become VOCs. GP-based analyses of variation across entire viral genomes can be used to monitor micro and macro features responsible for host-pathogen balance. The versatility of GP-based SCV defines starting point for understanding nature's evolutionary path to complexity through natural selection.
更多查看译文
关键词
Gaussian processes,evolution,SARS-CoV-2,spatial covariance,early warning,host-pathogen,machine learning,genomic surveillance
AI 理解论文
溯源树
样例
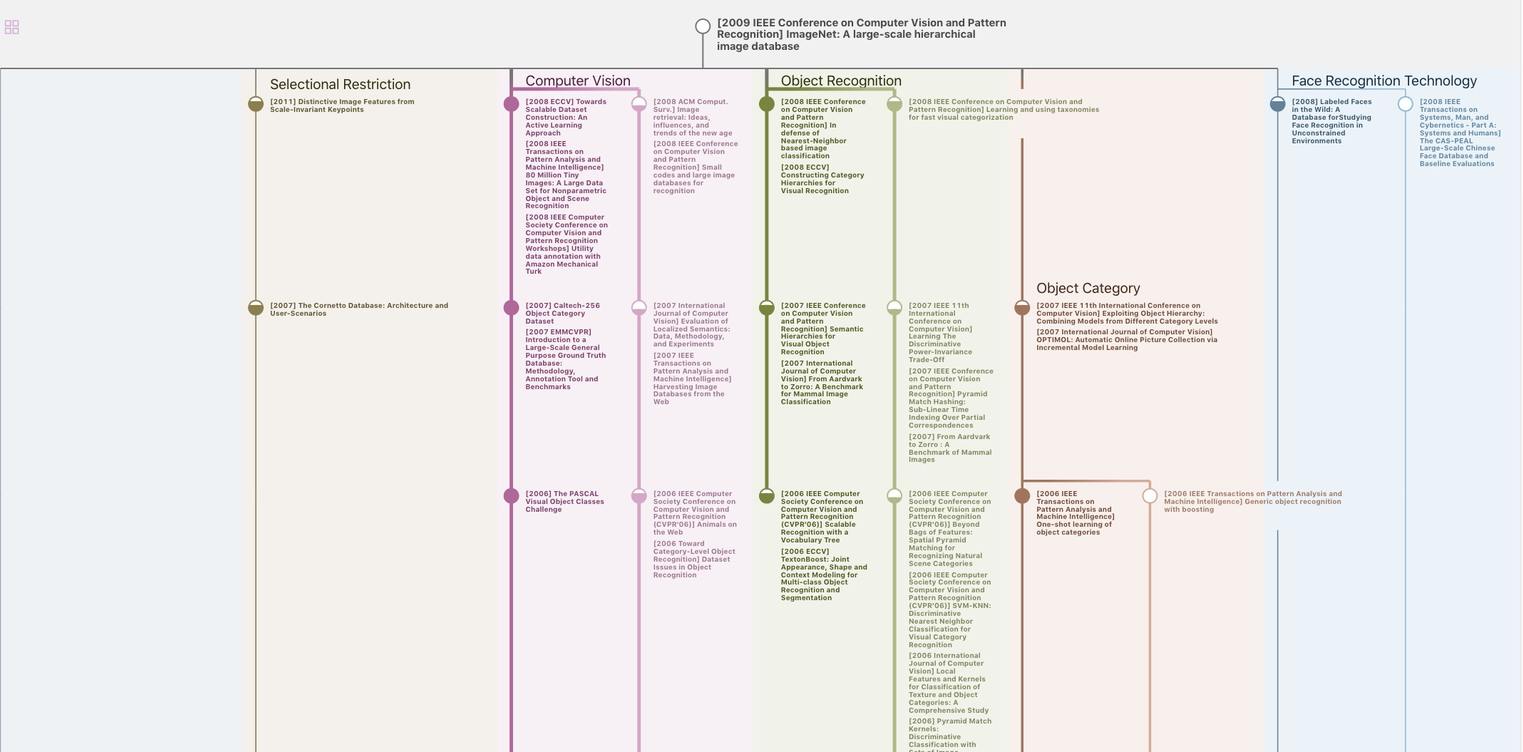
生成溯源树,研究论文发展脉络
Chat Paper
正在生成论文摘要