Stress representations for tensor basis neural networks: alternative formulations to Finger-Rivlin-Ericksen
CoRR(2023)
摘要
Data-driven constitutive modeling frameworks based on neural networks and classical representation theorems have recently gained considerable attention due to their ability to easily incorporate constitutive constraints and their excellent generalization performance. In these models, the stress prediction follows from a linear combination of invariant-dependent coefficient functions and known tensor basis generators. However, thus far the formulations have been limited to stress representations based on the classical Rivlin and Ericksen form, while the performance of alternative representations has yet to be investigated. In this work, we survey a variety of tensor basis neural network models for modeling hyperelastic materials in a finite deformation context, including a number of so far unexplored formulations which use theoretically equivalent invariants and generators to Finger-Rivlin-Ericksen. Furthermore, we compare potential-based and coefficient-based approaches, as well as different calibration techniques. Nine variants are tested against both noisy and noiseless datasets for three different materials. Theoretical and practical insights into the performance of each formulation are given.
更多查看译文
关键词
tensor basis,neural networks,finger-rivlin-ericksen
AI 理解论文
溯源树
样例
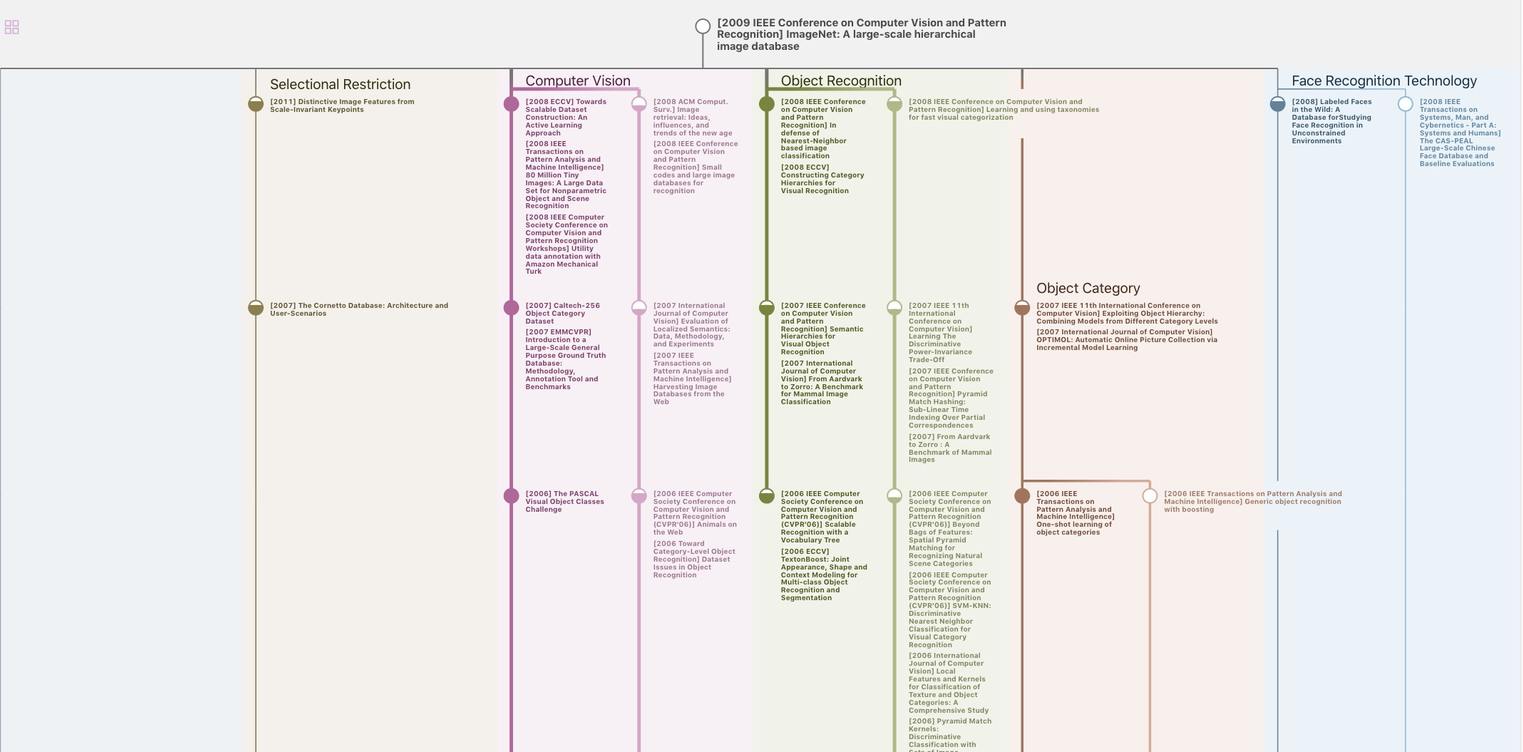
生成溯源树,研究论文发展脉络
Chat Paper
正在生成论文摘要