Decoupled Contrastive Multi-View Clustering with High-Order Random Walks
AAAI 2024(2024)
摘要
In recent, some robust contrastive multi-view clustering (MvC) methods have been proposed, which construct data pairs from neighborhoods to alleviate the false negative issue, i.e., some intra-cluster samples are wrongly treated as negative pairs. Although promising performance has been achieved by these methods, the false negative issue is still far from addressed and the false positive issue emerges because all in- and out-of-neighborhood samples are simply treated as positive and negative, respectively. To address the issues, we propose a novel robust method, dubbed decoupled contrastive multi-view clustering with high-order random walks (DIVIDE). In brief, DIVIDE leverages random walks to progressively identify data pairs in a global instead of local manner. As a result, DIVIDE could identify in-neighborhood negatives and out-of-neighborhood positives. Moreover, DIVIDE embraces a novel MvC architecture to perform inter- and intra-view contrastive learning in different embedding spaces, thus boosting clustering performance and embracing the robustness against missing views. To verify the efficacy of DIVIDE, we carry out extensive experiments on four benchmark datasets comparing with nine state-of-the-art MvC methods in both complete and incomplete MvC settings. The code is released on https://github.com/XLearning-SCU/2024-AAAI-DIVIDE.
更多查看译文
关键词
ML: Multi-instance/Multi-view Learning,ML: Clustering
AI 理解论文
溯源树
样例
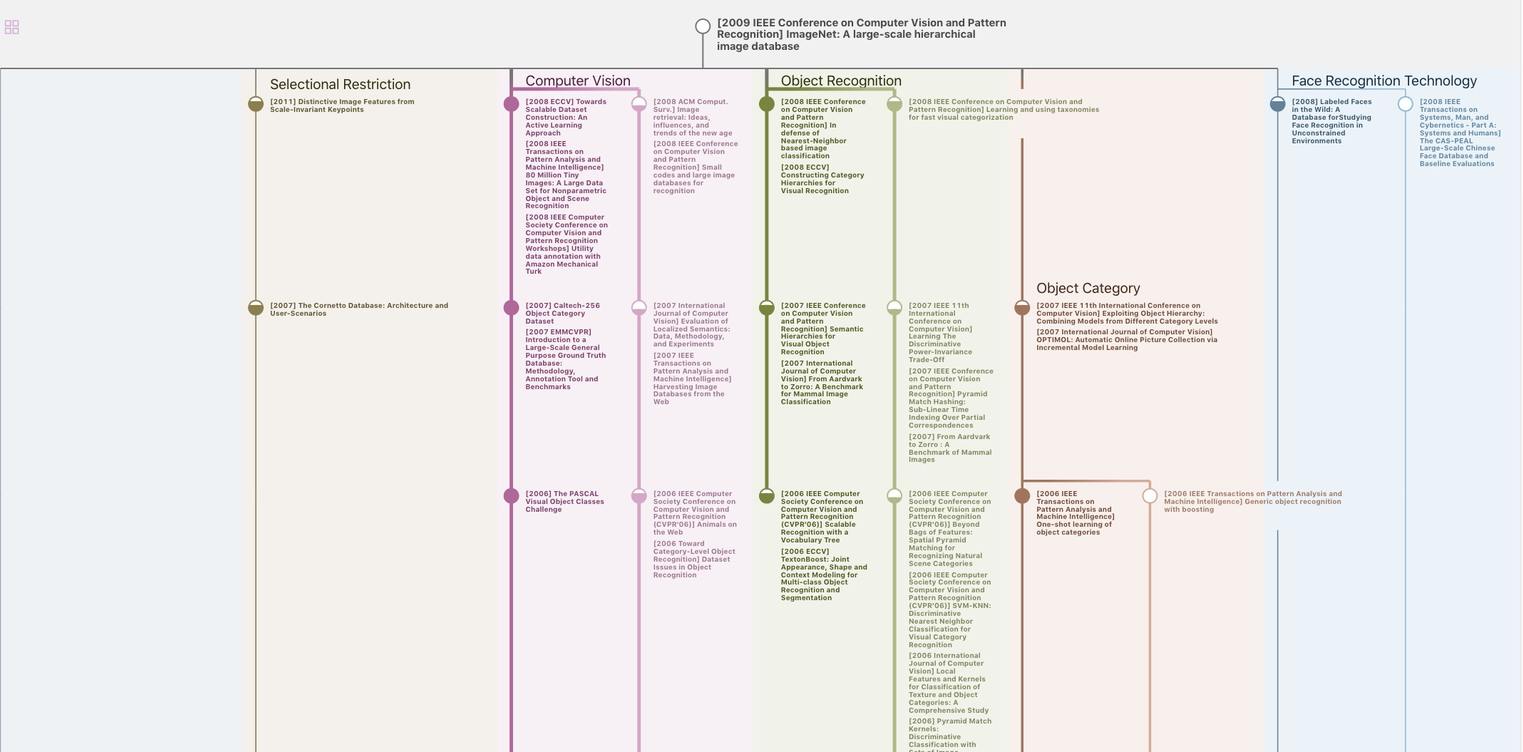
生成溯源树,研究论文发展脉络
Chat Paper
正在生成论文摘要