Computational Inference for Directions in Canonical Correlation Analysis
arXiv (Cornell University)(2023)
Abstract
Canonical Correlation Analysis (CCA) is a method for analyzing pairs of random vectors; it learns a sequence of paired linear transformations such that the resultant canonical variates are maximally correlated within pairs while uncorrelated across pairs. CCA outputs both canonical correlations as well as the canonical directions which define the transformations. While inference for canonical correlations is well developed, conducting inference for canonical directions is more challenging and not well-studied, but is key to interpretability. We propose a computational bootstrap method (combootcca) for inference on CCA directions. We conduct thorough simulation studies that range from simple and well-controlled to complex but realistic and validate the statistical properties of combootcca while comparing it to several competitors. We also apply the combootcca method to a brain imaging dataset and discover linked patterns in brain connectivity and behavioral scores.
MoreTranslated text
Key words
computational inference,correlation
AI Read Science
Must-Reading Tree
Example
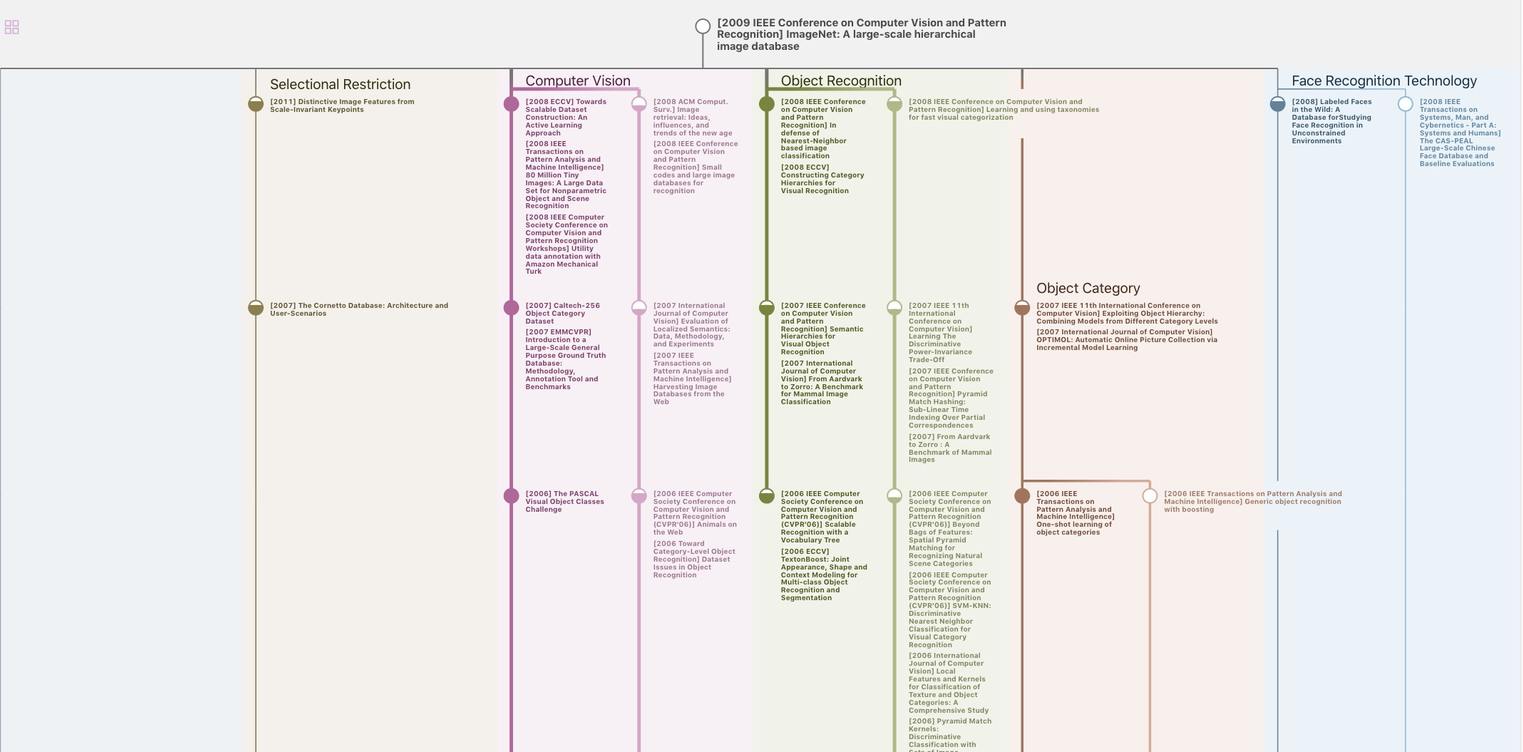
Generate MRT to find the research sequence of this paper
Chat Paper
Summary is being generated by the instructions you defined