Are current long-term video understanding datasets long-term?
2023 IEEE/CVF INTERNATIONAL CONFERENCE ON COMPUTER VISION WORKSHOPS, ICCVW(2023)
摘要
Many real-world applications, from sport analysis to surveillance, benefit from automatic long-term action recognition. In the current deep learning paradigm for automatic action recognition, it is imperative that models are trained and tested on datasets and tasks that evaluate if such models actually learn and reason over long-term information. In this work, we propose a method to evaluate how suitable a video dataset is to evaluate models for long-term action recognition. To this end, we define a long-term action as excluding all the videos that can be correctly recognized using solely short-term information. We test this definition on existing long-term classification tasks on three popular real-world datasets, namely Breakfast, CrossTask and LVU, to determine if these datasets are truly evaluating long-term recognition. Our study reveals that these datasets can be effectively solved using shortcuts based on short-term information. Following this finding, we encourage long-term action recognition researchers to make use of datasets that need long-term information to be solved.
更多查看译文
关键词
long-term,long-term
AI 理解论文
溯源树
样例
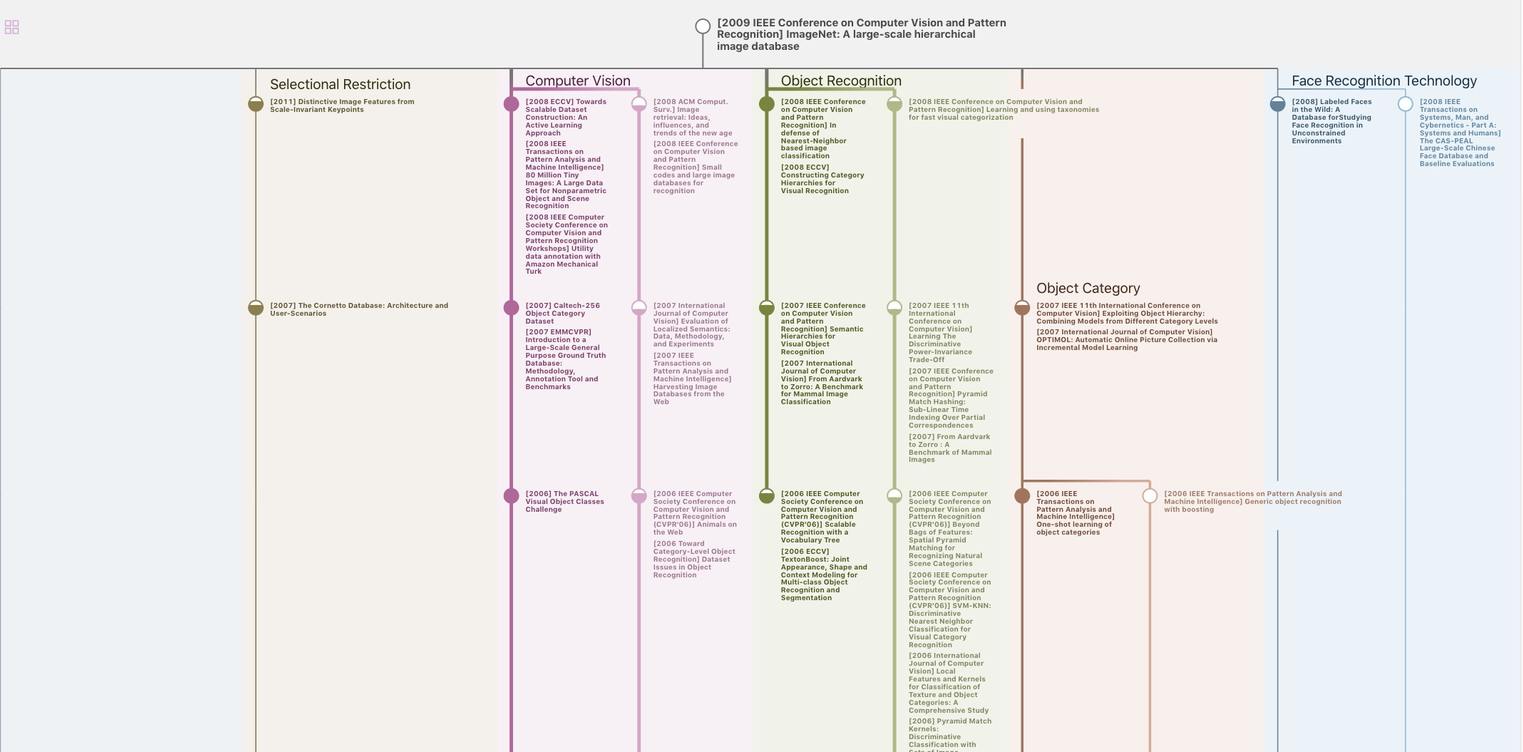
生成溯源树,研究论文发展脉络
Chat Paper
正在生成论文摘要