Boundary-RL: Reinforcement Learning for Weakly-Supervised Prostate Segmentation in TRUS Images
MACHINE LEARNING IN MEDICAL IMAGING, MLMI 2023, PT I(2024)
摘要
We propose Boundary-RL, a novel weakly supervised segmentation method that utilises only patch-level labels for training. We envision segmentation as a boundary detection problem, rather than a pixel-level classification as in previous works. This outlook on segmentation may allow for boundary delineation under challenging scenarios such as where noise artefacts may be present within the region-of-interest (ROI) boundaries, where traditional pixel-level classification-based weakly supervised methods may not be able to effectively segment the ROI. Particularly of interest, ultrasound images, where intensity values represent acoustic impedance differences between boundaries, may also benefit from the boundary delineation approach. Our method uses reinforcement learning to train a controller function to localise boundaries of ROIs using a reward derived from a pre-trained boundary-presence classifier. The classifier indicates when an object boundary is encountered within a patch, serving as weak supervision, as the controller modifies the patch location in a sequential Markov decision process. The classifier itself is trained using only binary patch-level labels of object presence, the only labels used during training of the entire boundary delineation framework. The use of a controller ensures that sliding window over the entire image is not necessary and reduces possible false-positives or -negatives by minimising number of patches passed to the boundary-presence classifier. We evaluate our approach for a clinically relevant task of prostate gland segmentation on trans-rectal ultrasound images. We show improved performance compared to other tested weakly supervised methods, using the same labels e.g., multiple instance learning.
更多查看译文
关键词
TRUS,Weak Supervision,Reinforcement Learning
AI 理解论文
溯源树
样例
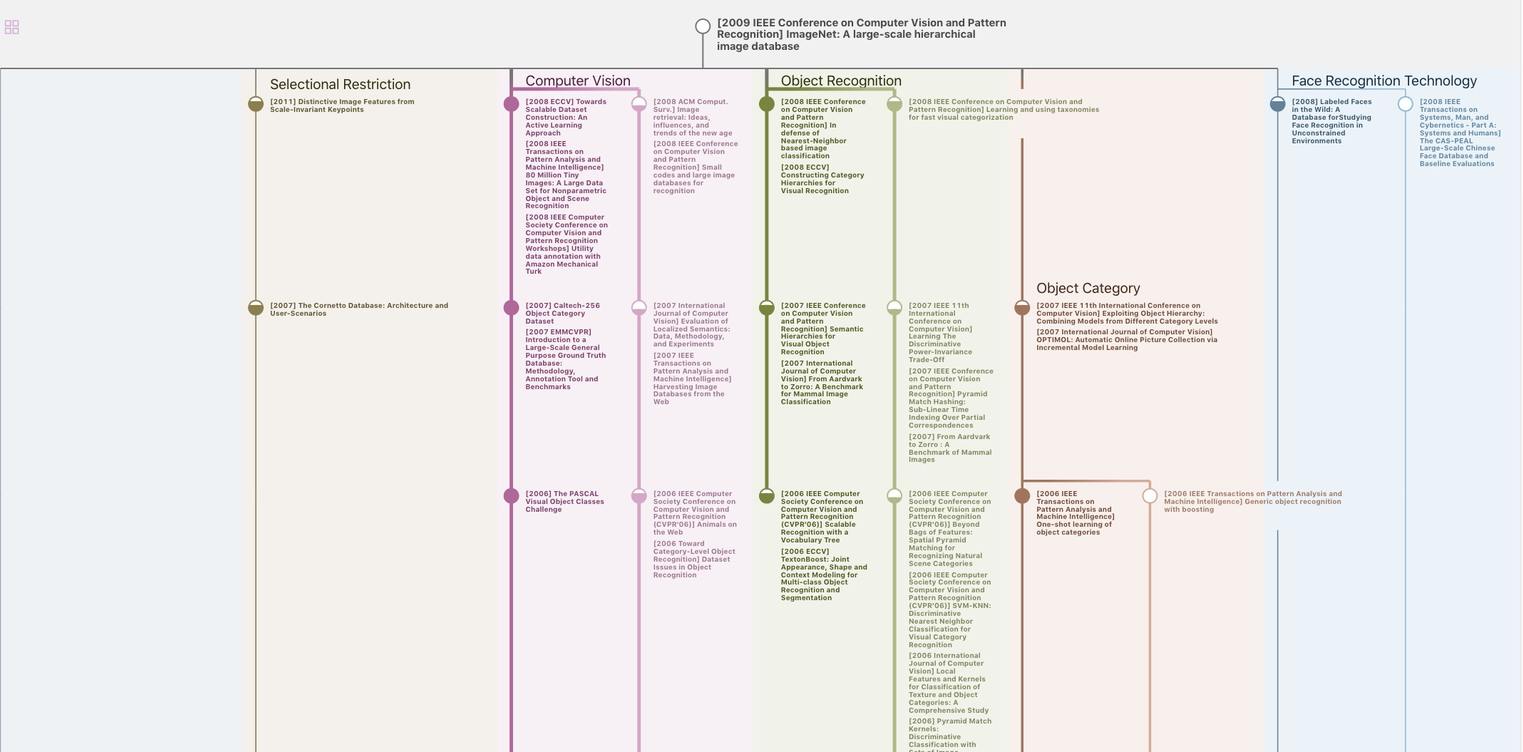
生成溯源树,研究论文发展脉络
Chat Paper
正在生成论文摘要