Generating airshower images for the VERITAS telescopes with conditional Generative Adversarial Networks
arXiv (Cornell University)(2023)
摘要
VERITAS (Very Energetic Radiation Imaging Telescope Array System) is the current-generation array comprising four 12-meter optical ground-based Imaging Atmospheric Cherenkov Telescopes (IACTs). Its primary goal is to indirectly observe gamma-ray emissions from the most violent astrophysical sources in the universe. Recent advancements in Machine Learning (ML) have sparked interest in utilizing neural networks (NNs) to directly infer properties from IACT images. However, the current training data for these NNs is generated through computationally expensive Monte Carlo (MC) simulation methods. This study presents a simulation method that employs conditional Generative Adversarial Networks (cGANs) to synthesize additional VERITAS data to facilitate training future NNs. In this test-of-concept study, we condition the GANs on five classes of simulated camera images consisting of circular muon showers and gamma-ray shower images in the first, second, third, and fourth quadrants of the camera. Our results demonstrate that by casting training data as time series, cGANs can 1) replicate shower morphologies based on the input class vectors and 2) generalize additional signals through interpolation in both the class and latent spaces. Leveraging GPUs strength, our method can synthesize novel signals at an impressive speed, generating over $10^6$ shower events in less than a minute.
更多查看译文
关键词
generative adversarial networks,airshower images,veritas telescopes
AI 理解论文
溯源树
样例
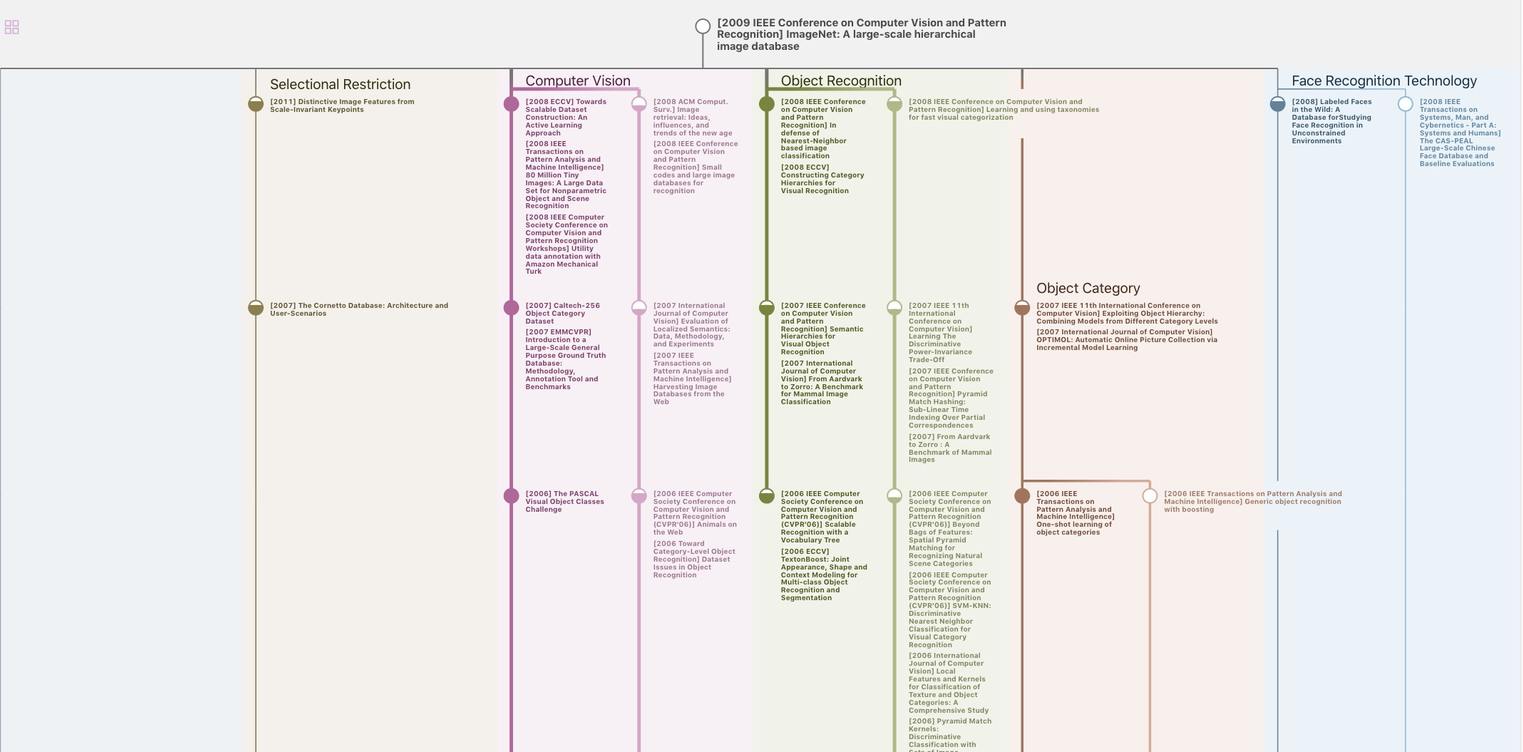
生成溯源树,研究论文发展脉络
Chat Paper
正在生成论文摘要