Internal Cross-layer Gradients for Extending Homogeneity to Heterogeneity in Federated Learning
arXiv (Cornell University)(2023)
摘要
Federated learning (FL) inevitably confronts the challenge of system
heterogeneity in practical scenarios. To enhance the capabilities of most
model-homogeneous FL methods in handling system heterogeneity, we propose a
training scheme that can extend their capabilities to cope with this challenge.
In this paper, we commence our study with a detailed exploration of homogeneous
and heterogeneous FL settings and discover three key observations: (1) a
positive correlation between client performance and layer similarities, (2)
higher similarities in the shallow layers in contrast to the deep layers, and
(3) the smoother gradients distributions indicate the higher layer
similarities. Building upon these observations, we propose InCo Aggregation
that leverages internal cross-layer gradients, a mixture of gradients from
shallow and deep layers within a server model, to augment the similarity in the
deep layers without requiring additional communication between clients.
Furthermore, our methods can be tailored to accommodate model-homogeneous FL
methods such as FedAvg, FedProx, FedNova, Scaffold, and MOON, to expand their
capabilities to handle the system heterogeneity. Copious experimental results
validate the effectiveness of InCo Aggregation, spotlighting internal
cross-layer gradients as a promising avenue to enhance the performance in
heterogeneous FL.
更多查看译文
关键词
gradients,heterogeneity,learning,internal,cross-layer
AI 理解论文
溯源树
样例
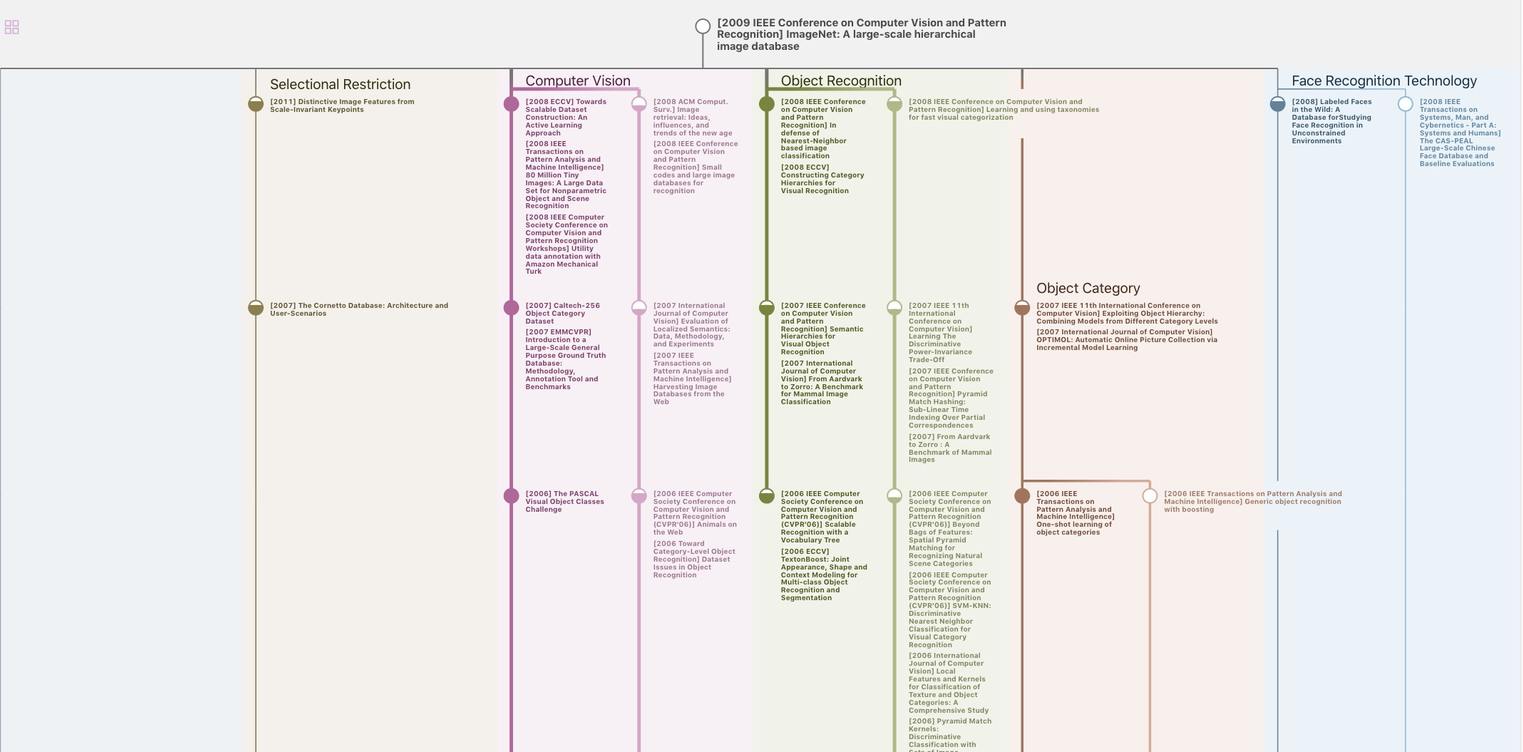
生成溯源树,研究论文发展脉络
Chat Paper
正在生成论文摘要