A LiDAR-Inertial SLAM Tightly Coupled With Dropout-Tolerant GNSS Fusion for Autonomous Mine Service Vehicles
CoRR(2023)
摘要
Multimodal sensor integration has become a crucial prerequisite for real-world navigation systems. Recent studies have reported successful deployment of such systems in many fields. However, it is still challenging for navigation tasks in mine scenes due to satellite signal dropouts, degraded perception, and observation degeneracy. To solve this problem, we propose a light detection and ranging (LiDAR)-inertial odometry method in this article, utilizing both Kalman filter and graph optimization. The front-end consists of multiple parallel running LiDAR-inertial odometries, where the laser points, IMU, and wheel odometer information are tightly fused in an error-state Kalman filter (ESKF). Instead of the commonly used feature points, we employ surface elements for registration. The back end constructs a pose graph and jointly optimizes the pose estimation results from inertial, LiDAR odometry, and global navigation satellite system (GNSS). Since the vehicle has a long operation time inside the tunnel, the largely accumulated drift may be not fully by the GNSS measurements. We hereby leverage a loop closure-based reinitialization process to achieve full alignment. In addition, the system robustness is improved through handling data loss, stream consistency, and estimation error. The experimental results show that our system has a good tolerance to the long-period degeneracy with the cooperation of different LiDARs and surfel registration, achieving meter-level accuracy even for tens of minutes running during GNSS dropouts.
更多查看译文
关键词
autonomous mine service vehicles,fusion,lidar-inertial,tightly-coupled,dropout-tolerant
AI 理解论文
溯源树
样例
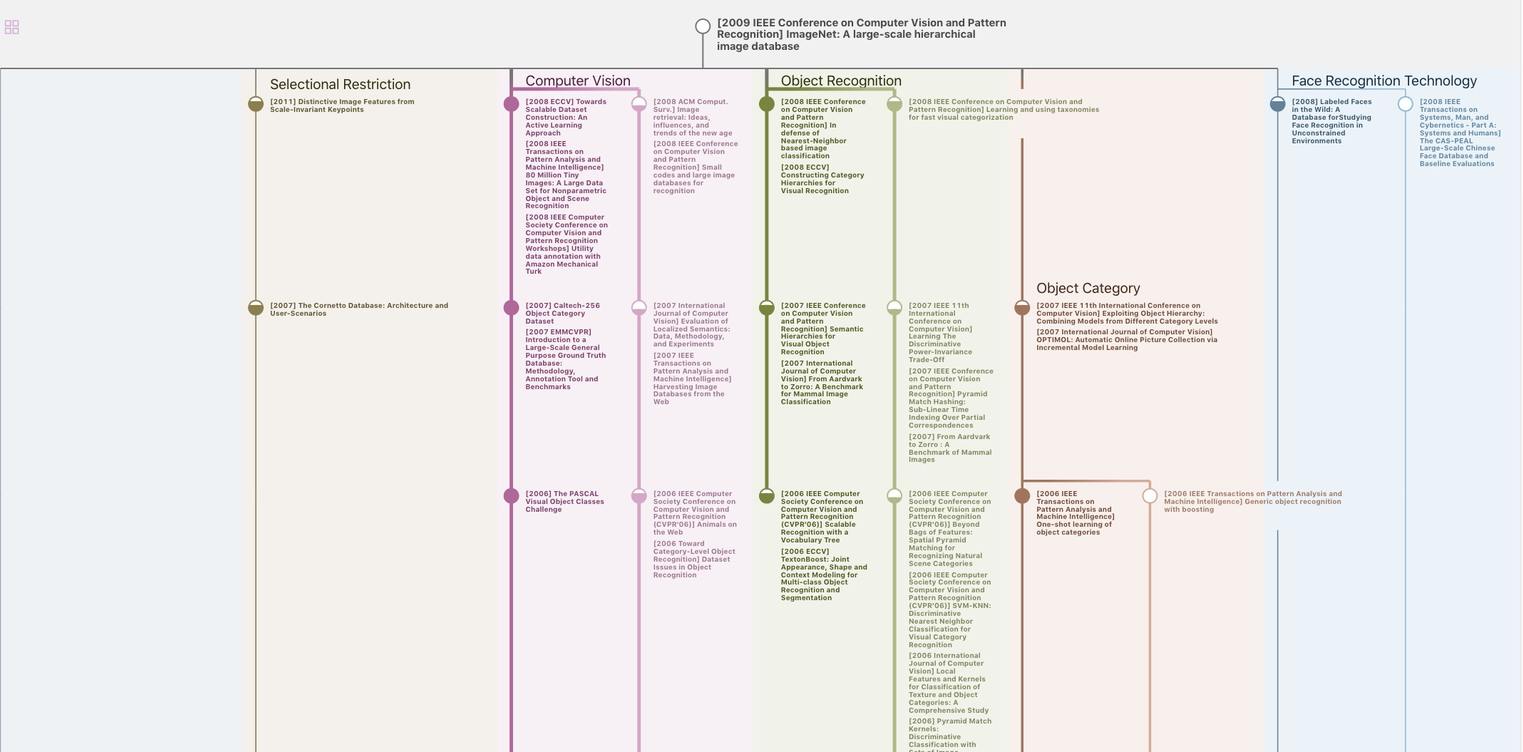
生成溯源树,研究论文发展脉络
Chat Paper
正在生成论文摘要