A CNN-LSTM Ensemble Model for Predicting Protein-Protein Interaction Binding Sites
IEEE-ACM TRANSACTIONS ON COMPUTATIONAL BIOLOGY AND BIOINFORMATICS(2023)
摘要
Proteins commonly perform biological functions through protein-protein interactions (PPIs). The knowledge of PPI sites is imperative for the understanding of protein functions, disease mechanisms, and drug design. Traditional biological experimental methods for studying PPI sites still incur considerable drawbacks, including long experimental time and high labor costs. Therefore, many computational methods have been proposed for predicting PPI sites. However, achieving high prediction performance and overcoming severe data imbalance remain challenging issues. In this paper, we propose a new sequence-based deep learning model called CLPPIS (standing for C NN-L STM ensemble based PPI S ites prediction). CLPPIS consists of CNN and LSTM components, which can capture spatial features and sequential features simultaneously. Further, it utilizes a novel feature group as input, which has 7 physicochemical, biophysical, and statistical properties. Besides, it adopts a batch-weighted loss function to reduce the interference of imbalance data. Our work suggests that the integration of protein spatial features and sequential features provides important information for PPI sites prediction. Evaluation on three public benchmark datasets shows that our CLPPIS model significantly outperforms existing state-of-the-art methods.
更多查看译文
关键词
Ensemble model,feature extraction,PPI sites prediction,protein sequence
AI 理解论文
溯源树
样例
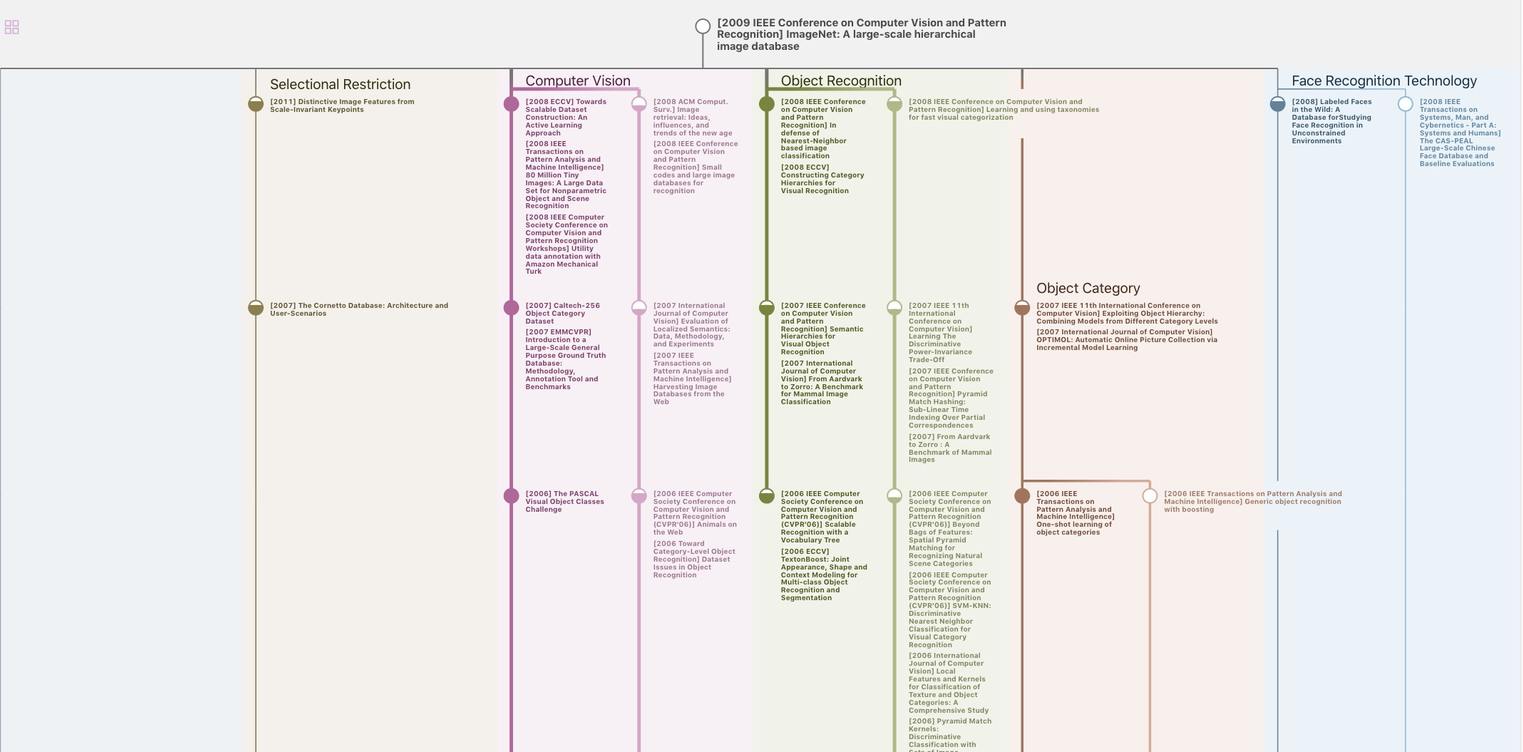
生成溯源树,研究论文发展脉络
Chat Paper
正在生成论文摘要