Robust deep learning-based PET prognostic imaging biomarker for DLBCL patients: a multicenter study
European journal of nuclear medicine and molecular imaging(2023)
摘要
Objective To develop and independently externally validate robust prognostic imaging biomarkers distilled from PET images using deep learning techniques for precise survival prediction in patients with diffuse large B cell lymphoma (DLBCL). Methods A total of 684 DLBCL patients from three independent medical centers were included in this retrospective study. Deep learning scores (DLS) were generated from PET images using deep convolutional neural network architecture known as VGG19 and DenseNet121. These DLSs were utilized to predict progression-free survival (PFS) and overall survival (OS). Furthermore, multiparametric models were designed based on results from the Cox proportional hazards model and assessed through calibration curves, concordance index (C-index), and decision curve analysis (DCA) in the training and validation cohorts. Results The DLS PFS and DLS OS exhibited significant associations with PFS and OS, respectively (P<0.05) in the training and validation cohorts. The multiparametric models that incorporated DLSs demonstrated superior efficacy in predicting PFS (C-index: 0.866) and OS (C-index: 0.835) compared to competing models in training cohorts. In external validation cohorts, the C-indices for PFS and OS were 0.760 and. 0.770 and 0.748 and 0.766, respectively, indicating the reliable validity of the multiparametric models. The calibration curves displayed good consistency, and the decision curve analysis (DCA) confirmed that the multiparametric models offered more net clinical benefits. Conclusions The DLSs were identified as robust prognostic imaging biomarkers for survival in DLBCL patients. Moreover, the multiparametric models developed in this study exhibited promising potential in accurately stratifying patients based on their survival risk.
更多查看译文
关键词
pet prognostic imaging biomarker,dlbcl patients,learning-based
AI 理解论文
溯源树
样例
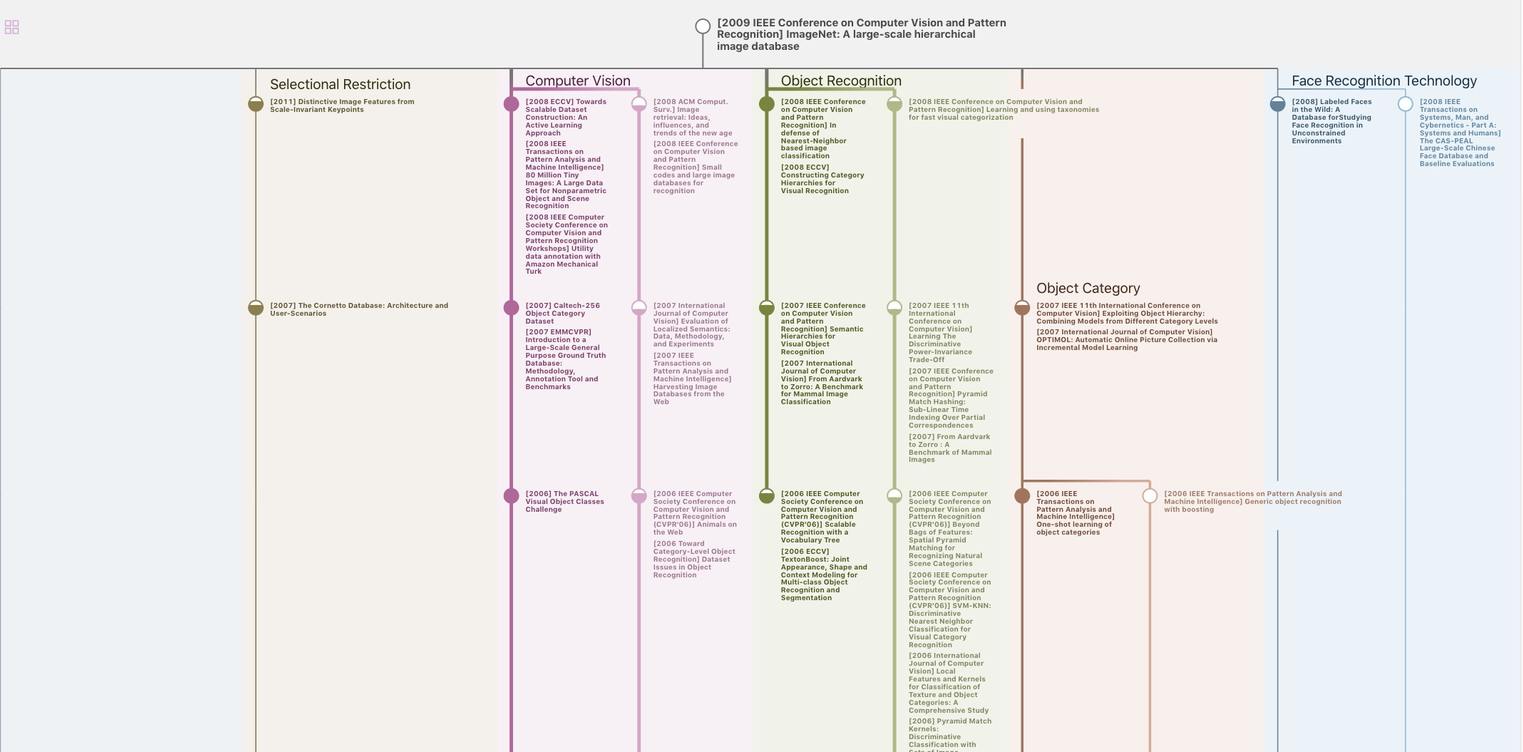
生成溯源树,研究论文发展脉络
Chat Paper
正在生成论文摘要