Neural network interatomic potential for laser-excited materials
Communications Materials(2023)
摘要
Data-driven interatomic potentials based on machine-learning approaches have been increasingly used to perform large-scale, first-principles quality simulations of materials in the electronic ground state. However, they are not able to describe situations in which the electrons are excited, like in the case of material processing by means of femtosecond laser irradiation or ion bombardment. In this work, we propose a neural network interatomic potential with an explicit dependency on the electronic temperature. Taking silicon as an example, we demonstrate its capability of reproducing important physical properties with first-principles accuracy and use it to simulate laser-induced surface modifications on a thin film at time and length scales that are impossible to reach with first-principles approaches like density functional theory. The method is general and can be applied not only to other laser-excited materials but also to condensed and liquid matter under non-equilibrium situations in which electrons and ions exhibit different temperatures.
更多查看译文
关键词
neural network,interatomic potential,materials,laser-excited
AI 理解论文
溯源树
样例
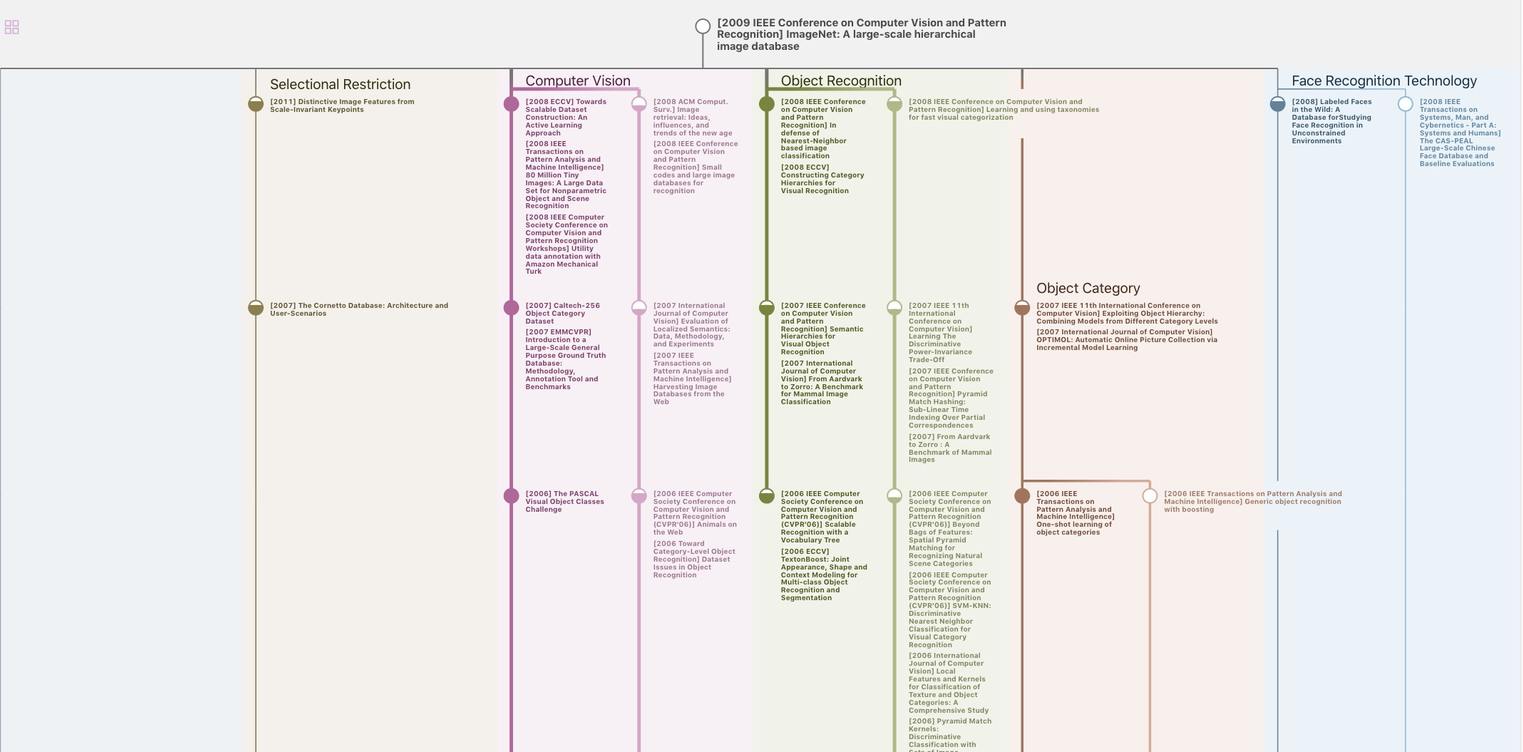
生成溯源树,研究论文发展脉络
Chat Paper
正在生成论文摘要