Predicting Sandstone Brittleness under Varying Water Conditions Using Infrared Radiation and Computational Techniques
WATER(2024)
摘要
The brittleness index is one of the most integral parameters used in assessing rock bursts and catastrophic rock failures resulting from deep underground mining activities. Accurately predicting this parameter is crucial for effectively monitoring rock bursts, which can cause damage to miners and lead to the catastrophic failure of engineering structures. Therefore, developing a new brittleness index capable of effectively predicting rock bursts is essential for the safe and efficient execution of engineering projects. In this research study, a novel mathematical rock brittleness index is developed, utilizing factors such as crack initiation, crack damage, and peak stress for sandstones with varying water contents. Additionally, the brittleness index is compared with previous important brittleness indices (e.g., B-1, B-2, B-3, and B-4) predicted using infrared radiation (IR) characteristics, specifically the variance of infrared radiation temperature (VIRT), along with various artificial intelligent (AI) techniques such as k-nearest neighbor (KNN), extreme gradient boost (XGBoost), and random forest (RF), providing comprehensive insights for predicting rock bursts. The experimental and AI results revealed that: (1) crack initiation, elastic modulus, crack damage, and peak stress decrease with an increase in water content; (2) the brittleness indices such as B-1, B-3, and B-4 show a positive linear exponential correlation, having a coefficient of determination of R-2 = 0.88, while B-2 shows a negative linear exponential correlation (R-2 = 0.82) with water content. Furthermore, the proposed brittleness index shows a good linear correlation with B-1, B-3, and B-4, with an R-2 > 0.85, while it shows a poor negative linear correlation with B-2, with an R-2 = 0.61; (3) the RF model, developed for predicting the brittleness index, demonstrates superior performance when compared to other models, as indicated by the following performance parameters: R-2 = 0.999, root mean square error (RMSE) = 0.383, mean square error (MSE) = 0.007, and mean absolute error (MAE) = 0.002. Consequently, RF stands as being recommended for accurate rock brittleness prediction. These research findings offer valuable insights and guidelines for effectively developing a brittleness index to assess the rock burst risks associated with rock engineering projects under water conditions.
更多查看译文
关键词
rock brittleness,water contents,rock burst,infrared radiation characteristics and artificial intelligent techniques
AI 理解论文
溯源树
样例
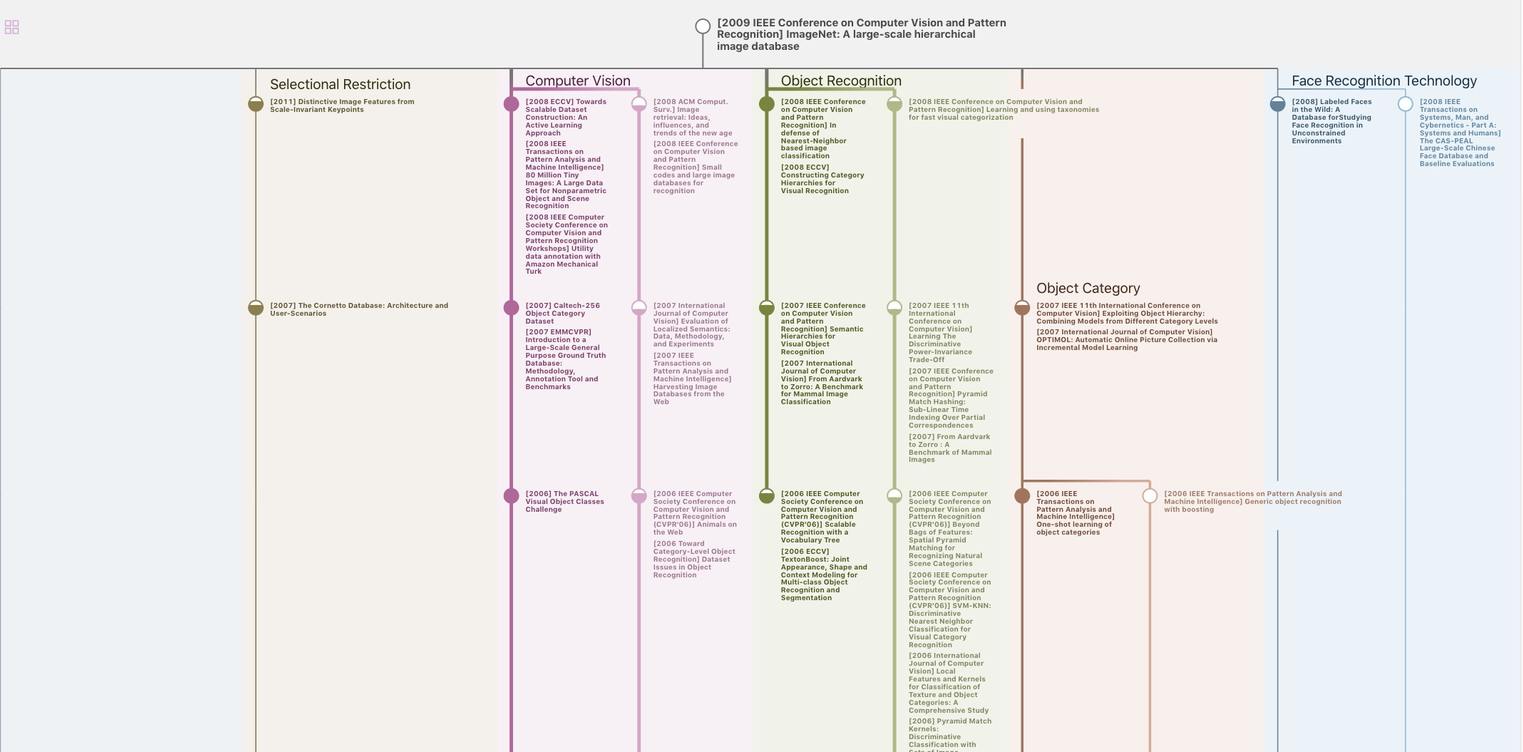
生成溯源树,研究论文发展脉络
Chat Paper
正在生成论文摘要