ACLS: Adaptive and Conditional Label Smoothing for Network Calibration
CoRR(2023)
摘要
We address the problem of network calibration adjusting miscalibrated confidences of deep neural networks. Many approaches to network calibration adopt a regularization-based method that exploits a regularization term to smooth the miscalibrated confidences. Although these approaches have shown the effectiveness on calibrating the networks, there is still a lack of understanding on the underlying principles of regularization in terms of network calibration. We present in this paper an in-depth analysis of existing regularization-based methods, providing a better understanding on how they affect to network calibration. Specifically, we have observed that 1) the regularization-based methods can be interpreted as variants of label smoothing, and 2) they do not always behave desirably. Based on the analysis, we introduce a novel loss function, dubbed ACLS, that unifies the merits of existing regularization methods, while avoiding the limitations. We show extensive experimental results for image classification and semantic segmentation on standard benchmarks, including CIFAR10, Tiny-ImageNet, ImageNet, and PASCAL VOC, demonstrating the effectiveness of our loss function.
更多查看译文
关键词
conditional label smoothing,calibration
AI 理解论文
溯源树
样例
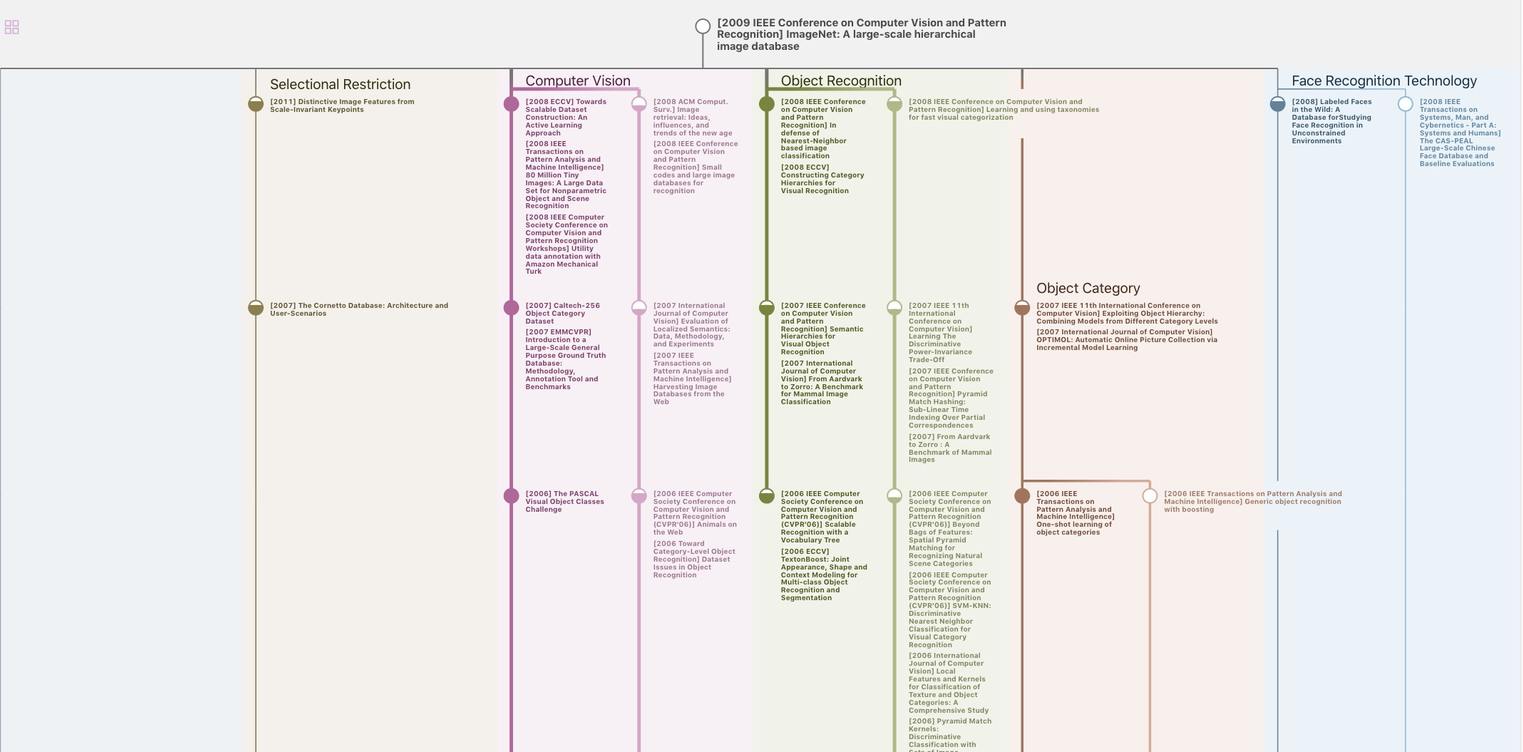
生成溯源树,研究论文发展脉络
Chat Paper
正在生成论文摘要