Anisotropic Hybrid Networks for liver tumor segmentation with uncertainty quantification
CoRR(2023)
摘要
The burden of liver tumors is important, ranking as the fourth leading cause of cancer mortality. In case of hepatocellular carcinoma (HCC), the delineation of liver and tumor on contrast-enhanced magnetic resonance imaging (CE-MRI) is performed to guide the treatment strategy. As this task is time-consuming, needs high expertise and could be subject to inter-observer variability there is a strong need for automatic tools. However, challenges arise from the lack of available training data, as well as the high variability in terms of image resolution and MRI sequence. In this work we propose to compare two different pipelines based on anisotropic models to obtain the segmentation of the liver and tumors. The first pipeline corresponds to a baseline multi-class model that performs the simultaneous segmentation of the liver and tumor classes. In the second approach, we train two distinct binary models, one segmenting the liver only and the other the tumors. Our results show that both pipelines exhibit different strengths and weaknesses. Moreover we propose an uncertainty quantification strategy allowing the identification of potential false positive tumor lesions. Both solutions were submitted to the MICCAI 2023 Atlas challenge regarding liver and tumor segmentation.
更多查看译文
关键词
liver tumor segmentation,liver tumor,networks,uncertainty quantification
AI 理解论文
溯源树
样例
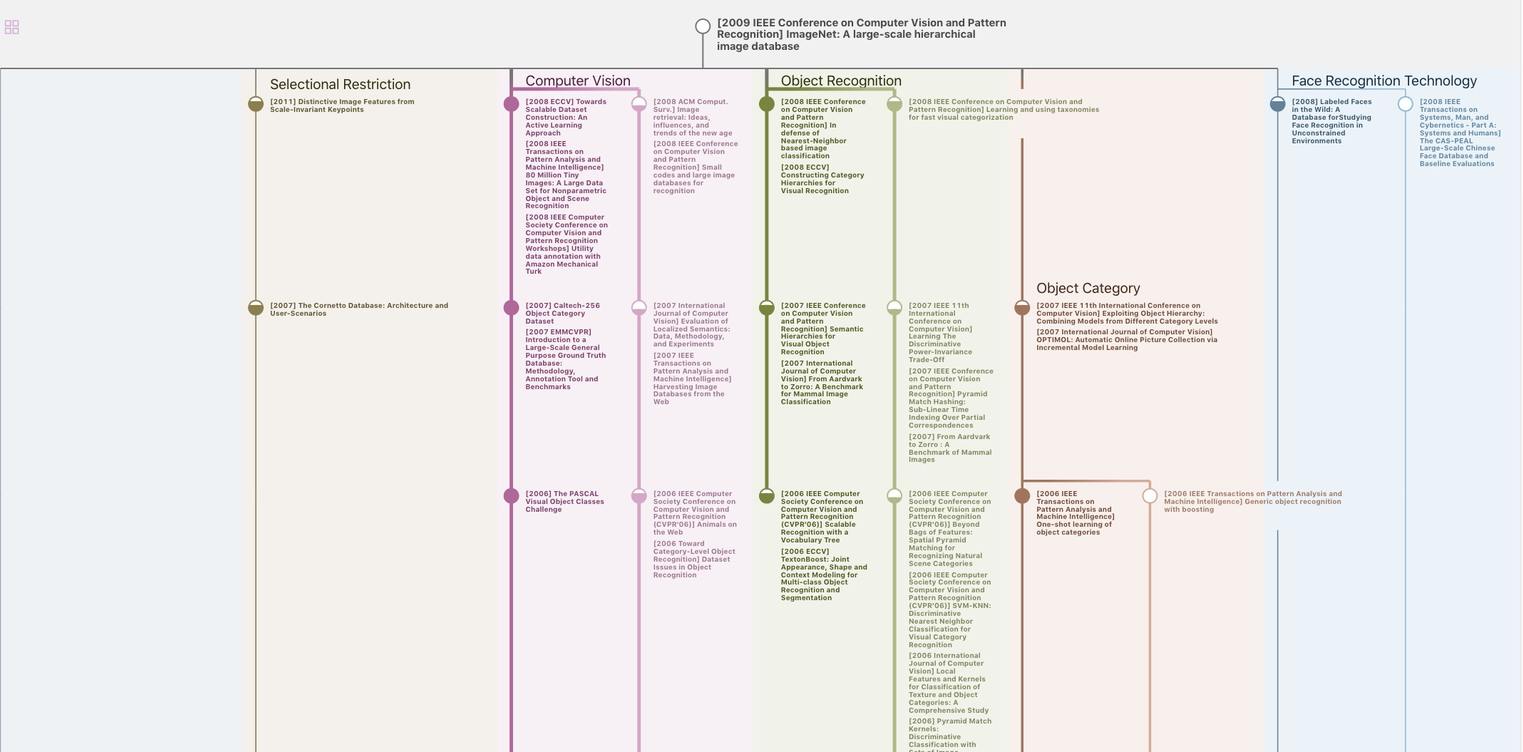
生成溯源树,研究论文发展脉络
Chat Paper
正在生成论文摘要