Quantifying the Uncertainty of Human Activity Recognition Using a Bayesian Machine Learning Method: A Prediction Study
medrxiv(2023)
摘要
Background Machine learning methods accurately predict physical activity outcomes using accelerometer data generated by wearable devices, thus allowing the investigation of the impact of built environment on population physical activity. While traditional machine learning methods do not provide prediction uncertainty, a new method, Bayesian Additive Regression Trees (BART) can quantify such uncertainty as posterior predictive distribution. We evaluated the performance of BART in predicting physical activity status.
Methods We applied multinomial BART and the benchmark method, random forest, to accelerometer data in 25,424 time points, which were generated by wearable devices attached to 37 participants. We evaluated prediction accuracies and confusion matrix using leave-one-out cross-validation.
Results BART and random forest demonstrated comparable accuracies in prediction.
Conclusions BART is a relatively novel ML method and will advance the incorporation of predicted physical activity status into built environment research. Future research includes the evaluation of the association between the built environment and predicted physical activity with and without accounting for prediction uncertainty.
### Competing Interest Statement
The authors have declared no competing interest.
### Funding Statement
The research was supported by the Postdoctoral fellowship from the Artificial Intelligence for Public Health (AI4PH) training platform, Canadian Institute for Health Research. The founder had no role in the design of the study and collection, analysis, and interpretation of data and in writing the manuscript.
### Author Declarations
I confirm all relevant ethical guidelines have been followed, and any necessary IRB and/or ethics committee approvals have been obtained.
Yes
The details of the IRB/oversight body that provided approval or exemption for the research described are given below:
This study was approved by the Memorial University Interdisciplinary Committee on Ethics in Human Research (ICEHR 20180188-EX).
I confirm that all necessary patient/participant consent has been obtained and the appropriate institutional forms have been archived, and that any patient/participant/sample identifiers included were not known to anyone (e.g., hospital staff, patients or participants themselves) outside the research group so cannot be used to identify individuals.
Yes
I understand that all clinical trials and any other prospective interventional studies must be registered with an ICMJE-approved registry, such as ClinicalTrials.gov. I confirm that any such study reported in the manuscript has been registered and the trial registration ID is provided (note: if posting a prospective study registered retrospectively, please provide a statement in the trial ID field explaining why the study was not registered in advance).
Yes
I have followed all appropriate research reporting guidelines, such as any relevant EQUATOR Network research reporting checklist(s) and other pertinent material, if applicable.
Yes
The de-identified accelerometer data are available publicly at: .
* BART
: Bayesian Additive Regression Trees
CI
: Credible Interval
ML
: Machine Learning
PA
: Physical Activity
更多查看译文
AI 理解论文
溯源树
样例
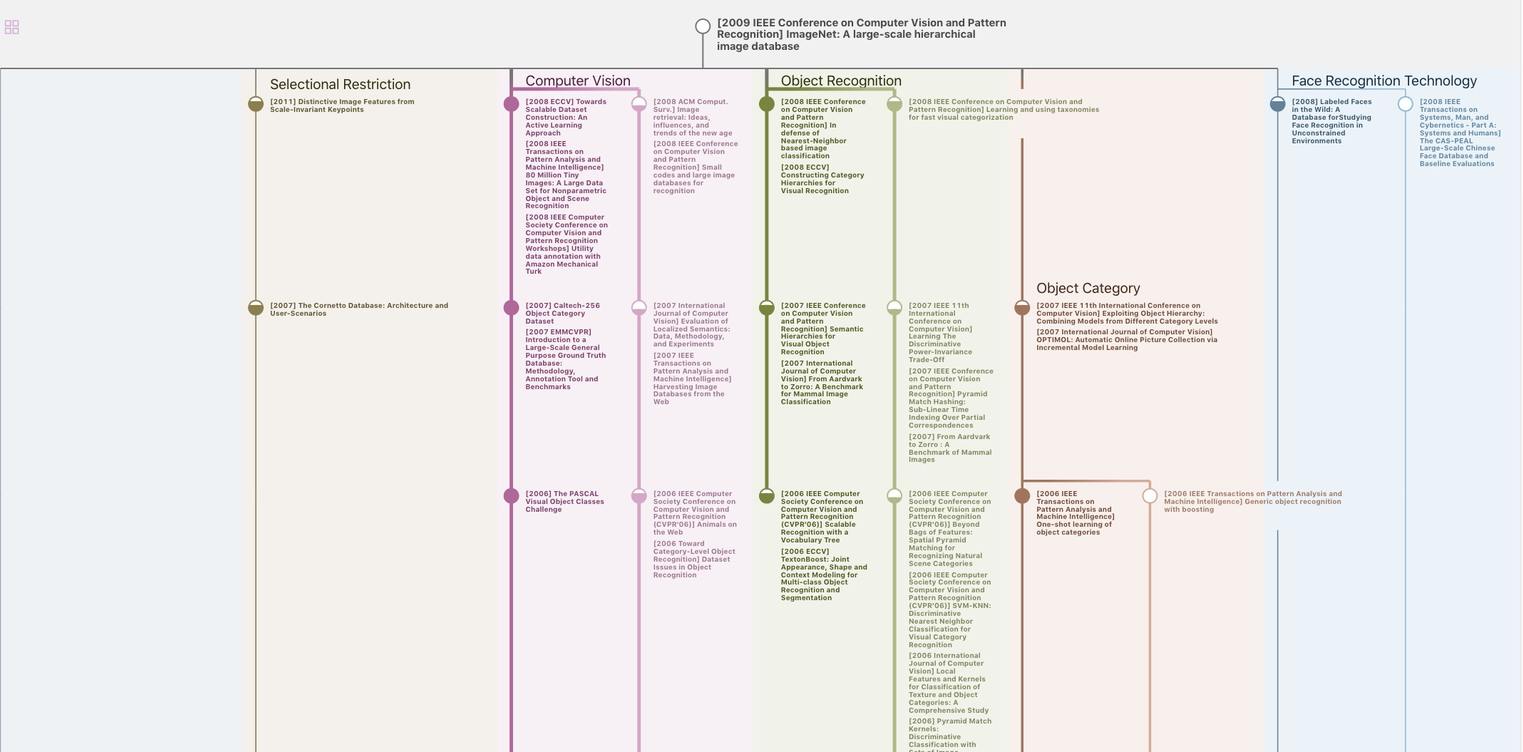
生成溯源树,研究论文发展脉络
Chat Paper
正在生成论文摘要