Phenotype-based targeted treatment of SGLT2 inhibitors and GLP-1 receptor agonists in type 2 diabetes
Diabetologia(2024)
摘要
A precision medicine approach in type 2 diabetes could enhance targeting specific glucose-lowering therapies to individual patients most likely to benefit. We aimed to use the recently developed Bayesian causal forest (BCF) method to develop and validate an individualised treatment selection algorithm for two major type 2 diabetes drug classes, sodium–glucose cotransporter 2 inhibitors (SGLT2i) and glucagon-like peptide-1 receptor agonists (GLP1-RA). We designed a predictive algorithm using BCF to estimate individual-level conditional average treatment effects for 12-month glycaemic outcome (HbA1c) between SGLT2i and GLP1-RA, based on routine clinical features of 46,394 people with type 2 diabetes in primary care in England (Clinical Practice Research Datalink; 27,319 for model development, 19,075 for hold-out validation), with additional external validation in 2252 people with type 2 diabetes from Scotland (SCI-Diabetes [Tayside Fife]). Differences in glycaemic outcome with GLP1-RA by sex seen in clinical data were replicated in clinical trial data (HARMONY programme: liraglutide [n=389] and albiglutide [n=1682]). As secondary outcomes, we evaluated the impacts of targeting therapy based on glycaemic response on weight change, tolerability and longer-term risk of new-onset microvascular complications, macrovascular complications and adverse kidney events. Model development identified marked heterogeneity in glycaemic response, with 4787 (17.5
更多查看译文
关键词
Bayesian non-parametric modelling,GLP1-receptor agonists,Heterogeneous treatment effects,Precision medicine,SGLT2-inhibitors,Type 2 diabetes
AI 理解论文
溯源树
样例
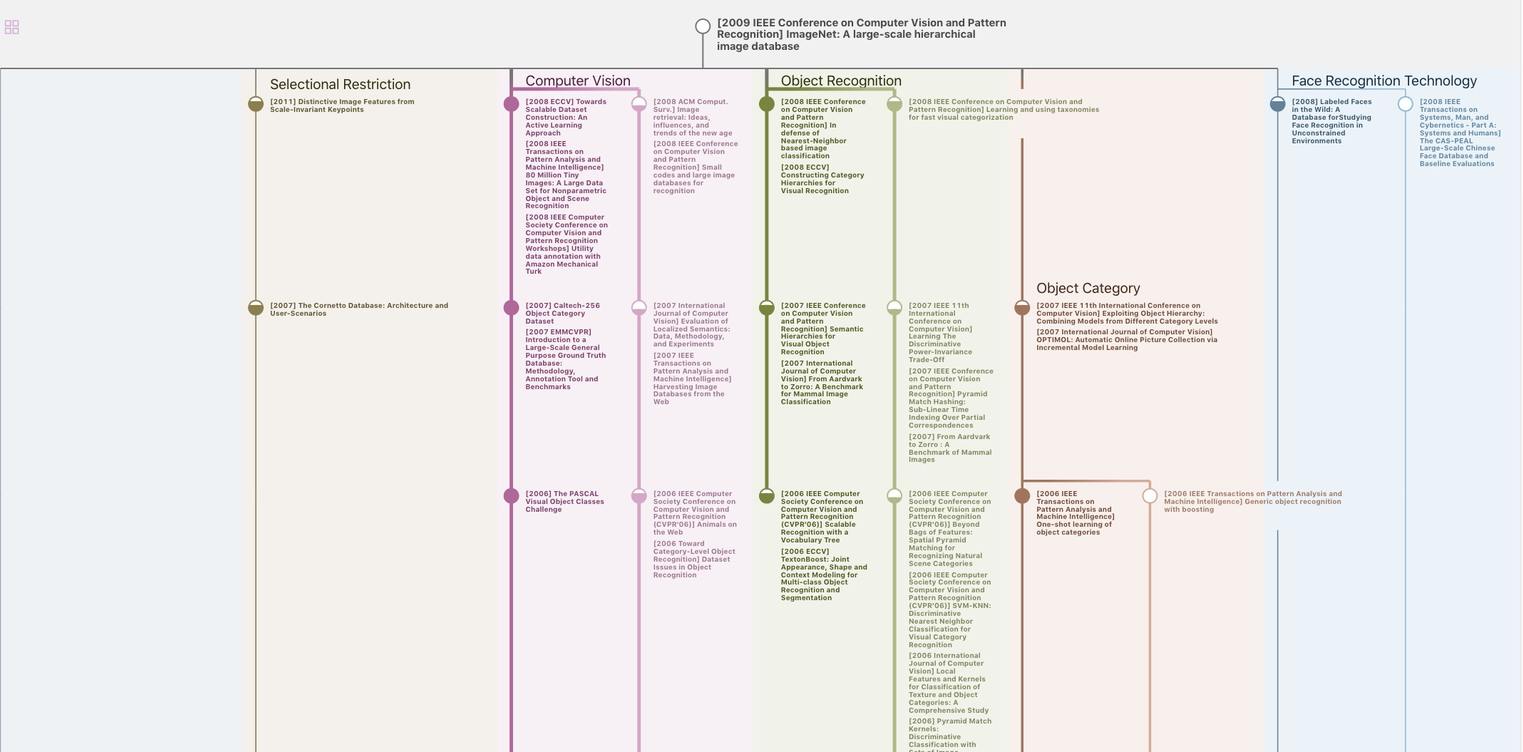
生成溯源树,研究论文发展脉络
Chat Paper
正在生成论文摘要