Robustness and Sensitivity of Artificial Neural Networks for Mechatronic Product Development
AmE 2023 – Automotive meets Electronics; 14. GMM Symposium(2023)
摘要
In the fast-paced world of automotive development, the need for effective information exchange between different domains is becoming increasingly critical. The parallelized and agile nature of development demands early and frequent access to accurate information across various departments. However, deviations and uncertainties in data can lead to costly disruptions in the development process. Artificial intelligence based prediction models offer a potential solution by providing estimates based on previous projects or analyzed products, even in the early stages of development. While rough estimates may suffice initially, it is important to understand the accuracy of such predictions. This study therefore aims to evaluate the performance characteristics of different uncertainty analysis methods and assess their applicability in agile automotive development processes. By considering the specific requirements and constraints of each method, a decision tree is proposed to recommend suitable and situation-appropriate methods for performing uncertainty analyses in network prediction. The goal is to enhance data exchange between departments, mitigate disruptions, and ensure informed decision-making throughout the development process.
更多查看译文
AI 理解论文
溯源树
样例
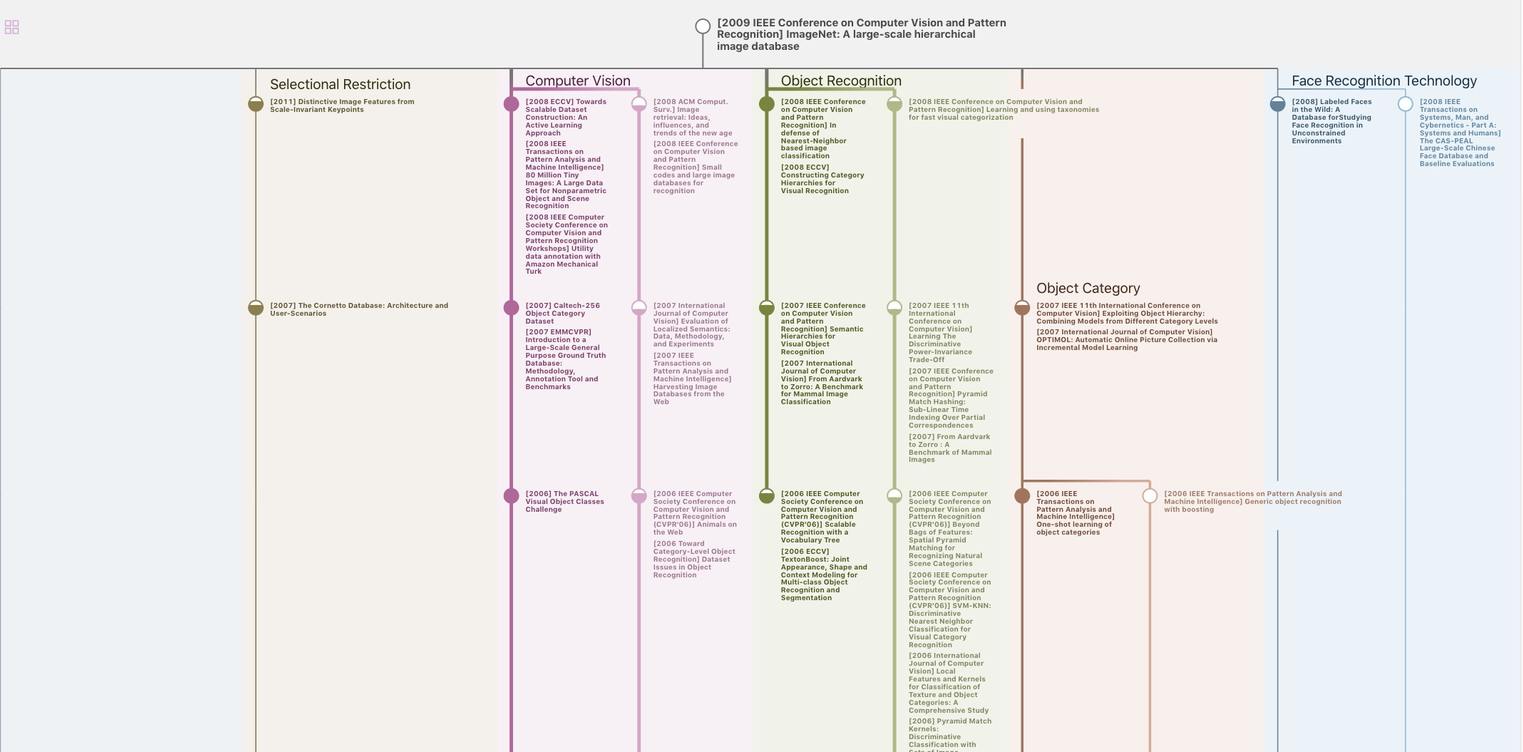
生成溯源树,研究论文发展脉络
Chat Paper
正在生成论文摘要