APART: Diverse Skill Discovery using All Pairs with Ascending Reward and DropouT
CoRR(2023)
摘要
We study diverse skill discovery in reward-free environments, aiming to discover all possible skills in simple grid-world environments where prior methods have struggled to succeed. This problem is formulated as mutual training of skills using an intrinsic reward and a discriminator trained to predict a skill given its trajectory. Our initial solution replaces the standard one-vs-all (softmax) discriminator with a one-vs-one (all pairs) discriminator and combines it with a novel intrinsic reward function and a dropout regularization technique. The combined approach is named APART: Diverse Skill Discovery using All Pairs with Ascending Reward and Dropout. We demonstrate that APART discovers all the possible skills in grid worlds with remarkably fewer samples than previous works. Motivated by the empirical success of APART, we further investigate an even simpler algorithm that achieves maximum skills by altering VIC, rescaling its intrinsic reward, and tuning the temperature of its softmax discriminator. We believe our findings shed light on the crucial factors underlying success of skill discovery algorithms in reinforcement learning.
更多查看译文
关键词
diverse skill discovery,ascending reward,dropout,pairs
AI 理解论文
溯源树
样例
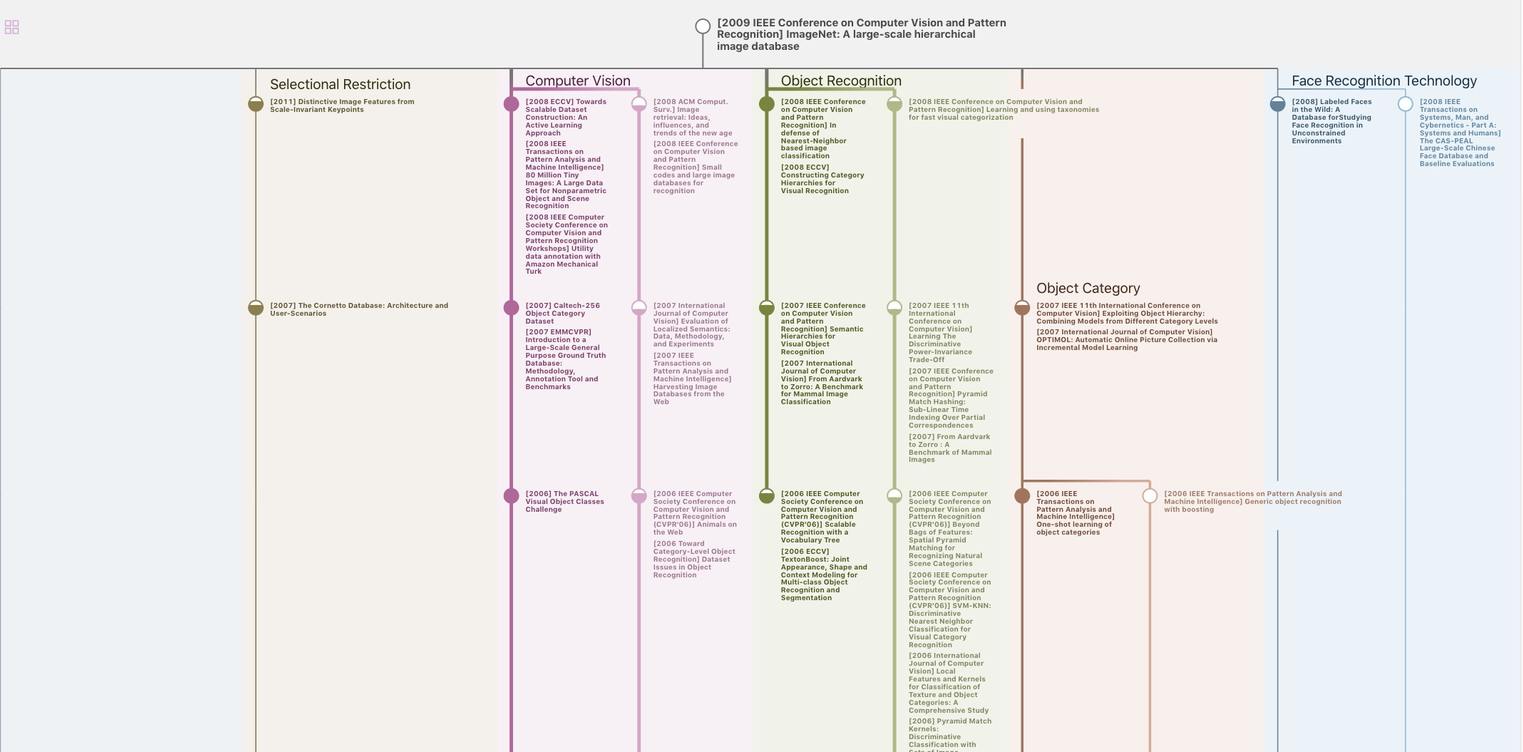
生成溯源树,研究论文发展脉络
Chat Paper
正在生成论文摘要