Solving Forward and Inverse Problems of Contact Mechanics Using Physics-Informed Neural Networks
Advanced modeling and simulation in engineering sciences(2024)
摘要
This paper explores the ability of physics-informed neural networks (PINNs)to solve forward and inverse problems of contact mechanics for smalldeformation elasticity. We deploy PINNs in a mixed-variable formulationenhanced by output transformation to enforce Dirichlet and Neumann boundaryconditions as hard constraints. Inequality constraints of contact problems,namely Karush-Kuhn-Tucker (KKT) type conditions, are enforced as softconstraints by incorporating them into the loss function during networktraining. To formulate the loss function contribution of KKT constraints,existing approaches applied to elastoplasticity problems are investigated andwe explore a nonlinear complementarity problem (NCP) function, namelyFischer-Burmeister, which possesses advantageous characteristics in terms ofoptimization. Based on the Hertzian contact problem, we show that PINNs canserve as pure partial differential equation (PDE) solver, as data-enhancedforward model, as inverse solver for parameter identification, and asfast-to-evaluate surrogate model. Furthermore, we demonstrate the importance ofchoosing proper hyperparameters, e.g. loss weights, and a combination of Adamand L-BFGS-B optimizers aiming for better results in terms of accuracy andtraining time.
更多查看译文
关键词
Physics-informed neural networks,Mixed-variable formulation,Contact mechanics,Enforcing inequalities,Fischer-Burmeister NCP-function
AI 理解论文
溯源树
样例
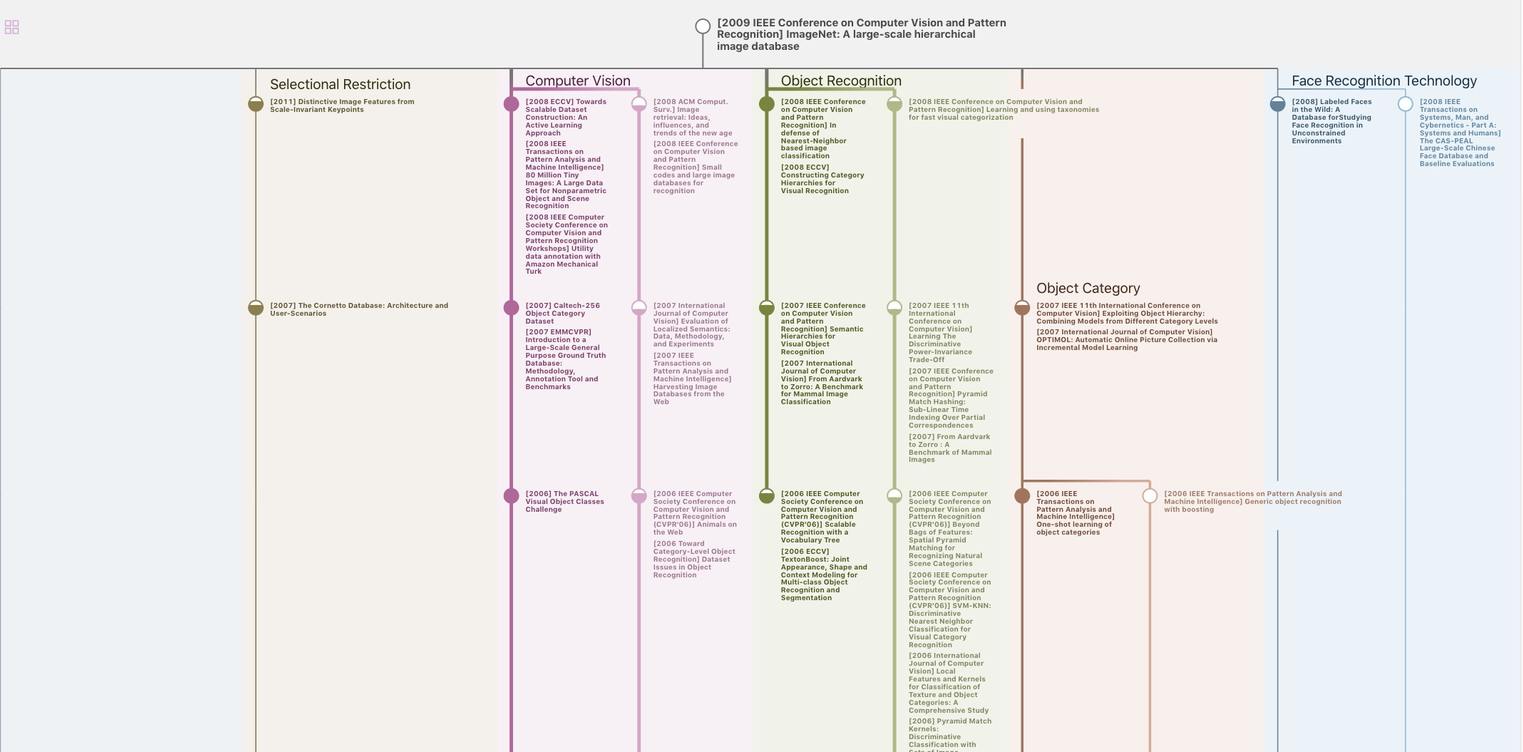
生成溯源树,研究论文发展脉络
Chat Paper
正在生成论文摘要