Jet Energy Calibration with Deep Learning as a Kubeflow Pipeline
Comput. Softw. Big Sci.(2023)
摘要
Precise measurements of the energy of jets emerging from particle collisions at the LHC are essential for a vast majority of physics searches at the CMS experiment. In this study, we leverage well-established deep learning models for point clouds and CMS open data to improve the energy calibration of particle jets. To enable production-ready machine learning based jet energy calibration an end-to-end pipeline is built on the Kubeflow cloud platform. The pipeline allowed us to scale up our hyperparameter tuning experiments on cloud resources, and serve optimal models as REST endpoints. We present the results of the parameter tuning process and analyze the performance of the served models in terms of inference time and overhead, providing insights for future work in this direction. The study also demonstrates improvements in both flavor dependence and resolution of the energy response when compared to the standard jet energy corrections baseline.
更多查看译文
关键词
kubeflow pipeline,jet,deep learning,calibration,energy
AI 理解论文
溯源树
样例
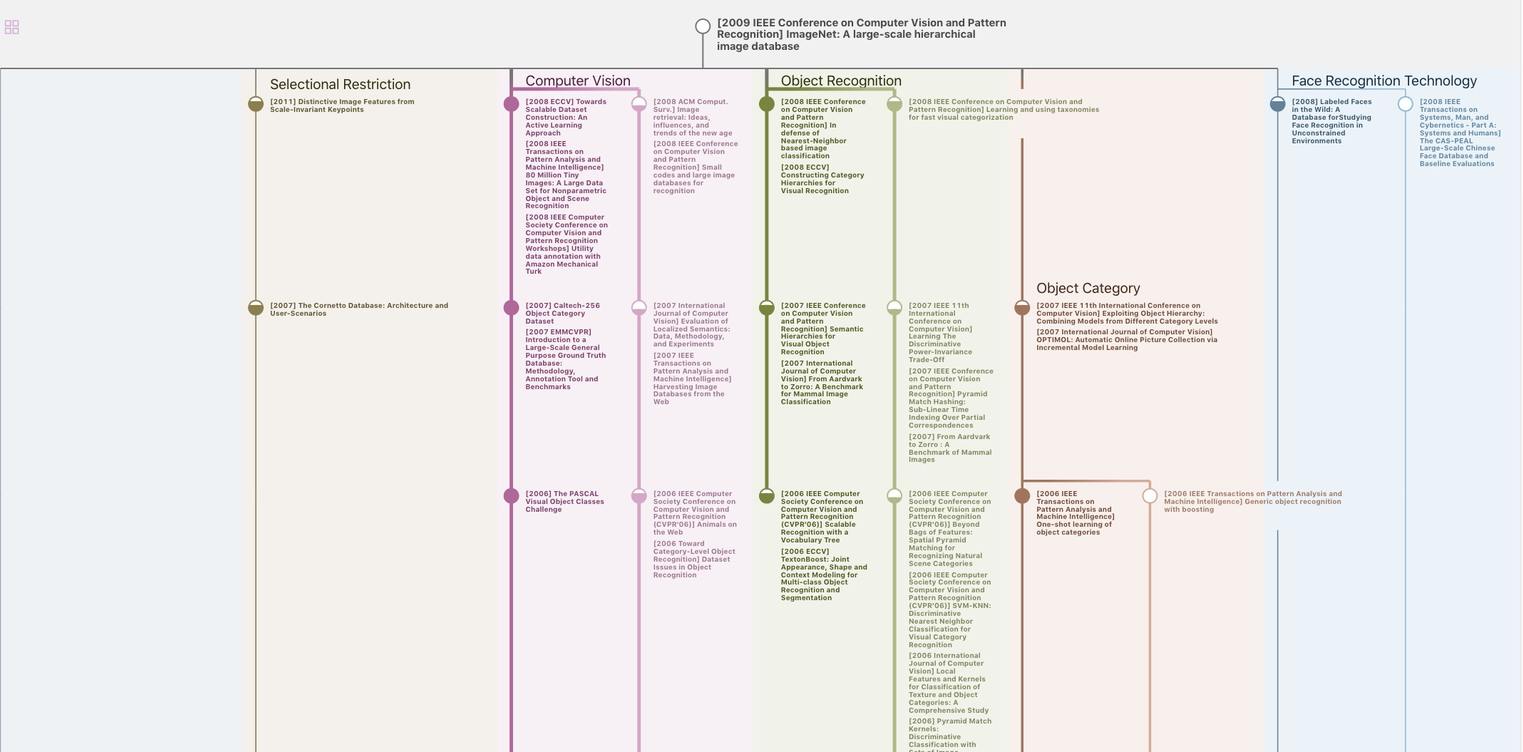
生成溯源树,研究论文发展脉络
Chat Paper
正在生成论文摘要