Incremental Learning for Simultaneous Augmentation of Feature and Class
IEEE TRANSACTIONS ON PATTERN ANALYSIS AND MACHINE INTELLIGENCE(2023)
摘要
With the emergence of new data collection ways in many dynamic environment applications, the samples are gathered gradually in the accumulated feature spaces. With the incorporation of new type features, it may result in the augmentation of class numbers. For instance, in activity recognition, using the old features during warm-up, we can separate different warm-up exercises. With the accumulation of new attributes obtained from newly added sensors, we can better separate the newly appeared formal exercises. Learning for such simultaneous augmentation of feature and class is crucial but rarely studied, particularly when the labeled samples with full observations are limited. In this paper, we tackle this problem by proposing a novel incremental learning method for Simultaneous Augmentation of Feature and Class (SAFC) in a two-stage way. To guarantee the reusability of the model trained on previous data, we add a regularizer in the current model, which can provide solid prior in training the new classifier. We also present the theoretical analyses about the generalization bound, which can validate the efficiency of model inheritance. After solving the one-shot problem, we also extend it to multi-shot. Experimental results demonstrate the effectiveness of our approaches, together with their effectiveness in activity recognition applications.
更多查看译文
关键词
Incremental learning,simultaneous feature and class augmentation,reusability
AI 理解论文
溯源树
样例
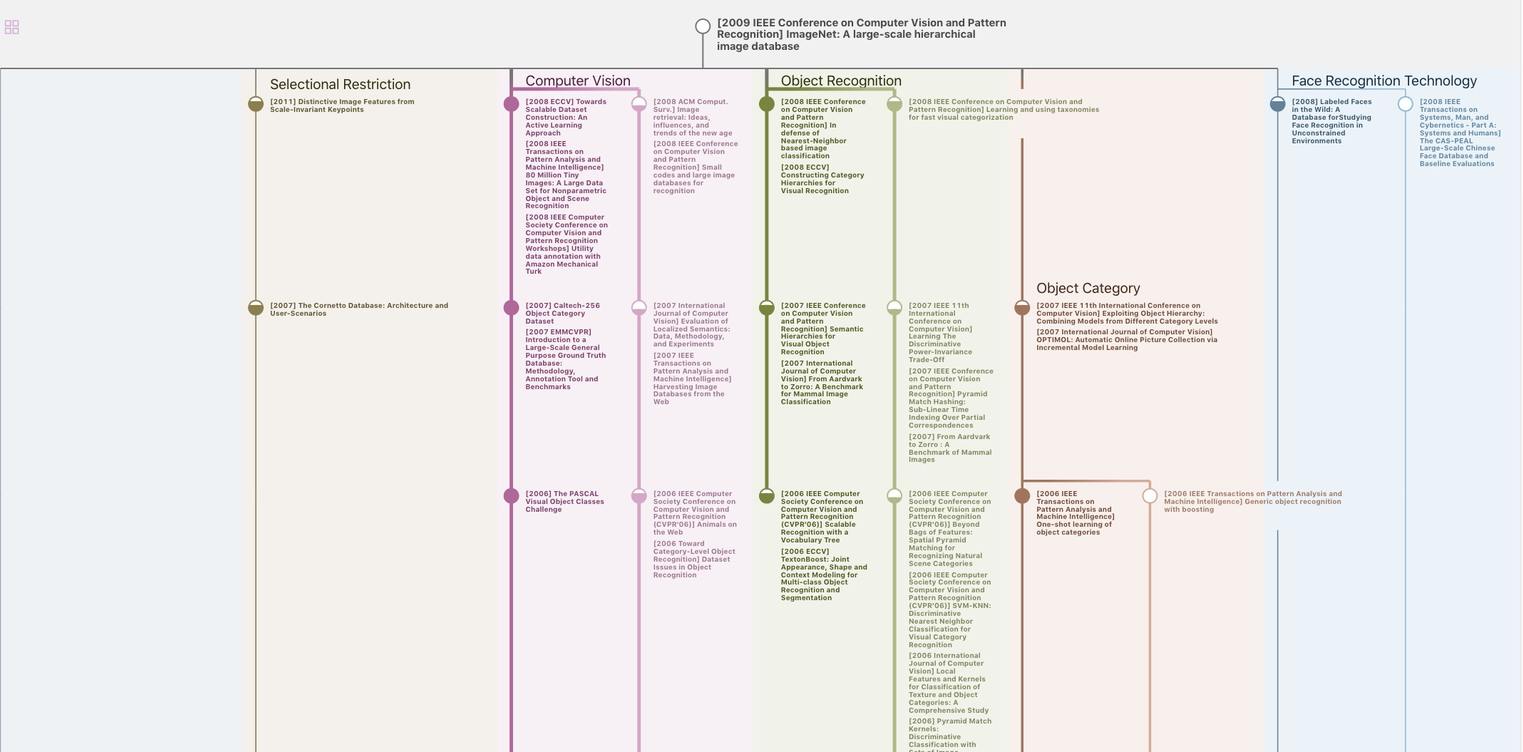
生成溯源树,研究论文发展脉络
Chat Paper
正在生成论文摘要