Artificial neural networks and adaptive neuro-fuzzy inference systems for prediction of soil respiration in forested areas southern Brazil
Environmental Monitoring and Assessment(2023)
摘要
The purpose of this study was to estimate the temporal variability of CO 2 emission (FCO 2 ) from O 2 influx into the soil (FO 2 ) in a reforested area with native vegetation in the Brazilian Cerrado, as well as to understand the dynamics of soil respiration in this ecosystem. The database is composed of soil respiration data, agroclimatic variables, improved vegetation index (EVI), and soil attributes used to train machine learning algorithms: artificial neural network (ANN) and an adaptive neuro-fuzzy inference system (ANFIS). The predictive performance was evaluated based on the mean absolute error (MEA), root mean square error (RMSE), mean absolute percentage error (MAPE), agreement index ( d ), confidence coefficient ( c ), and coefficient of determination ( R 2 ). The best estimation results for validation were FCO 2 with multilayer perceptron neural network (MLP) ( R 2 = 0.53, RMSE = 0.967 µmol m −2 s −1 ) and radial basis function neural network (RBF) ( R 2 = 0.54, RMSE = 0.884 µmol m −2 s −1 ) and FO 2 with MLP ( R 2 = 0.45, RMSE = 0.093 mg m −2 s −1 ) and RBF ( R 2 = 0.74, 0.079 mg m −2 s −1 ). Soil temperature and macroporosity are important predictors of FCO 2 and FO 2 . The best combination of variables for training the ANFIS was selected based on trial and error. The results were as follows: FCO 2 ( R 2 = 16) and FO 2 ( R 2 = 29). In all models, FCO 2 outperformed FO 2 . A primary factor analysis was performed, and FCO 2 and FO 2 correlated best with the weather and soil attributes, respectively.
更多查看译文
关键词
Soil CO 2 emission
AI 理解论文
溯源树
样例
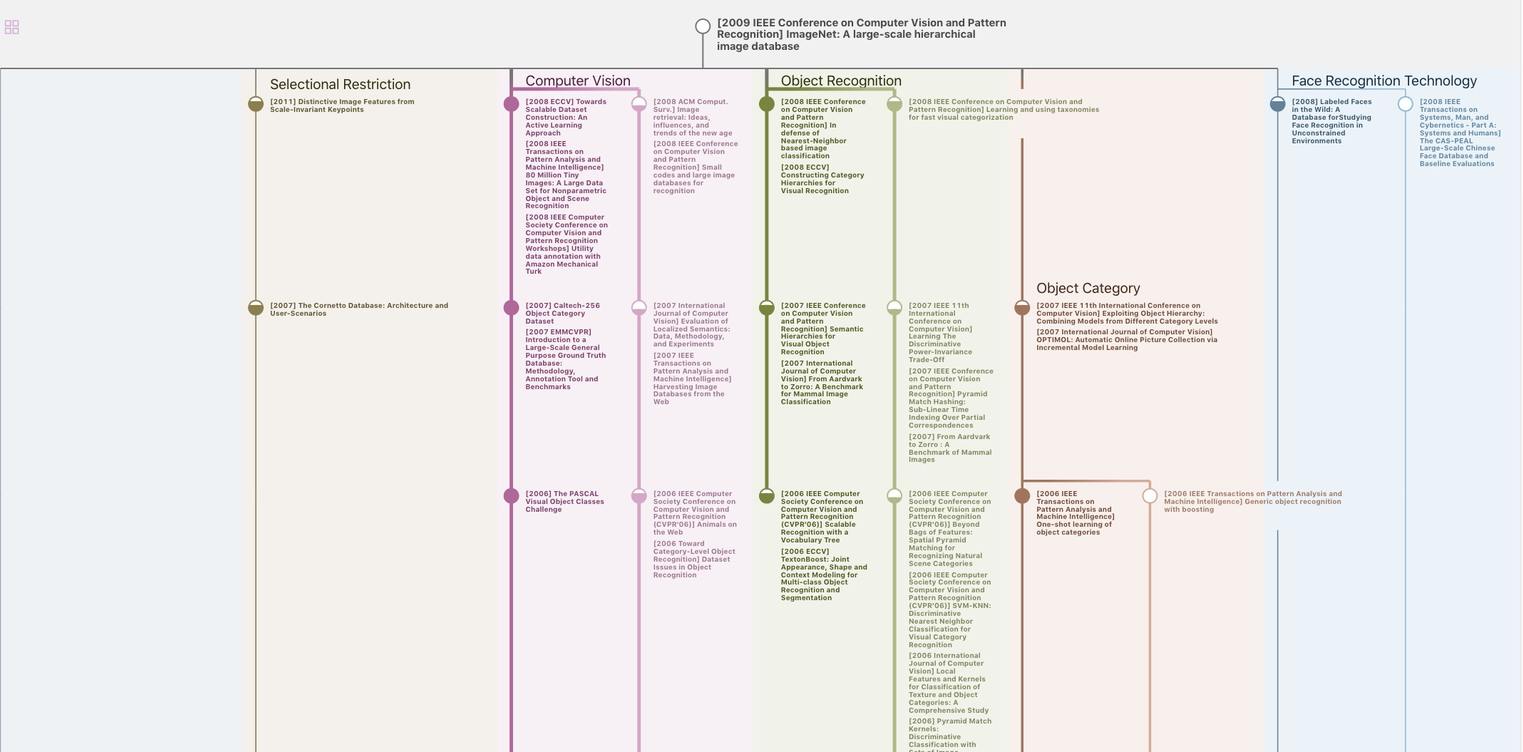
生成溯源树,研究论文发展脉络
Chat Paper
正在生成论文摘要