DreamDecompiler: Bayesian Program Learning by Decompiling Amortised Knowledge
arXiv (Cornell University)(2023)
摘要
Solving program induction problems requires searching through an enormous space of possibilities. DreamCoder is an inductive program synthesis system that, whilst solving problems, learns to simplify search in an iterative wake-sleep procedure. The cost of search is amortised by training a neural search policy, reducing search breadth and effectively "compiling" useful information to compose program solutions across tasks. Additionally, a library of program components is learnt to express discovered solutions in fewer components, reducing search depth. In DreamCoder, the neural search policy has only an indirect effect on the library learnt through the program solutions it helps discover. We present an approach for library learning that directly leverages the neural search policy, effectively "decompiling" its amortised knowledge to extract relevant program components. This provides stronger amortised inference: the amortised knowledge learnt to reduce search breadth is now also used to reduce search depth. We integrate our approach with DreamCoder and demonstrate faster domain proficiency with improved generalisation on a range of domains, particularly when fewer example solutions are available.
更多查看译文
关键词
bayesian program learning,dreamdecompiler,decompiling amortised
AI 理解论文
溯源树
样例
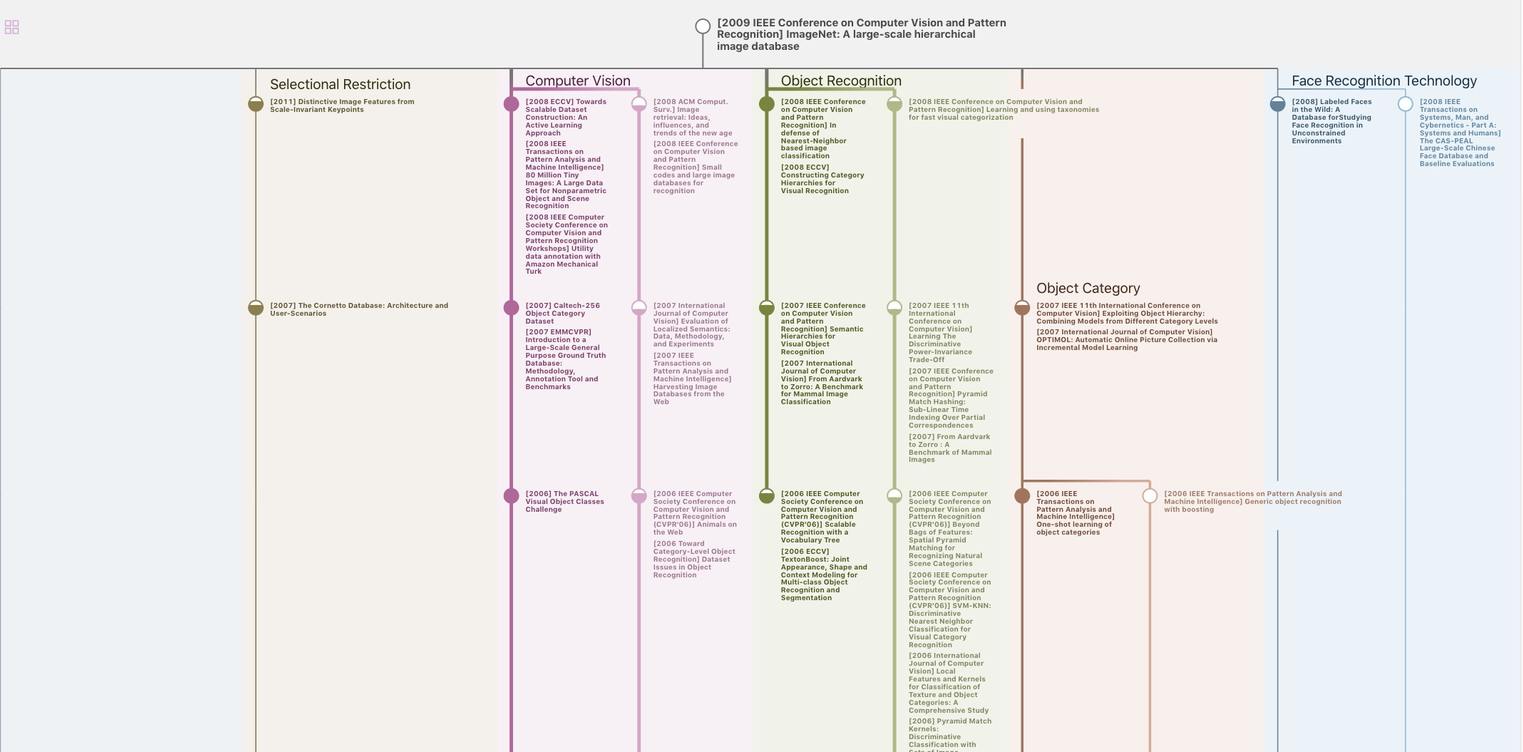
生成溯源树,研究论文发展脉络
Chat Paper
正在生成论文摘要