174-OR: Identification of Gestational Diabetes (GDM) Subtypes via Machine Learning and Associations with Perinatal Complications
Diabetes(2023)
摘要
There has been increasing recognition of GDM heterogeneity. We aimed to identify GDM subtypes using 45 variables from the electronic health records via Uniform Manifold Approximation and Projection and Density-Based Spatial Clustering of Applications with Noise, and assess associations with risk of perinatal complications via logistic regression. Since risk factors for GDM and complications were used to identify subtypes, association between subtypes and complications were not adjusted. In a cohort study of 37,561 racially and ethnically diverse individuals with GDM in 2011-2021, we split the sample into training/validation (70%/30%) sets. In the training set, 4 clusters (C) were identified. C1 (14.4%) and C2 (7.8%) were more likely to have early GDM diagnosed by Carpenter-Coustan criteria, pre-existing conditions (hypertension, prediabetes, polycystic ovary syndrome, and obesity), and to be older and multiparous, compared to C3 (65.7%) and C4 (12.1%; Table). Compared to C3 as the mildest and most common subtype, C2 and C4 had higher risk of preeclampsia/eclampsia, C2 had higher risk of placental abruption, and C1, C2, and C4 had higher risk of preterm birth, large for gestational age, and neonatal intensive care unit admission. Findings were confirmed in the validation set. Subtyping GDM may inform personalized risk assessment and management to improve perinatal outcomes. Disclosure Y.Zhu: None. R.Harvill: None. M.Greenberg: None. A.Ngo: None. L.D.Liao: None. R.F.Chehab: None. A.Ferrara: None. Funding National Institute of Diabetes and Digestive and Kidney Diseases (K01DK120807); National Heart, Lung, and Blood Institute (R01HL157666)
更多查看译文
关键词
gestational diabetes,machine learning,gdm,subtypes
AI 理解论文
溯源树
样例
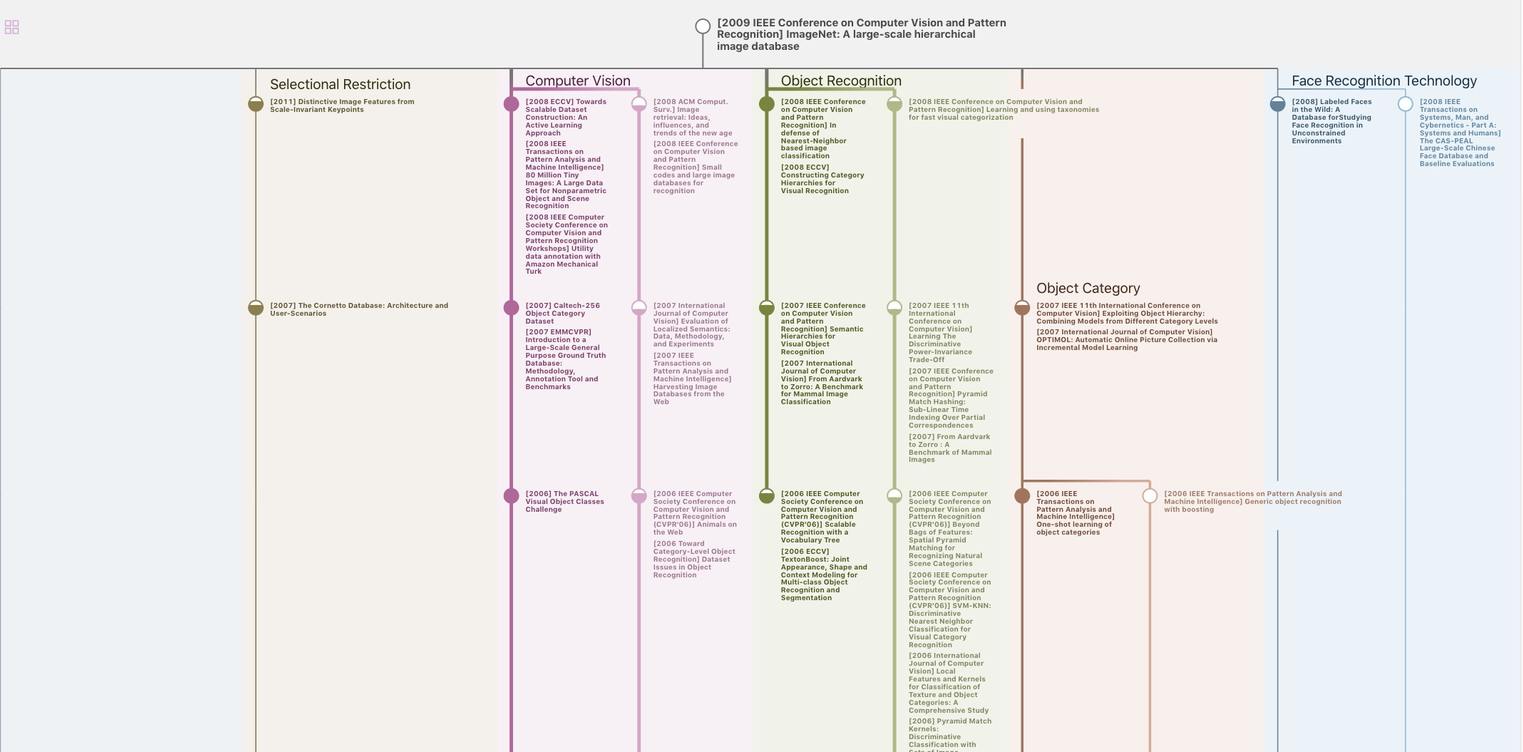
生成溯源树,研究论文发展脉络
Chat Paper
正在生成论文摘要