A Supervised Machine Learning Model to Predict Therapy Response and Mortality at 90 days After Acute Myeloid Leukemia Diagnosis
medRxiv (Cold Spring Harbor Laboratory)(2023)
摘要
Background and Objective The main objective in this paper is to validate a machine-learning model trained to predict the 90-day risk of complications for patients with Acute Myeloid Leukemia using variables available at diagnosis. This is a first fundamental step towards the development of a tool that could help physicians in their therapeutic decisions.
Methods 266 patients and 36 variables form the training dataset collected by Hospital 12 de Octubre (Madrid, Spain). The external test cohort provided by Instituto de Investigación Sanitaria La Fe (Valencia, Spain) contains 162 observations. An XGBoost model was trained with one dataset and validated with the other. Additionally, the features were ranked by permutation importance and compared with the ELN 2022 risk classification by genetics at initial diagnosis.
Results The model was evaluated with the training cohort using leave-one-out cross-validation, reaching a ROC-AUC of 0.85. By setting the functioning point that maximises Youden’s index, 3 out of 4 patients with complications and 84 out of 100 in remission are correctly classified. The model was validated with external data collected in a different hospital, achieving 0.7 ROC-AUC. At the best functioning point, almost 6 out of 10 patients with complications and 8 out of 10 patients in remission are correctly classified. Ranking the variables by descending importance, the top four are, in order: age, white-blood-cells count, Gender, and TP53. The list exhibits good coherence with the ELN 2022 risk classification.
Conclusions The model achieves performances that suggest it could be used as a therapeutical decision support tool. Important variables are coherent with ELN 2022 risk classification. Further work is needed to understand the reasons for the drop in test performance. The 90-day model should be supplemented by others that predict the risk of complications at six months or one year.
### Competing Interest Statement
The authors have declared no competing interest.
### Funding Statement
This work was partially supported by the Instituto de Salud Carlos III (ISCIII) through the projects PI19/00730, PI19/01518 and PI22/1088. It was co-funded by the European Union and by the Instituto de Investigación Hospital 12 de Octubre (imas12). This work was also funded by Generalitat Valenciana through IVACE (Valencian Institute of Business Competitiveness, https://www.ivace.es/index.php/es/) and the European Union through FEDER funding under project IMDEEA/2022/23.
### Author Declarations
I confirm all relevant ethical guidelines have been followed, and any necessary IRB and/or ethics committee approvals have been obtained.
Yes
The details of the IRB/oversight body that provided approval or exemption for the research described are given below:
The Institutional Ethics Committee for Clinical Research of Instituto de Investigación Sanitaria La Fe (IISLaFe, Valencia, Spain) approved this study with registration number 2019/0117. The Comité de Ética de la Investigación Clínica con Medicamentos (CEIm) of the Hospital 12 de Octubre (Madrid, Spain) approved this work with registration number 19/434.
I confirm that all necessary patient/participant consent has been obtained and the appropriate institutional forms have been archived, and that any patient/participant/sample identifiers included were not known to anyone (e.g., hospital staff, patients or participants themselves) outside the research group so cannot be used to identify individuals.
Yes
I understand that all clinical trials and any other prospective interventional studies must be registered with an ICMJE-approved registry, such as ClinicalTrials.gov. I confirm that any such study reported in the manuscript has been registered and the trial registration ID is provided (note: if posting a prospective study registered retrospectively, please provide a statement in the trial ID field explaining why the study was not registered in advance).
Yes
I have followed all appropriate research reporting guidelines, such as any relevant EQUATOR Network research reporting checklist(s) and other pertinent material, if applicable.
Yes
All data produced in the present study are available upon reasonable request to the authors.
更多查看译文
关键词
supervised machine learning model,acute myeloid,machine learning,leukemia,mortality
AI 理解论文
溯源树
样例
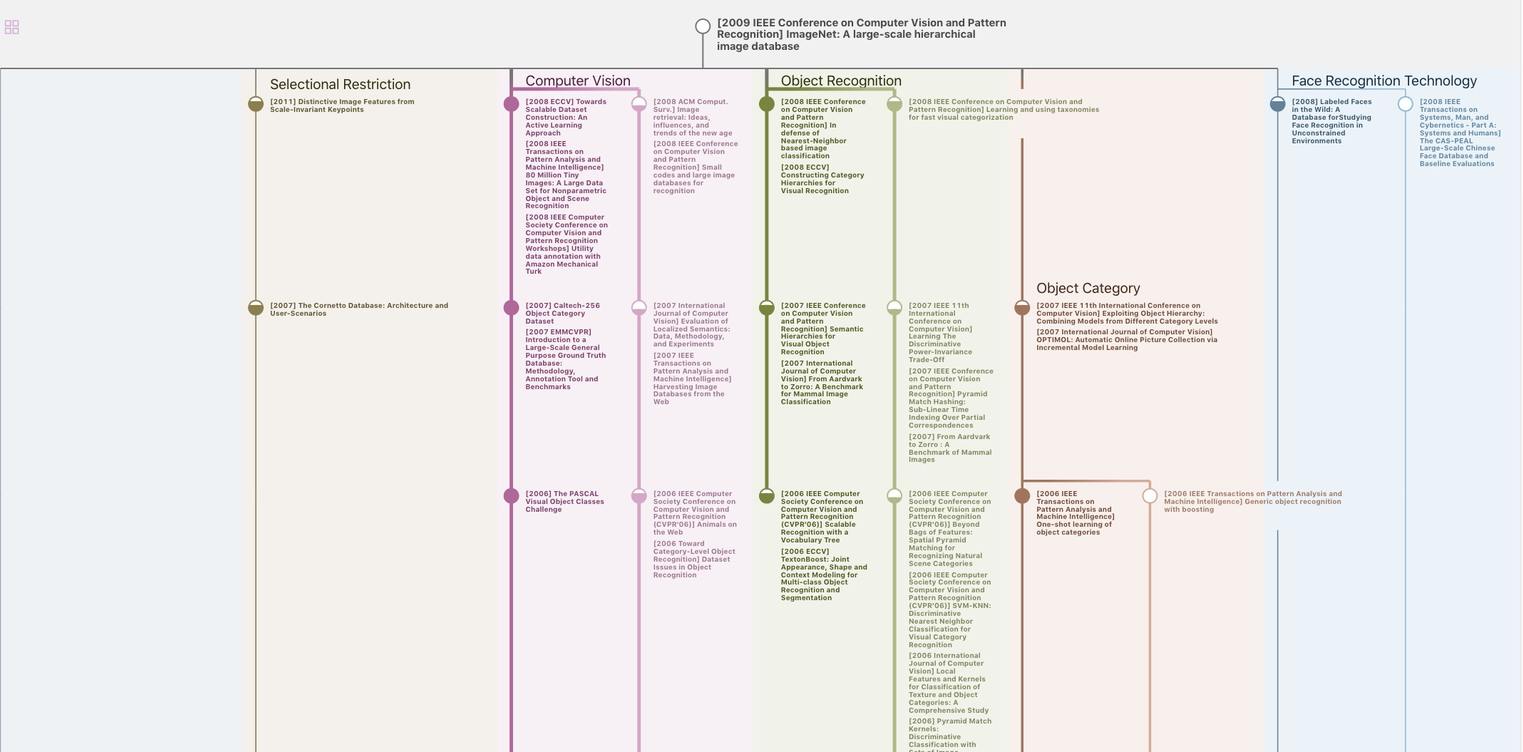
生成溯源树,研究论文发展脉络
Chat Paper
正在生成论文摘要