Development of a holistic Python package for optimal selection of experimental design criteria in kinetic model discrimination
Computer-aided chemical engineering(2023)
摘要
Starting with a candidate set of kinetic models for a reaction, model-based design of experiment (MBDoE) techniques can be used to determine experimental conditions for fast model identification of reaction kinetics using the minimum number of experimental runs to specify both model structure and corresponding parameters. However, practically, determining optimal settings, including criteria and selection methods for model discrimination for efficient model identification under consideration of parametric uncertainty in the whole identification procedure is still an open and challenging task. In this work, a holistic Python package is presented which comprises MBDoE for model discrimination and, subsequently, MBDoE for parameter precision. The new package is tested on in-silico experiments for the identification of a Baker’s Yeast model to evaluate and compare: i) the total number of experiments required for kinetic model identification considering different experimental design criteria; ii) the rate of correct model selections using different model selection methods.
更多查看译文
关键词
kinetic model discrimination,experimental design criteria,holistic python package,optimal selection
AI 理解论文
溯源树
样例
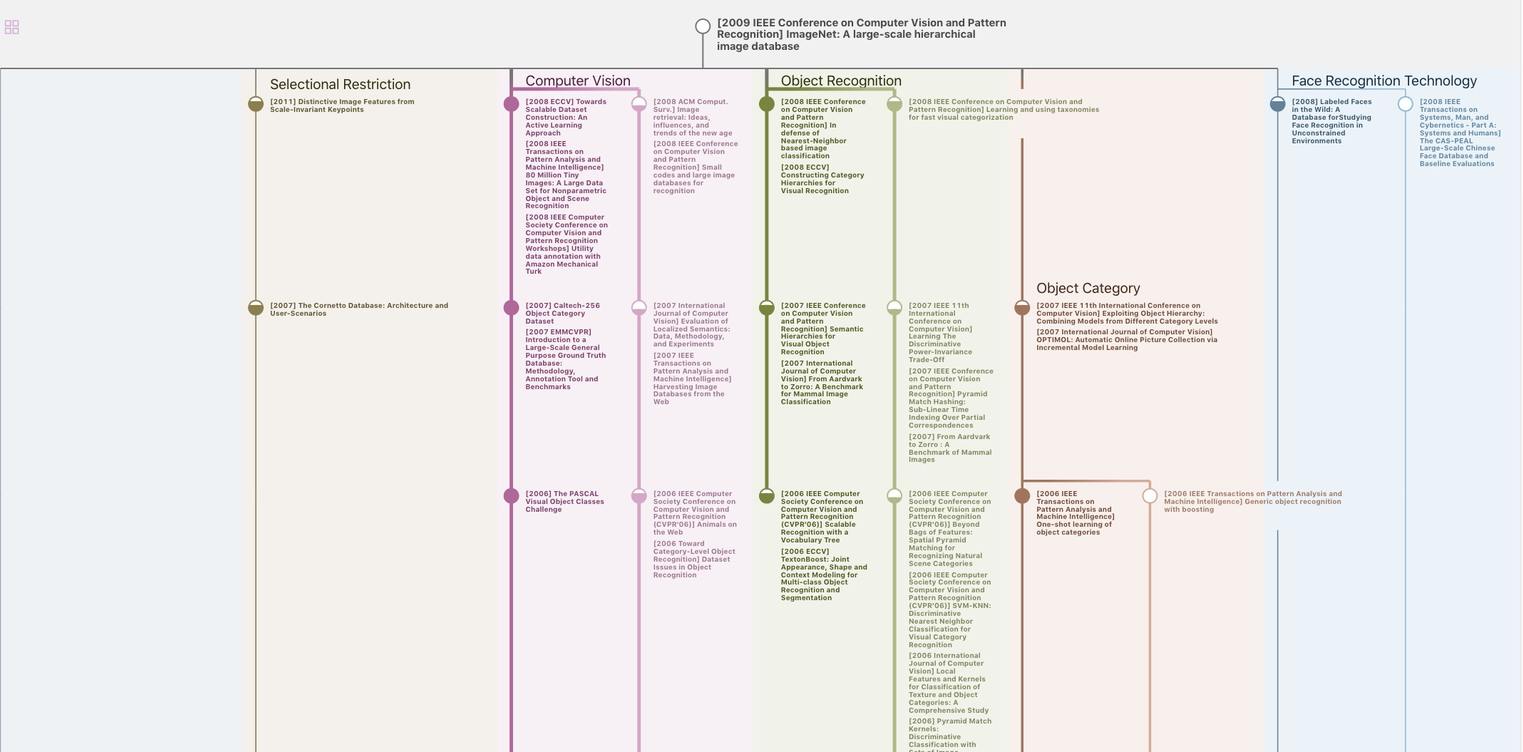
生成溯源树,研究论文发展脉络
Chat Paper
正在生成论文摘要