Modeling whole brain Electroencephalogram (EEG) in a spatially organized oscillatory neural network
bioRxiv (Cold Spring Harbor Laboratory)(2023)
摘要
ABSTRACT In this study, we model high-dimensional Electroencephalogram signals in sleep stages, using a general trainable network of Hopf oscillators. The proposed architecture has two components: a layer of oscillators with lateral connections, and a complex valued feedforward network with and without a hidden layer. The output of the Hopf oscillators, whose dynamics is described in the complex domain, is fed as input to the feedforward network and the output predicts the EEG signals. The proposed network is trained in two stages: in the 1st stage, intrinsic frequencies of the oscillators and the lateral connections are trained whereas, in the 2nd stage, the complex-valued feed-forward network is trained. Reconstruction error obtained when there is a hidden layer in the feedforward network is an order of magnitude smaller than that obtained without a hidden layer. Also, it has been shown that during testing our model is able to generate EEG signals, whose spectral properties accurately describe the corresponding target signals. In the first, the oscillators do not have any spatial localization, whereas in the second the oscillators are spatially distributed in a spherical shell geometry. The model described can be interpreted as a stepping stone towards a large-scale model of brain dynamics.
更多查看译文
关键词
whole brain electroencephalogram,eeg,neural network
AI 理解论文
溯源树
样例
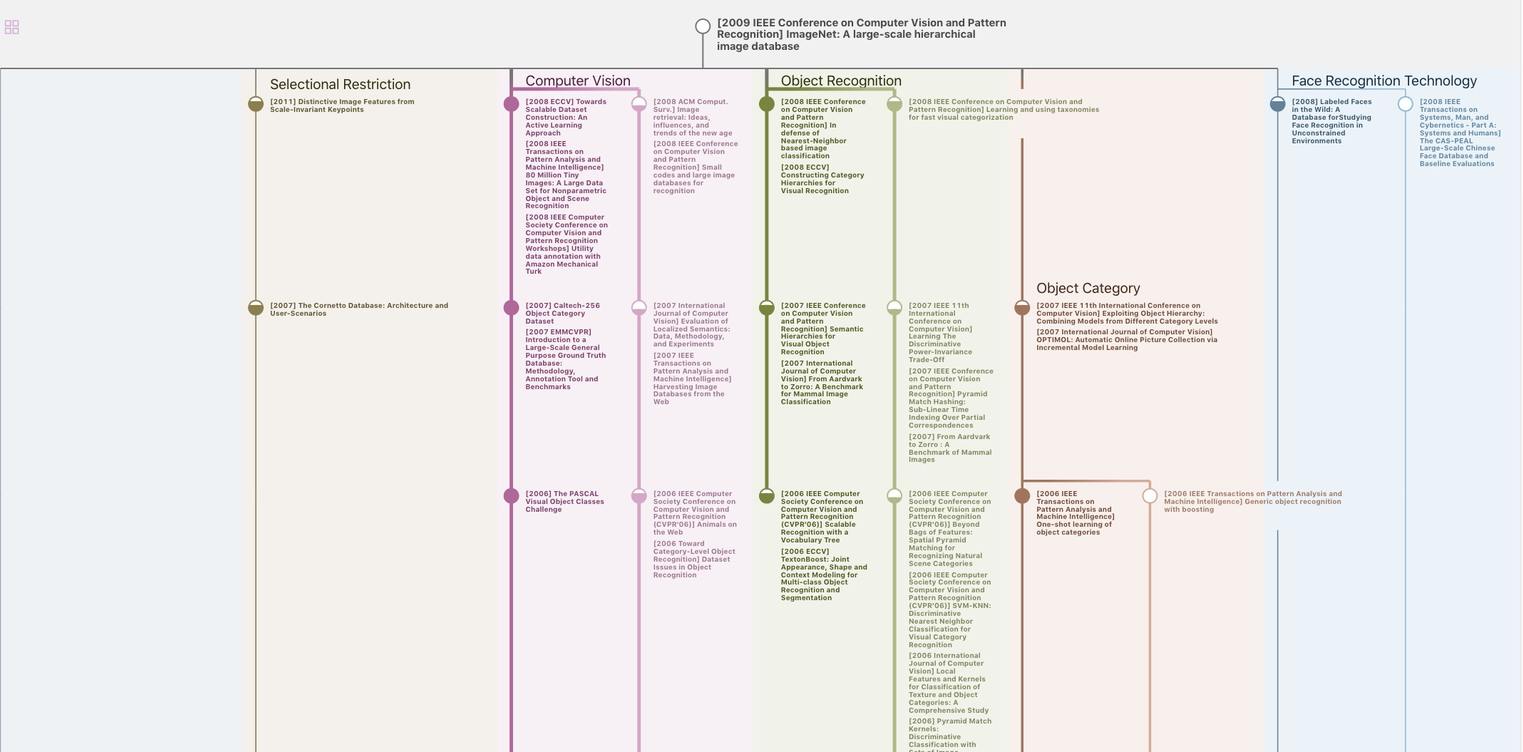
生成溯源树,研究论文发展脉络
Chat Paper
正在生成论文摘要