Biological Instance Segmentation with a Superpixel-Guided Graph
Proceedings of the Thirty-First International Joint Conference on Artificial Intelligence(2022)
摘要
Recent advanced proposal-free instance segmentation methods have made significant progress in biological images. However, existing methods are vulnerable to local imaging artifacts and similar object appearances, resulting in over-merge and over-segmentation. To reduce these two kinds of errors, we propose a new biological instance segmentation framework based on a superpixel-guided graph, which consists of two stages, i.e., superpixel-guided graph construction and superpixel agglomeration. Specifically, the first stage generates enough superpixels as graph nodes to avoid over-merge, and extracts node and edge features to construct an initialized graph. The second stage agglomerates superpixels into instances based on the relationship of graph nodes predicted by a graph neural network (GNN). To solve over-segmentation and prevent introducing additional over-merge, we specially design two loss functions to supervise the GNN, i.e., a repulsion-attraction (RA) loss to better distinguish the relationship of nodes in the feature space, and a maximin agglomeration score (MAS) loss to pay more attention to crucial edge classification. Extensive experiments on three representative biological datasets demonstrate the superiority of our method over existing state-of-the-art methods. Code is available at https://github.com/liuxy1103/BISSG.
更多查看译文
关键词
segmentation,instance,superpixel-guided
AI 理解论文
溯源树
样例
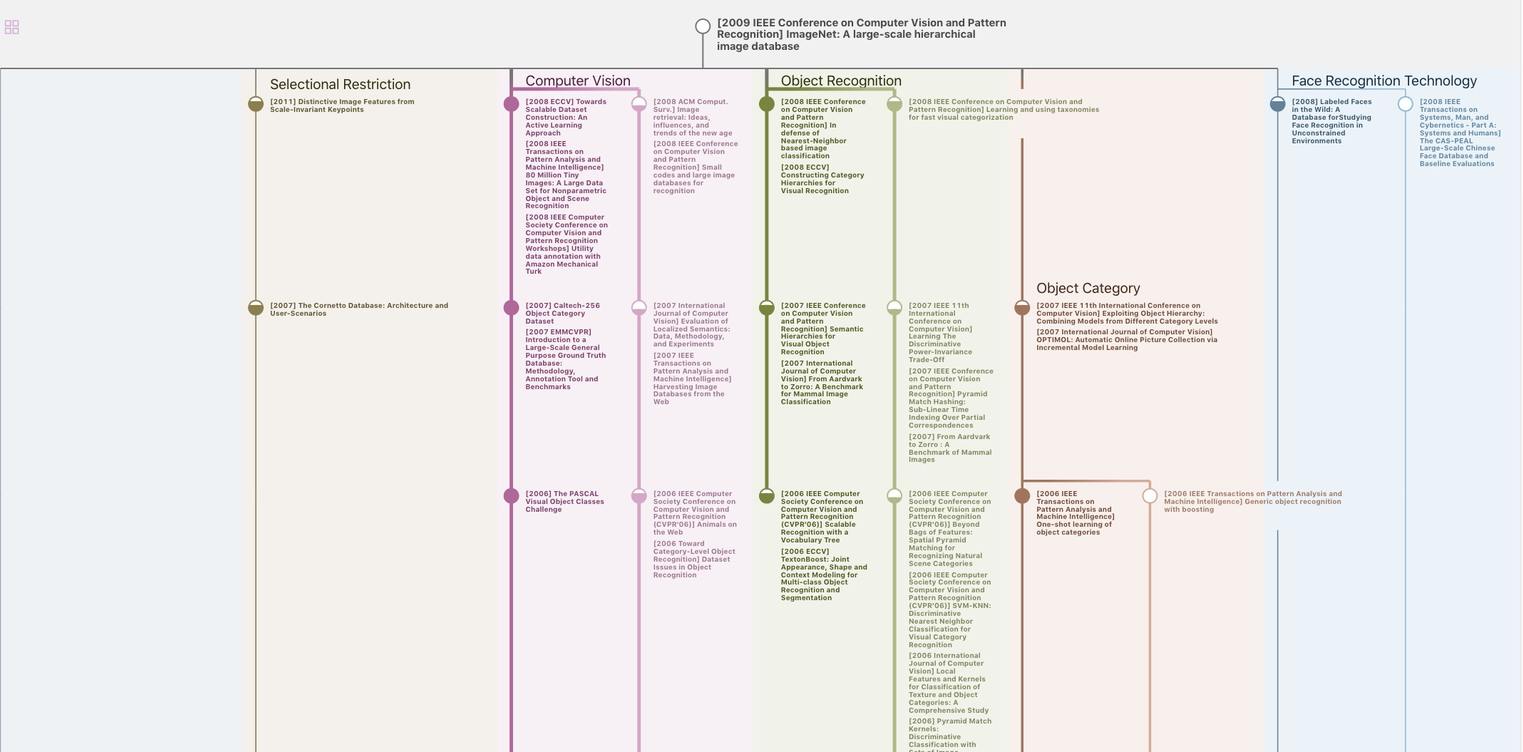
生成溯源树,研究论文发展脉络
Chat Paper
正在生成论文摘要