Beyond federated learning: On confidentiality-critical machine learning applications in industry
Procedia Computer Science(2021)
摘要
Federated machine learning frameworks, which take into account confidentiality of distributed data sources are of increasing interest in smart manufacturing. However, the scope of applicability of most such frameworks is restricted in industrial settings due to limitations in the assumptions on the data sources involved. In this work, first, we shed light on the nature of this arising gap between current federated learning and requirements in industrial settings. Our discussion aims at clarifying related notions in emerging sub-disciplines of machine learning, which are partially overlapping. Second, we envision a new confidentiality-preserving approach for smart manufacturing applications based on the more general setting of transfer learning, and envision its implementation in a module-based platform.
更多查看译文
关键词
machine learning,federated learning,collaborative learning,transfer learning,smart manufacturing
AI 理解论文
溯源树
样例
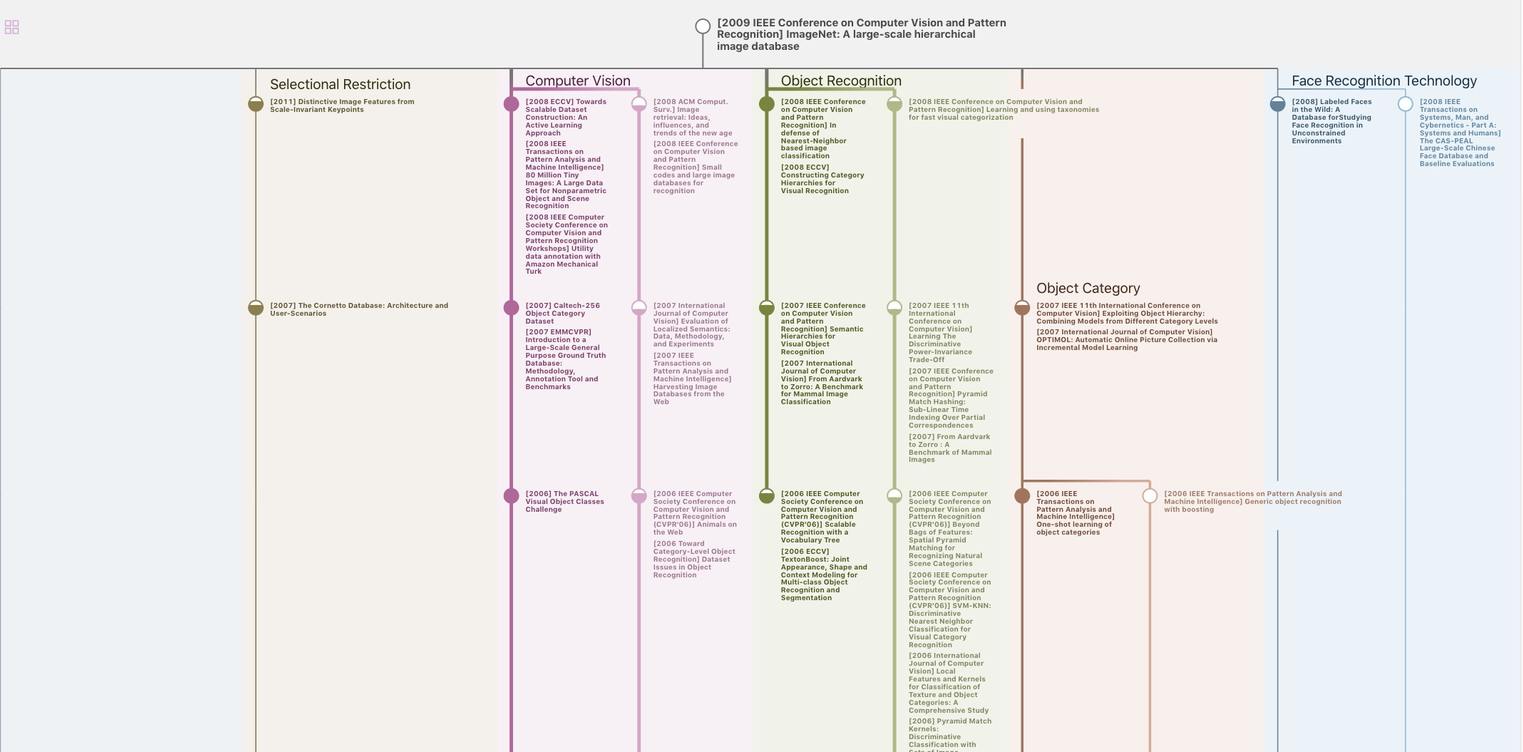
生成溯源树,研究论文发展脉络
Chat Paper
正在生成论文摘要